Documentation Revision Date: 2016-08-08
Data Set Version: V1
Summary
The global forest loss data set, a component of the Global Forest Change products (Hansen et al., 2013), provided a detailed assessment of annual forest loss across the full span of Russian forests between 2001 and 2012. Similar assessment from Landsat or other satellite imagery before 2001 was not possible due to large gaps in satellite image availability both in space and time. To obtain a wall-to-wall map of forest regrowth since the mid-1980s, a previously developed algorithm was used for reconstructing forest disturbances from MODIS data. Those estimates were combined with a Landsat-based assessment aggregated to the MODIS 500-m resolution to produce a nearly 30-year-long record of forest regeneration and a young forest distribution map.
There is one data file with this data set in GeoTIFF (.tif) format.
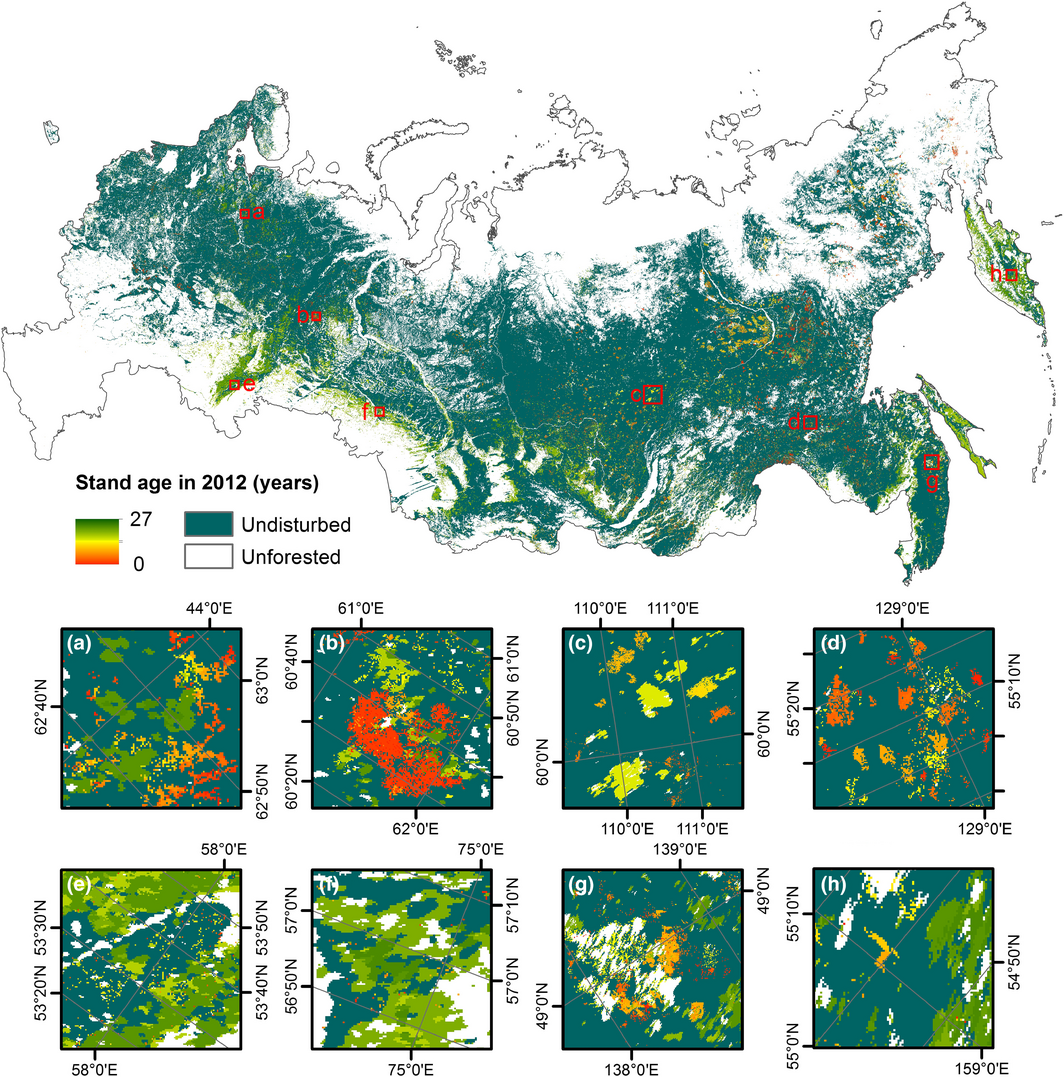
Figure 1. Distribution of young forests across Russia. Insets (a-h) show a closer view of stand age at eight selected sites. From Loboda and Chen (2016).
Citation
Loboda, T.V., and D. Chen. 2016. Distribution of Young Forests and Estimated Stand Age across Russia, 2012. ORNL DAAC, Oak Ridge, Tennessee, USA. http://dx.doi.org/10.3334/ORNLDAAC/1330
Table of Contents
- Data Set Overview
- Data Characteristics
- Application and Derivation
- Quality Assessment
- Data Acquisition, Materials, and Methods
- Data Access
- References
Data Set Overview
Project: Vegetation Collections
Russian forests span a large geographic domain and are governed by dramatically different climatic conditions. This results in a number of diverse forest types even though 95% of these forests belong within the boreal biome. In addition to the clear zonal distribution of forest types, the dominant species composition is also driven by the increased continentality of climate from west to east and further complicated by altitudinal gradients within the mountainous regions (Loboda and Chen, 2016). This data set provides estimated forest stand age and distribution of young (0-27 years of age) Russian forests, at the end of year 2012, across the full extent of Russia. The study utilized data from Hansen et al. (2013) and satellite data. The global forest loss data set, which is a component of the Global Forest Change products (Hansen et al., 2013), provided a detailed assessment of annual forest loss across the full span of Russian forests between 2001 and 2012. Similar assessment from Landsat or other satellite imagery before 2001 was not possible due to large gaps in satellite image availability both in space and time. To obtain a wall-to-wall map of forest regrowth since the mid-1980s, a previously developed algorithm was used for reconstructing forest disturbances from MODIS data. Those estimates were combined with a Landsat-based assessment aggregated to the MODIS 500-m resolution to produce a nearly 30-year-long record of forest regeneration and a young forest distribution map.
Related publication:
Loboda, T.V. and D. Chen, (2016). Spatial distribution of young forests and carbon fluxes within recent disturbances in Russia. Glob Change Biol. doi:10.1111/gcb.13349
Related data set:
Chen, D., T.V. Loboda, S. Channan, and A. Hoffman-Hall. 2015. Russian Boreal Forest Disturbance Maps Derived from Landsat Imagery, 1984-2000. ORNL DAAC, Oak Ridge, Tennessee, USA. http://dx.doi.org/10.3334/ORNLDAAC/1294
Acknowledgements:
This project was supported by the National Aeronautics and Space Administration's Carbon Cycle project NNX11AE39G and Land Cover and Land Use Change project NNX12AD34G.
Data Characteristics
Spatial Coverage: Russia
Spatial Resolution: 500 m
Temporal Coverage: Year 2012
Temporal Resolution: One time
Site boundaries: (All latitude and longitude given in decimal degrees)
Site (region) | Westernmost Longitude | Easternmost Longitude | Northernmost Latitude | Southernmost Latitude |
---|---|---|---|---|
Russia | -180 | 180 | 87.2401495 | 32.8630675 |
Data file information
There is one data file with this data set in GeoTIFF (.tif) format. File name: Russia_Stand_Age.tif
These data represent the spatial distribution of young forests (stand age < 27 years) in Russia at the end of 2012. Pixel values are stand age in years or the NoData value of 255.
Spatial Data Properties
Spatial Representation Type: Raster
Pixel Depth: 16 bit
Pixel Type: unsigned integer
Compression Type: LZW
Number of Bands: 1
Raster Format: TIFF
Source Type: generic
No Data Value: 255
Scale Factor: 1
Number Columns: 17,587
Column Resolution: 463.31 meter
Number Rows: 8,905
Row Resolution: 463.31 meter
Extent in the item's coordinate system
North: 3193236.4
South: -932539.15
West: -4325389.383
East: 3822843.587
Spatial Reference Properties
Type: Projected
Geographic Coordinate Reference: WGS 84
Projection: Albers Conical Equal Area
Open Geospatial Consortium (OGC) Well Known Text (WKT)
PROJCS["Albers_Conical_Equal_Area",
GEOGCS["WGS 84",
DATUM["WGS_1984",
SPHEROID["WGS 84",6378137,298.257223563,
AUTHORITY["EPSG","7030"]],
AUTHORITY["EPSG","6326"]],
PRIMEM["Greenwich",0],
UNIT["degree",0.0174532925199433],
AUTHORITY["EPSG","4326"]],
PROJECTION["Albers_Conic_Equal_Area"],
PARAMETER["standard_parallel_1",50],
PARAMETER["standard_parallel_2",70],
PARAMETER["latitude_of_center",56],
PARAMETER["longitude_of_center",100],
PARAMETER["false_easting",0],
PARAMETER["false_northing",0],
UNIT["metre",1,
AUTHORITY["EPSG","9001"]]]
Application and Derivation
These data could be used in studies that are closely related to stand age, including carbon and energy budgets (Loboda and Chen, 2016).
Quality Assessment
The data were evaluated through two accuracy assessments based on the administrative unit-aggregated forest inventory data for January 2003 from the Russian Forest Fund (Fomchenkov et al., 2003) and the Landsat-based spatially explicit estimates of forest conversion within the Eastern European Forest Cover Dynamics Project (Potapov et al., 2015).
In the statistical data from the Forest Fund, forest stand age is reported by four categories: young, transitional, nearing maturity, and mature spatially aggregated by administrative regions of Russia. Young forests, defined in the reported forestry statistics as forests of 30 years of age or younger, encompass the full range of stand ages within this study and were compared to the mapped regrowing forests as a whole. During the inventory assessment against the Forest Fund data, the stand age was assessed for young forest and total forested area within each of the 73 administrative regions encompassing seven Russian Federal Okrugs that cover the contiguous belt of Russian forests. To match the January 2003 assessment time stamp of the inventory data, ‘young forests’ in this assessment were defined as those with stand age between 10 and 27 years. The areas of these two classes within each administrative region were then cross-compared with the corresponding values documented in the Forest Fund data. Linear regression was fitted to assess the consistency between the two data sets.
The spatially explicit long-term Landsat-based assessment of forest dynamics over European Russia developed by Potapov et al. (2015) allowed for a spatially explicit accuracy assessment over a limited area of Russian forests. More importantly, it allowed for the assessment of MODIS-based estimates for 1985-2000 separately from the aggregated Landsat-based assessments between 2001 and 2010. The accuracy was assessed over the full extent of European Russia within the four different forest types subdivided by the bioclimatic zones. The overall accuracy for the distribution of forests exceeded 85% within three largest bioclimatic vegetation zones (northern, middle, and southern taiga), although accuracy for disturbed classes was generally low (the highest of 31% for user's and producer's accuracy for the 12–27 age class and the maximum of 74% for user's and 32% for producer's accuracy for the 0–11 age class) (Loboda and Chen, 2016).
Figure 2. Major geographic regions and distribution of forest cover by four bioclimatic zones across Russia. From Loboda and Chen (2016).
Data Acquisition, Materials, and Methods
Site description
Russian forests span a large geographic domain and are governed by dramatically different climatic conditions (Figure 2). This results in a number of diverse forest types even though 95% of these forests belong within the boreal biome. In addition to the clear zonal distribution of forest types, the dominant species composition is also driven by the increased continentality of climate from west to east and further complicated by altitudinal gradients within the mountainous regions. The boreal zone of European Russia is largely covered by dark coniferous and mixed (dark coniferous/broadleaved) forests with a distinct secondary succession by broadleaved deciduous species. The dark coniferous forest species include spruce (Picea abies and obovata), fir (Abies sibirica), and pine (Pinus sibirica) with birch (Betula spp.) and aspen (Populus spp.) in the understory and as secondary succession species. Further to the south through the middle taiga and into the southern taiga, proportional representation of pine and broadleaved species increases creating mixed coniferous/broadleaved forests with the gradual inclusion of poplar (Populus spp.), alder (Alnus spp.), and oak (Quercus spp.). Temperate forests of European Russia are typically oak, poplar, alder, linden (Tilia spp.), and hornbeam (Carpinus spp.) stands surrounded by agricultural development with birch frequently found in succession (Loboda and Chen, 2016).
This assessment does not include forests within the Southern Federal Okrug which are not part of the contiguous belt of boreal/temperate forests and are not included in this study (Loboda and Chen, 2016).
Methods
This study utilized data from Hansen et al. 2013, and satellite data. The global forest loss data set, which is a component of the Global Forest Change products (Hansen et al., 2013), provided a detailed assessment of annual forest loss across the full span of Russian forests between 2001 and 2012. Similar assessment from Landsat or other satellite imagery before 2001 was not possible due to large gaps in satellite image availability both in space and time. To obtain a wall-to-wall map of forest regrowth since the mid-1980s, a previously developed algorithm was used for reconstructing forest disturbances from MODIS data. Those estimates were combined with a Landsat-based assessment aggregated to the MODIS 500-m resolution to produce a nearly 30-year-long record of forest regeneration and a young forest distribution map.
Forest loss and regrowth were utilized only within areas which were defined in the Global Forest Change map (Hansen et al., 2013) as regions with tree cover of 10% or greater in year 2000 and any forest gained between 2001 and 2012 (Loboda and Chen, 2016).
Fig 3. Overall processing flow for estimating the stand age and distribution of young Russian forests (Loboda and Chen, 2016).
Forest stand age from MODIS data
A reconstructing algorithm was used based on random forest statistical projections of forest stand age using a set of MODIS metrics reflecting the spectral properties of forests of known age. Opportunistic samples of disturbances offered by the spatially and temporally discontinuous archive of Landsat Thematic Mapper (TM) and Enhanced Thematic Mapper Plus (ETM+) imagery across Russian forests between 1984 and 2000 were used to develop a set of known disturbances of an approximate age and detect undisturbed forests during the time of mapping forest stands across Russia (Chen et al., 2015). Fifty Landsat tiles where cloud-free images were available for five or more years between 1984 and 2000 were identified through random sampling within tree-dominated landscapes across Russia. Five additional image tile stacks were added to at the second round of selections to increase the volume of training data in underrepresented regions of Western and Eastern Siberia where image stack density was very low (2–3 images). Using the disturbance index (Healey et al., 2005) as the basis for the multitemporal change detection approach, stand-replacing disturbances and stable forest cover that could be identified with a high degree of certainty were mapped. Stand-replacing disturbances were subsequently tagged with the approximate year of disturbance based on the date of the imagery in which the area was identified as disturbed (Chen et al., 2015).
Fifty-four of the fifty-five Landsat tile-sample maps (excluding the tile sample from the temperate forests of Northern Caucasus) were aggregated to the 500-m resolution of MODIS surface reflectance imagery and used to yield three sets of training samples, each corresponding to one of the three subregions: European Russia (including western slopes of the Ural Mountains, 15 tile samples), Western Siberia (covering the Siberian lowlands between the Ural Mountains and the Yenisey River, seven tile samples), and Eastern Siberia (covering the upland regions east of the Yenisey River and mountainous areas in the south, 32 tile samples). These broad geographic regions represent general dominance of different forest types and various dominant regrowth patterns across Russian boreal forests. MODIS surface reflectance (MCD43A4) and thermal emissivity (MOD/MYD11A2) data from Terra and Aqua satellites for the year 2003 were used to develop 43 metrics characterizing forest state (Loboda et al., 2012). Capturing the relationship between time since disturbance and the 43 metrics at the MODIS resolution, the disturbance samples were fitted by three separate random forest classifiers (one for each subregion) to project stand age of young forests and identify undisturbed forests across each subregion as a whole. The three stand age maps were then merged into one, covering the Russian forest in its entirety (Loboda and Chen, 2016).
Merging the MODIS record with post-2000 era Landsat assessment of forest cover loss
In order to extend the record of stand age to the climatologically relevant time frame, Landsat-based 2001 - 2012 forest loss maps (Hansen et al., 2013) were aggregated to 500-m resolution using the MODIS overlay grid and the majority aggregation rule. The individual ‘year of forest loss’ layers (2001 - 2012) were collapsed into a single ‘latest year of forest loss’ layer prior to aggregation. Finally, the resultant map was converted into stand age with the 2012 disturbances corresponding to stand age of 0, and 2001 disturbances corresponding to stand age of 11. The aggregated disturbances were merged with the MODIS-based map.
To account for the overlap in mapping time frames between the MODIS-based (using 2003 data) and Landsat-based (2001 - 2012) data sets, all forests tagged with age category between 0 and 3 in the MODIS data-based product (equivalent to Landsat-based forest loss for the years 2001 - 2003) were removed from the map and replaced with the Landsat-based aggregated estimates. The resultant map presents a combination of mapped and modeled young forests likely disturbed between 1985 and 2012 as well as forests which have not been disturbed during this time frame and considered near mature and mature in this study. While it is likely that some areas were disturbed more than once during this period, the map reflects only regrowth since the most recent disturbances and thus reported statistics may underestimate the decadal rates of disturbance. The reported stand age within the MODIS-based record reflects a combination of various factors that influence forest regrowth after the disturbance. Those can include time since disturbance, burn severity, logging patterns, seed bank quality, climatic conditions following the disturbance that can enhance or impede seed establishment, and forest management decisions (e.g., planting of specific economically viable species or reseeding previously logged or burned regions) all of which may alter specific recovery trajectory and rate of various forest stands. In contrast, the reported stand age within the aggregated Landsat-based assessment reflects time since disturbance and has little association with regrowth rates (Loboda and Chen, 2016).
Data Access
These data are available through the Oak Ridge National Laboratory (ORNL) Distributed Active Archive Center (DAAC).
Distribution of Young Forests and Estimated Stand Age across Russia, 2012
Contact for Data Center Access Information:
- E-mail: uso@daac.ornl.gov
- Telephone: +1 (865) 241-3952
References
Chen, D., T.V. Loboda, S. Channan, and A. Hoffman-Hall. 2015. Russian Boreal Forest Disturbance Maps Derived from Landsat Imagery, 1984-2000. ORNL DAAC, Oak Ridge, Tennessee, USA. http://dx.doi.org/10.3334/ORNLDAAC/1294
Fomchenkov V, Sdobnova V, Danilov N, Danilova S, Kurdina G, Beljakova T (2003). Forest Fund of Russia (Data of State Forest Account, State by 1 January 2003) [in Russian]. All-Russia Research Institute of Forestry and Mechanisation, Moscow, Russia.
Hansen M.C., Potapov P. V., Moore R., Hancher M., Turubanova S. A., Tyukavina A., Thau D., Stehman S.V., Goetz S.J., Loveland T.R., Kommareddy A., Egorov A., Chini L., Justice C.O., Townshend J.R.G. (2013). High-resolution global maps of 21-st-century forest cover change. Science, 342, 850-853.
Healey SP, Cohen WB, Zhiqiang Y, Krankina ON (2005). Comparison of Tasseled Cap-based Landsat data structures for use in forest disturbance detection. Remote Sensing of Environment, 97, 301–310.
Loboda, T.V. and D. Chen, (2016). Spatial distribution of young forests and carbon fluxes within recent disturbances in Russia. Glob Change Biol. doi:10.1111/gcb.13349
Loboda TV, Zhang Z, O'neal KJ, Sun G, Csiszar IA, Shugart HH, and Sherman NJ.,. (2012). Reconstructing disturbance history using satellite-based assessment of the distribution of land cover in the Russian Far East. Remote Sensing of Environment, 118, 241–248.
Potapov PV, Turubanova SA, Tyukavina A, Krylov AM, Mccarty JL, Radeloff VC, Hansen M.C., (2015). Eastern Europe's forest cover dynamics from 1985 to 2012 quantified from the full Landsat archive. Remote Sensing of Environment, 159, 28–43.