Documentation Revision Date: 2015-11-12
Summary
A time-stamped single-layer disturbance map was produced for each of the 55 Landsat stacks. Across the full extent of Russian forests, 15 classes were mapped: 14 disturbed classes represented by the individual years during which the disturbances were observed, and one undisturbed class. Not all 14 classes were mapped in each stack and the number of classes was determined by stack density.
These maps provide crucial information regarding disturbance history for the selected regions across the Russian boreal forest and are designed to serve as a training and/or validation data set for coarse resolution data products. The overall disturbance classification accuracy was assessed to be good. This data set will benefit subsequent studies on a variety of aspects of the Russian boreal forest, especially in relation to the carbon budget and climate.
There are 55 forest disturbance maps provided in GeoTIFF (.tif) format with this data set.
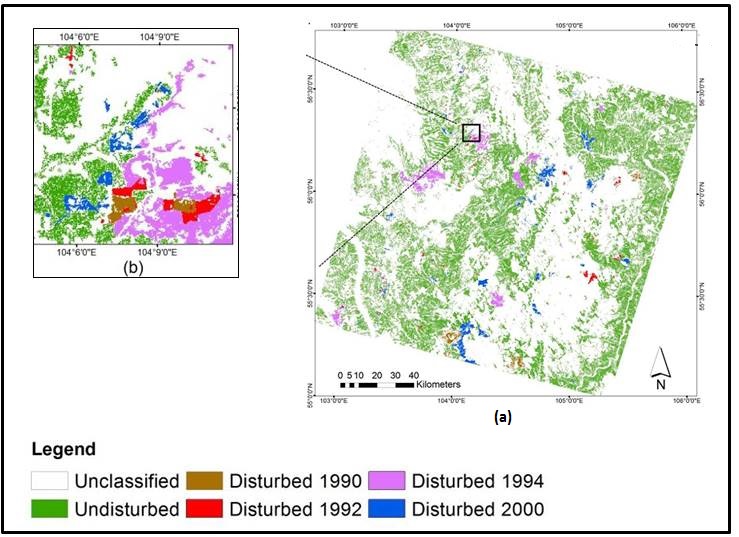
Disturbance map for a boreal forest site (Landsat Path 135, Row 21). Inset shows fine resolution of classifications. After Chen et al., 2014.
Citation
Chen, D., T.V. Loboda, S. Channan, and A. Hoffman-Hall. 2015. Russian Boreal Forest Disturbance Maps Derived from Landsat Imagery, 1984-2000. ORNL DAAC, Oak Ridge, Tennessee, USA. http://dx.doi.org/10.3334/ORNLDAAC/1294
Table of Contents
- Data Set Overview
- Data Characteristics
- Application and Derivation
- Quality Assessment
- Acquisition Materials and Methods
- Data Access
- References
Data Set Overview
This data set provides boreal forest disturbance maps at 30-m resolution across the Russian Federation for the years 1984 to 2000 derived from Landsat Thematic Mapper (TM) and Enhanced Thematic Mapper Plus (ETM+) imagery (stacks). MODIS Vegetation Continuous Fields (MOD44B) data for the year 2000 was used to delineate the forest areas to which subsequent processes were applied.
A time-stamped single-layer disturbance map was produced for each of the 55 Landsat stacks. Across the full extent of Russian boreal forests, 15 classes were mapped: 14 disturbed classes represented by the individual years during which the disturbances were observed, and one undisturbed class. Not all 14 classes were mapped in each stack and the number of classes was determined by stack density.
Data Characteristics
Spatial Coverage
The study area was 55 Landsat scenes located across the boreal forest in Northern Eurasia within the Russian Federation.
Spatial Resolution
30-m resolution for forest disturbance classes within each Landsat scene (approximately 170-km north-south by 185-km east-west)
Temporal Coverage
Data are for the periods 1984-2000
Temporal Resolution
Annual during the growing season, June-August
Study Area (All latitude and longitude given in decimal degrees)
Site | Westernmost Longitude | Easternmost Longitude | Northernmost Latitude | Southernmost Latitude |
---|---|---|---|---|
The boreal forest in Northern Eurasia within the Russian Federation. | 29.35547 | 139.0429 | 66.694742 | 41.75385 |
Data File Information
There are 55 GeoTIFF (.tif) files at 30-m resolution with this data set.
The files are named by the Landsat path and row number. For example, DSM_114021 where 114 is the path and 021 is the row. The DSM_ prefix denotes these are “disturbance sample maps”.
Mapped Disturbance Classes
Across the full extent of Russian boreal forests, 15 classes were mapped: 14 disturbed classes represented by the individual years during which the disturbances were observed, and one undisturbed class. Not all 14 classes were mapped in each stack and the number of classes was determined by stack density.
Table 1. Mapped forest disturbance classes.
Class Value | Class Meaning |
---|---|
0 | Unclassified |
1 | Undisturbed forest |
85 | Forest disturbed in 1985 |
86 | Forest disturbed in 1986 |
87 | Forest disturbed in 1987 |
88 | Forest disturbed in 1988 |
89 | Forest disturbed in 1989 |
90 | Forest disturbed in 1990 |
91 | Forest disturbed in 1991 |
92 | Forest disturbed in 1992 |
93 | Forest disturbed in 1993 |
94 | Forest disturbed in 1994 |
95 | Forest disturbed in 1995 |
96 | Forest disturbed in 1996 |
97 | Forest disturbed in 1997 |
98 | Forest disturbed in 1998 |
99 | Forest disturbed in 1999 |
100 | Forest disturbed in 2000 |
Spatial Data and Spatial Reference Properties
Table 2. GeoTIFF properties common to all data files.
GeoTIFF Property |
Common File Value |
file_type |
raster |
file_format |
GTiff |
crs_proj4 |
+proj=utm +zone=XX +datum=WGS84 +units=m +no_defs The content of the proj4 string varies only by UTM Zone (XX). The UTM Zone for each file is given in the next table. |
map_units |
meters |
res_x |
30 |
res_y |
30 |
n_bands |
1 |
data_type |
Int16 |
value_range_min |
0 |
Table 3. GeoTIFF properties unique to each data file.
Filename |
UTM Zone |
min_x |
min_y |
max_x |
max_y |
n_cols |
n_rows |
Value _range _max |
mapped classes |
DSM_114021.tif |
53 |
518775 |
6100665 |
721395 |
6297255 |
6754 |
6553 |
100 |
2000 |
DSM_114024.tif |
53 |
388785 |
5629575 |
586665 |
5829165 |
6596 |
6653 |
100 |
1999, 2000 |
DSM_115025.tif |
53 |
232755 |
5471205 |
452115 |
5686215 |
7312 |
7167 |
100 |
1992, 2000 |
DSM_117020.tif |
53 |
272175 |
6250215 |
487395 |
6457905 |
7174 |
6923 |
100 |
1999, 2000 |
DSM_117021.tif |
53 |
220095 |
6095175 |
452115 |
6314715 |
7734 |
7318 |
100 |
1999, 2000 |
DSM_117022.tif |
52 |
562155 |
5948655 |
779445 |
6153315 |
7243 |
6822 |
99 |
1994, 1999 |
DSM_117023.tif |
52 |
515685 |
5787945 |
733875 |
5995515 |
7273 |
6919 |
100 |
2000 |
DSM_121017.tif |
52 |
417825 |
6710535 |
637245 |
6919965 |
7314 |
6981 |
100 |
2000 |
DSM_121019.tif |
52 |
317895 |
6400035 |
536835 |
6612735 |
7298 |
7090 |
100 |
2000 |
DSM_121022.tif |
51 |
550185 |
5944515 |
754185 |
6143715 |
6800 |
6640 |
100 |
1990, 2000 |
DSM_122021.tif |
51 |
492075 |
6086385 |
718815 |
6298725 |
7558 |
7078 |
100 |
1992, 1999, 2000 |
DSM_123020.tif |
51 |
439695 |
6240285 |
665415 |
6457095 |
7524 |
7227 |
100 |
2000 |
DSM_124017.tif |
51 |
491775 |
6712665 |
705375 |
6922545 |
7120 |
6996 |
99 |
1999 |
DSM_124018.tif |
51 |
445425 |
6560685 |
668715 |
6777015 |
7443 |
7211 |
99 |
1999 |
DSM_124021.tif |
51 |
292695 |
6094365 |
523515 |
6312615 |
7694 |
7275 |
100 |
2000 |
DSM_127018.tif |
50 |
526425 |
6557805 |
736215 |
6765135 |
6993 |
6911 |
100 |
1998, 2000 |
DSM_127019.tif |
50 |
478425 |
6401235 |
701115 |
6619815 |
7423 |
7286 |
100 |
1998, 2000 |
DSM_130017.tif |
50 |
329355 |
6707805 |
540555 |
6921525 |
7040 |
7124 |
100 |
2000 |
DSM_131020.tif |
49 |
414315 |
6247845 |
628095 |
6457935 |
7126 |
7003 |
100 |
1994, 2000 |
DSM_133018.tif |
49 |
339525 |
6555465 |
554745 |
6770685 |
7174 |
7174 |
95 |
1995 |
DSM_133019.tif |
49 |
283395 |
6394185 |
510015 |
6624315 |
7554 |
7671 |
100 |
1995, 2000 |
DSM_135017.tif |
48 |
546135 |
6707685 |
772815 |
6925395 |
7556 |
7257 |
100 |
2000 |
DSM_135018.tif |
48 |
502395 |
6551985 |
726315 |
6777615 |
7464 |
7521 |
99 |
1999 |
DSM_135019.tif |
48 |
455205 |
6407265 |
664305 |
6607785 |
6970 |
6684 |
100 |
1994, 2000 |
DSM_135020.tif |
48 |
402615 |
6252015 |
631815 |
6465315 |
7640 |
7110 |
94 |
1990, 1992, 1994 |
DSM_135021.tif |
48 |
360765 |
6095025 |
583845 |
6309315 |
7436 |
7143 |
100 |
1990, 1992, 1994, 2000 |
DSM_135022.tif |
48 |
315015 |
5936355 |
522705 |
6146355 |
6923 |
7000 |
100 |
1990, 2000 |
DSM_137019.tif |
48 |
278235 |
6393585 |
501015 |
6616395 |
7426 |
7427 |
100 |
1991, 1995, 1999, 2000 |
DSM_137020.tif |
48 |
222105 |
6242085 |
447315 |
6468615 |
7507 |
7551 |
100 |
1991, 1995, 1998, 1999, 2000 |
DSM_138021.tif |
47 |
448185 |
6092235 |
666915 |
6308715 |
7291 |
7216 |
100 |
1991, 1992, 1994, 1999, 2000 |
DSM_140019.tif |
47 |
353775 |
6402105 |
564195 |
6613785 |
7014 |
7056 |
100 |
1990, 2000 |
DSM_143017.tif |
46 |
529635 |
6713775 |
737505 |
6924105 |
6929 |
7011 |
100 |
1992, 2000 |
DSM_147017.tif |
45 |
519735 |
6712275 |
731565 |
6924105 |
7061 |
7061 |
100 |
2000 |
DSM_148019.tif |
45 |
334875 |
6401595 |
548595 |
6615285 |
7124 |
7123 |
99 |
1990, 1991, 1999 |
DSM_151015.tif |
45 |
310545 |
7015785 |
537315 |
7234875 |
7559 |
7303 |
99 |
1999 |
DSM_152016.tif |
44 |
478215 |
6866535 |
691125 |
7078215 |
7097 |
7056 |
100 |
2000 |
DSM_161017.tif |
42 |
323505 |
6708975 |
542745 |
6925695 |
7308 |
7224 |
93 |
1987, 1988, 1993 |
DSM_164017.tif |
41 |
400635 |
6709365 |
612705 |
6923805 |
7069 |
7148 |
100 |
1987, 1989, 1999, 2000 |
DSM_165018.tif |
41 |
262635 |
6558915 |
472815 |
6772905 |
7006 |
7133 |
99 |
1988, 1992, 1993, 1999 |
DSM_165019.tif |
40 |
553815 |
6406605 |
769245 |
6618615 |
7181 |
7067 |
93 |
1986, 1988, 1993 |
DSM_165020.tif |
40 |
507195 |
6251505 |
726915 |
6462615 |
7324 |
7037 |
100 |
1986, 1987, 1988, 1990, 1992, 1993, 2000 |
DSM_167018.tif |
40 |
423045 |
6550785 |
644415 |
6769035 |
7379 |
7275 |
93 |
1987, 1988, 1993 |
DSM_170015.tif |
40 |
336525 |
7015095 |
559935 |
7233405 |
7447 |
7277 |
100 |
2000 |
DSM_170017.tif |
39 |
555255 |
6719595 |
751965 |
6922365 |
6557 |
6759 |
88 |
1988 |
DSM_170020.tif |
39 |
406035 |
6247650 |
615525 |
6457740 |
6983 |
7003 |
99 |
1987, 1988, 1989, 1999 |
DSM_171016.tif |
39 |
512985 |
6870015 |
734115 |
7082715 |
7371 |
7090 |
100 |
1987, 1988, 1989, 1994, 2000 |
DSM_171018.tif |
39 |
408825 |
6556815 |
621225 |
6767985 |
7080 |
7039 |
100 |
1987, 1999, 2000 |
DSM_173029.tif |
37 |
556665 |
4845435 |
741465 |
5037825 |
6160 |
6413 |
100 |
1986, 1988, 1989, 1991, 1992, 1998, 1999, 2000 |
DSM_174017.tif |
38 |
543300 |
6717750 |
744060 |
6921090 |
6692 |
6778 |
89 |
1987, 1988, 1989 |
DSM_175015.tif |
39 |
259515 |
7019175 |
491115 |
7241715 |
7720 |
7418 |
100 |
1987, 1988, 1989, 2000 |
DSM_176019.tif |
38 |
261885 |
6401325 |
475635 |
6616305 |
7125 |
7166 |
89 |
1988, 1989 |
DSM_179015.tif |
38 |
246645 |
7017225 |
481515 |
7243815 |
7829 |
7553 |
100 |
1988, 2000 |
DSM_179016.tif |
37 |
501795 |
6869205 |
704295 |
7074045 |
6750 |
6828 |
99 |
1985, 1986, 1988, 1999 |
DSM_182020.tif |
36 |
375345 |
6246765 |
583185 |
6457485 |
6928 |
7024 |
95 |
1988, 1992, 1994, 1995 |
DSM_183016.tif |
36 |
487590 |
6863520 |
697560 |
7075590 |
6999 |
7069 |
89 |
1988, 1989 |
Application and Derivation
These samples provide crucial information regarding disturbance history in selected regions across the Russian boreal forest and are designed to serve as a training and/or validation data set for coarse resolution data products. It can provide insights regarding the timing and locations of fire and logging events that occurred from 1985 to 2000 within the 55 selected Landsat stacks in the Russian boreal forest. Even though it is not a wall-to-wall assessment, this effort represents the first known product with this spatial resolution and geographical span before the year 2000 and could be expanded further using additional stacks. However, the current selection of stacks was considered to be a good representation of the heterogeneity of the Russian boreal forest in terms of both forest composition and disturbance regime.
Although the timing of disturbances are approximated and linked to the dates of available imagery rather than to the actual timing of disturbance, the applications of this data set include:
- An opportunity to assess variation in temporal changes in disturbance rates at decadal scales across Russia as a whole, and at finer temporal scales in European Russia, where dense stacks of Landsat data are available.
- The quantification of long-term forest disturbance history within individual Landsat scenes. While we did not provide a differentiation between fire- and logging-driven disturbances, this data set represents the basis for attribution based on extent, shape and possibly additional spectral information in the data.
- The potential to serve as a data input to studies that require information regarding the disturbance history of the Russian boreal forest.
This data set will benefit subsequent studies on a variety of aspects of the Russian boreal forest, especially in relation to the carbon budget and climate.
Quality Assessment
The results of the disturbance classification algorithm were evaluated through an analyst-driven double-blind validation (Chen et al., 2014). The double-blind method separates the process of mapping and random point generation from analyst-driven assessment by involving a separate set of analysts who have no a-priori knowledge of disturbances in the area and no prior involvement in the processing stream. These analysts are requested to examine the stack of imagery and assign the year of disturbance or undisturbed category to a set of points with no attributive information. No prior information is given to the analyst regarding the number of pixels expected to belong to a particular disturbed or undisturbed category with the varying number of points among image stacks.
The time-stamped sample points were compared with the classification results through the construction of confusion matrices and corresponding statistics including the omission and commission errors, overall accuracy and Kappa coefficient. In addition to the global accuracy assessment (i.e., across the entire Russian boreal forest), the 55 maps were divided into several groups based on either geographical location (i.e., European Russia, Western Siberia and Eastern Siberia) or stack density (i.e., sparse or dense). For each of these groups, the evaluation statistics were also calculated and compared.
The overall accuracy, calculated based on the 55 maps across the entire Russian boreal forest, was 83.98%, along with a Kappa coefficient of 0.83. Overall commission and omission errors of the classification were low. The average omission error for all classes was 11.24%, with the lowest omission error for 1998 disturbances (0.00%). The omission error for the undisturbed class was relatively high at 55.04%. In terms of commission error, the average value was 16.28%, and the range across all classes was relatively narrower than that of the omission error, with the largest and smallest being 30.48% (for 1990 disturbances) and 1.00% (for 1995 disturbances), respectively.
The values of overall accuracy and Kappa coefficient were similar for all the maps across the entire Russian forests as well as across three major geographic regions and between dense and sparse stacks of imagery. The lowest Kappa (0.76) and overall accuracy (79.01%) were found in Western Siberia—a region dominated by wetlands. The highest Kappa (0.85) and overall accuracy (86.69%) were found in Eastern Siberia. A small difference in Kappa and overall accuracy was also registered between sparse and dense stacks of imagery. It appeared that the confusion between specific time-stamps of disturbances was slightly higher in dense stacks, whereas a smaller number of selections in sparse stacks resulted in a clearer identification of the time of disturbance.
The classifier performance was less desirable in the identification of the undisturbed class (Error of Omission: 55.04%). This may have multiple causes. First, because the number of validation points selected for each class was similar (approximately 100), and because the undisturbed class appeared in almost all classified images, the number of validation points selected from the undisturbed class in each image was small (two points). Such a small number of validation points for this particular class resulted in the high sensitivity of the undisturbed class to errors. Another potential reason involved the capability of the analysts to correctly identify disturbances. There was large inter-annual variation in the beginning and end dates for the growing season. It is possible that the presence of phenological variations confused the analysts and caused them to identify forests as disturbed when this was not the case. Also, the analysts may have been less likely to differentiate between inter-annual and seasonal variability in forest conditions and natural or anthropogenic disturbances. As a result, the analysts might have mistakenly identified the undisturbed pixels as part of the disturbed classes, which inflated the Error of Omission for the undisturbed class. Also, forests affected by insect infestation may also have shown signs of depressed growth over a period of time, but never resulted in stand mortality, thus contributing to the misclassification by the analysts.
The accuracy of these disturbance maps was also examined according to stratification by geographic region and stack density. The results suggested that regardless of the grouping criteria (i.e., geographical location or stack density), the accuracy of the classification algorithm was generally consistent.
Acquisition Materials and Methods
Study area
The selected study area was the boreal forest in Northern Eurasia within the Russian Federation (European Russia, Western Siberia and Eastern Siberia).
- About 300 tree species are distributed across this vast area; the dominant species include Larix sibirica (Siberian or Russian Larch), L. gmelinii (Dahurian Larch), Pinus sylvestris (Scots Pine), P. sibirica (Siberian Pine), Picea abies (European or Norway Spruce), P. obovata (Siberian Spruce) and Betula spp. (Birch) (Tishkov 2002).
- The climate in the region is highly or extremely continental, characterized by very cold winters and warm summers (Shahgedanova 2002). Precipitation in the region is light or modest (Shahgedanova 2002).
- As with other boreal regions, wildfire is the most important disturbance agent in the Northern Eurasian boreal forest. An average area of 20,000–30,000 km2 is estimated as burned each year across the region (Goldammer and Furyaev 1996).
- In addition to wildfire, another major disturbance agent in the boreal forest of Northern Eurasia is logging.
Measurement Methods
Image Selection
This data set was derived from Landsat Thematic Mapper (TM) and Enhanced Thematic Mapper Plus (ETM+) imagery spanning the period between 1984 and 2000. MODIS Vegetation Continuous Fields (MOD44B) data for the year 2000 were used to delineate the forest areas to which subsequent processes were applied. The Vegetation Continuous Fields product was used to focus the distribution of the Landsat stacks within forested areas (defined here as all pixels with percent tree cover greater than 10%) and thus excluded large zones of croplands and grasslands in southern Russia and tundra in the north.
Preference was given to sites with Landsat images (stacks) containing the largest number of cloud-free images during the growing season (1 June through 31 August) and with near-annual observations rather than to those with the greatest number of images available since many images came from the growing season of the same year.
Selected Landsat images (stacks) for the 55 sites included 241 terrain-corrected L1T images acquired during the 1 June to 31 August growing seasons of 1984-2000. Sites were distributed across the full extent of Russian forests. European Russia, Western Siberia and Eastern Siberia had 16, 7, and 32 sites, respectively.
Figure 2. Distribution of the sites for which disturbance maps were generated. Red, blue and green represent sites in European Russia (16), Western Siberia (7), and Eastern Siberia (32), respectively. Letters in the polygons indicate the number of Landsat images in each time series stack, with ‘D’ and ‘S’ representing dense and sparse stacks. Note that the number of years (classes) of disturbed pixels was determined, in part, by the availability of Landsat images.
Image Pre-Processing and Masking
All L1T Landsat images were converted to surface reflectance using the Landsat Ecosystem Disturbance Adaptive Processing System (LEDAPS, V1.1.1) (Masek et al., 2006, Feng et al., 2013). The image stacks were clipped to their common extent to eliminate the variation in exact scene coverage between different images. Although preference was given to near-cloud-free imagery, considerable amounts of cloud and cloud shadow remained. To ensure high confidence disturbances mapping, liberal masks were designed to overestimate cloud and cloud shadow presence, particularly at the edges of clouds and cloud shadows. The masked-out pixels in the Landsat images were removed from the subsequent processes.
Disturbance Mapping
The method described in Healey, et al. (2005), which is based on the disturbance index (DI), was adopted as the core algorithm to detect disturbed pixels from the multiple Landsat images (stack) at a site. Multiple DI images from the growing season of the same year were composited to create a single annual DI image. DI image stacks were subsequently used to map and time-stamp forest disturbances. Difference DI (ΔDI) was calculated for each two adjacent years. Based on the ΔDI and additional criteria (Chen et al., 2014) a pixel was identified as a disturbed pixel and marked by the year in which it was discovered to be disturbed (i.e., the latter year in each image pair). If a pixel was disturbed multiple times as recorded by the temporal DI stack, the latest disturbance event in each stack was given precedence.
Following this protocol, a time-stamped single-layer disturbance map was produced for each site from the original Landsat images. Across the full extent of Russian forests, 15 classes were mapped: 14 disturbed classes represented by the individual years during which the disturbances were observed, and one undisturbed class. However, it is important to note that not all 14 disturbed classes were mapped for a given site and the number of classes was determined, in part, by the availability of Landsat images.
Data Access
This data is available through the Oak Ridge National Laboratory (ORNL) Distributed Active Archive Center (DAAC).
Russian Boreal Forest Disturbance Maps Derived from Landsat Imagery, 1984-2000
Contact for Data Center Access Information:
- E-mail: uso@daac.ornl.gov
- Telephone: +1 (865) 241-3952
References
Crist, E.P. A TM tasseled cap equivalent transformation for reflectance factor data. Remote Sens. Environ. 1985, 17, 301–306.
Crist, E.P. and R.C. Cicone. A physically-based transformation of thematic mapper data—The TM tasseled cap. IEEE Trans. Geosci. Remote Sens. 1984, 3, 256–263.
Feng, M., J.O. Sexton, C. Huang, J.G. Masek, E.F. Vermote, F. Gao, R. Narasimhan, S. Channan, R.E. Wolfe, and J.R. Townshend. Global surface reflectance products from Landsat: Assessment using coincident MODIS observations. Remote Sens. Environ. 2013, 134, 276–293.
Goldammer, J.G.; Furyaev, V.V. Fire in Ecosystems of Boreal Eurasia: Ecological Impacts and Links to the Global System. In Fire in Ecosystems of Boreal Eurasia; Goldammer, J.G., Furyaev, V.V., Eds.; Kluwer Academic Publishers: Dordrecht, the Netherlands, 1996; Volume 48, pp. 1–20.
Hansen, M.; DeFries, R.; Townshend, J.R.; Carroll, M.; Dimiceli, C.; Sohlberg, R. Percent Tree Cover, Collection 4, Vegetation Continuous Fields MOD44B. Available online: http://glcf.umd.edu/ data/vcf/ (accessed on 4 May 2014).
Healey, S.P.; Cohen, W.B.; Yang, Z.; Krankina, O.N. Comparison of tasseled cap-based Landsat data structures for use in forest disturbance detection. Remote Sens. Environ. 2005, 97, 301–310.
Jones, J.W.; Starbuck, M.J.; Jenkerson, C.B. Landsat Surface Reflectance Quality Assurance Extraction (Version 1.7); U.S. Geological Survey: Reston, VA, USA, 2013; Chapter C7, p. 9.
Kauth, R.J.; Thomas, G.S. The Tasseled Cap—A Graphic Description of the Spectral-Temporal Development of Agricultural Crops as Seen by Landsat. In Proceedings of the Symposium on Machine Processing of Remotely Sensed Data, West Lafayette, IN, USA, 29 June–1 July 1976; pp. 41–51.
Loboda, T.V.; Zhang, Z.; O’Neal, K.J.; Sun, G.; Csiszar, I.A.; Shugart, H.H.; Sherman, N.J. Reconstructing disturbance history using satellite-based assessment of the distribution of land cover in the Russian far east. Remote Sens. Environ. 2012, 118, 241–248.
Masek, J.G.; Vermote, E.F.; Saleous, N.E.; Wolfe, R.; Hall, F.G.; Huemmrich, K.F.; Feng, G.; Kutler, J.; Teng-Kui, L. A Landsat surface reflectance dataset for North America, 1990–2000. IEEE Geosci. Remote Sens. Lett. 2006, 3, 68–72.
Shahgedanova, M. Climate at Present and in the Historical Past. In The Physical Geography of Northern Eurasia; Shahgedanova, M., Ed.; Oxford University Press: New York, NY, USA, 2002; pp. 70–102.
Tishkov, A. Boreal Forests. In The Physical Geography of Northern Eurasia; Shahgedanova, M., Ed.; Oxford University Press: New York, NY, USA, 2002; pp. 216–233.