Documentation Revision Date: 2024-10-31
Dataset Version: 1
Summary
There are 204 data files in cloud optimized GeoTIFF format in this dataset.
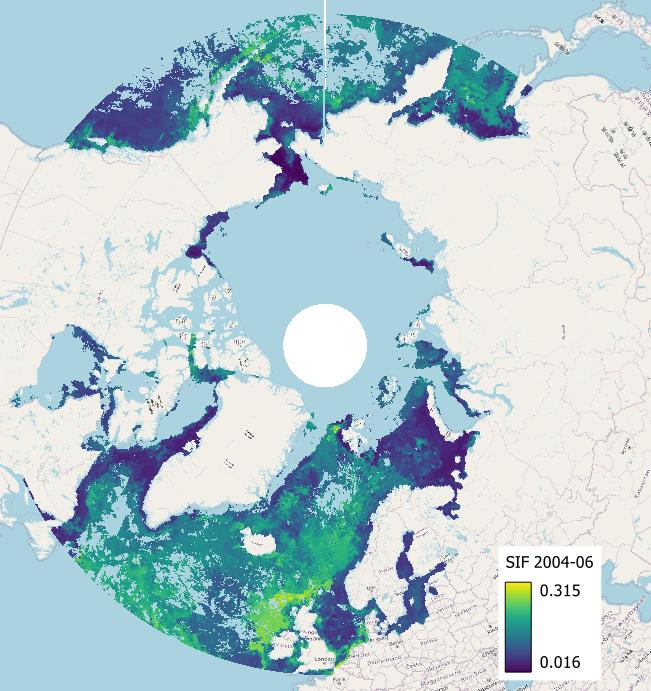
Figure 1. Monthly average solar-induced fluorescence (SIF) for Arctic Ocean for June 2004. Source: TROPOMI_ArcticOcean_SIF_2004_06.tif
Citation
Madani, N., N.C. Parazoo, M. Manizza, A. Chatterjee, D. Carroll, D. Menemenlis, V. Le Fouest, A. Matsuoka, K.M. Luis, C. Serra-Pompei, and C.E. Miller. 2024. Monthly SIF Estimates from TROPOMI over the Arctic Ocean, 2004-2020. ORNL DAAC, Oak Ridge, Tennessee, USA. https://doi.org/10.3334/ORNLDAAC/2378
Table of Contents
- Dataset Overview
- Data Characteristics
- Application and Derivation
- Quality Assessment
- Data Acquisition, Materials, and Methods
- Data Access
- References
Dataset Overview
This dataset provides solar-induced chlorophyll fluorescence (SIF) estimates over the Arctic Ocean at a 0.05-degree resolution for each month from January 2004 through December 2020. Red SIF data from TROPOMI (2018 to 2021) were extended over the study period using a random forest machine learning model trained using TROPOMI SIF climatological records. These data are useful for monitoring the physiological responses of phytoplankton to ongoing climate change over this ocean region.
Applications of this dataset are expected to provide new insights into how phytoplankton respond across time and space to environmental shifts and to contribute to a more nuanced understanding of their role in shaping high-latitude marine ecosystems.
Project: Solar Induced Fluorescence Earth Science Data Record
This project is developing a global, observation-based Earth System Data Record (ESDR) for quantifying global vegetation solar induced fluorescence (SIF) and photosynthesis gross primary productivity (GPP) from 1996-2020. It was funded under the 2017 Making Earth System Data Records for Use in Research Environments (MEaSUREs) call (17-MEASURES-0032).
Related Publication
Madani, N., N. C. Parazoo, M. Manizza, A. Chatterjee, D. Carroll, D. Menemenlis, V. Le Fouest, A. Matsuoka, K. Luis, C. Serra-Pompei, and C. E. Miller. 2024. A machine learning approach to produce a continuous solar-induced chlorophyll fluorescence dataset for understanding Ocean productivity. ESS Open Archive (2024-03-28). https://doi.org/10.22541/essoar.171164956.61516407/v1
Acknowledgments
This work received support from NASA's Interdisciplinary Research in Earth Science program.
Data Characteristics
Spatial Coverage: Arctic Ocean
Spatial Resolution: 0.05 degree
Temporal Coverage: 2004-01-01 to 2020-12-31
Temporal Resolution: Monthly
Study Area: Latitude and longitude are given in decimal degrees.
Site | Northernmost Latitude | Southernmost Latitude | Easternmost Longitude | Westernmost Longitude |
---|---|---|---|---|
Arctic Ocean | 90 | 50 | 180 | -180 |
Data File Information
There are 204 data files in cloud optimized GeoTIFF format in this dataset. Each file holds monthly averaged values of solar-induced fluorescence (SIF) over waters of the Arctic Ocean.
The files are named TROPOMI_ArcticOcean_SIF_<YYYY>_<MM>.tif (e.g., TROPOMI_ArcticOcean_SIF_2004_01.tif), where
- <YYYY> represents the year 2004 to 2020
- <MM> is the two-digit month
GeoTIFF characteristics
- Coordinate system: geographic cooridinates, WGS 1984 datum, EPSG 4326
- Spatial resolution: 0.05 degree
- Dimensions: 7200 columns x 673 rows
- Pixel values: Monthly average solar-induced fluorescence (SIF) in mW m-2 sr-1 nm-1
- Number of bands: one
- Nodata value: -9999
Application and Derivation
SIF as a proxy for primary productivity has dramatically increased our understanding of the response of global ecosystems to climate and disturbance events. Madani et al. (2024) highlights the significant potential of red SIF in monitoring marine photosynthesis responses across diverse ocean regions, particularly concerning seasonal and interannual variations in ocean biogeography. Moreover, these findings can offer deeper insights for quantifying and diagnosing sources of spatial and temporal variability in oceanic net primary production (NPP).
Given this first assessment, uncertainties persist regarding the assumptions about photosynthetic quantum yield in SIF, necessitating further research to accurately estimate NPP from SIF. Nevertheless, this study offers a new pathway to study ocean productivity, providing an independent approach that is complementary to chlorophyll-a based estimates, and which can be utilized in numerical, climate, and statistical models to assess ecosystem responses to recent changes in climate and physical ocean properties.
Quality Assessment
For quality assessment, machine learning models were developed using the random forests (RF) decision tree platform from a random sample of 70% of the TROPOMI SIF data and associated predictor variables. To prevent overfitting, the number of trees was constrained to 60. Estimates of MODIS chlorophyll-a (Chl_a) and normalized fluorescence line height (NFLH) were assessed as the main predictive components of the model. MODIS NFLH exhibited a higher proportion of variance in spatio-temporal correlations with the observed SIF across the study domain compared to Chl_a. Specifically, during the months of August and September, distinct differences between SIF and Chl_a were particularly noticeable along the coastal regions.
The model validation process included two steps. First, for each model, the significance of the model results was evaluated by comparing the fitted versus observed SIF values. Second, models were tested by fitting the trained models on the remaining 30% of the independent SIF and corresponding predictor variables. Additional analyses were performed at regional scales, where the model predicted SIF was compared with satellite observations of Chl_a and NFLH. In addition, In situ chlorophyll data obtained from the Tara Oceans Polar Circle cruises during 2013 was used for validation and comparison. The RF model prediction of SIF for the year 2013 was compared with direct in situ observations of Chl_a and corresponding direct observations of MODIS based Chl_a and NFLH.
Data Acquisition, Materials, and Methods
The TROPOMI sensor onboard the Sentinel-5 satellite provides wavelengths to capture SIF spectra for monitoring terrestrial, aquatic, and marine photosynthetic activity. Global retrievals of red wavelength SIF data have the advantage of retrieving photosynthetic information in variable atmospheric conditions with approximately 5-km spatial resolution (Köhler et al., 2018; Köhler et al., 2020). SIF signals are capable of penetrating through cloud and aerosol layers. Furthermore, unlike traditional usage of visible spectral bands, SIF is a photosynthesis by-product and is insensitive to ocean color. Despite the advantages of oceanic red SIF from TROPOMI, the recent availability of data (from April 2018 onward) makes quantifying long-term trends and anomalies in Arctic Ocean (AO) marine systems challenging.
This dataset provides spatial and temporally coherent red-SIF products beyond the original retrieved data that covers Apr 2018 to April 2021 (Köhler et al., 2020). Gridded TROPOMI red SIF climatological records were used to train monthly Random Forest (RF) models over selected predictive variables that are believed to impact photosynthetic activity (Table 1). Subsequently, SIF was predicted over the 2004-2020 period using temporal information provided by each of the explanatory variables.
Table 1. Candidate explanatory variables for predicting TROPOMI red-SIF for 2004-2020.
Variable | Abbreviation1 | Spatial resolution (degrees) | Source |
---|---|---|---|
Chlorophyll-a Concentration | Chl_a | Retrieved at 0.05 | NASA (2018a) |
Normalized Fluorescence Line Height | NFLH* | Retrieved at 0.05 | NASA (2018b) |
Sea-surface Temperature | SST* | Retrieved at 0.05 | Saha et al. (2018) |
Sea-surface Salinity | SSS* | 0.25 | Carroll et al. (2020) |
Meridional Wind Stress | vWind | 0.25 | Carroll et al. (2020) |
Zonal Wind Stress | uWind* | 0.25 | Carroll et al. (2020) |
Surface-ocean U Velocity | U | 0.25 | Carroll et al. (2020) |
Surface-ocean V Velocity | V* | 0.25 | Carroll et al. (2020) |
Distance from Coastal Zones | Distance* | 0.05 | - |
Aquatic Ecoregions | Ecoregions* | - | Spalding et al. (2007) |
*Variables used in the final model.
Explanatory variables were selected to represent spatial distribution of phytoplankton communities, as well as representing biotic, abiotic and physical characteristics of marine ecosystems across the AO domain. MODIS Chl_a and NFLH were obtained from Google Earth Engine (Gorelick et al., 2017), where monthly means were calculated based on 0.05-degree spatial resolution, consistent with TROPOMI SIF data. Marine biophysical data inputs were from the ECCO-Darwin ocean biogeochemistry state estimate (Carroll et al., 2020). Aquatic ecoregions (Spalding et al., 2007) were used as a proxy to represent seasonal nutrient cycle and availability across the AO ecosystems. Additionally, Euclidian distance from the coastal zones was used to represent nutrient transport along the land-ocean continuum. All analyses were performed in R (R Core Team, 2017) using open source libraries.
Data Access
These data are available through the Oak Ridge National Laboratory (ORNL) Distributed Active Archive Center (DAAC).
Monthly SIF Estimates from TROPOMI over the Arctic Ocean, 2004-2020
Contact for Data Center Access Information:
- E-mail: uso@daac.ornl.gov
- Telephone: +1 (865) 241-3952
References
Carroll, D., D. Menemenlis, J.F. Adkins, K.W. Bowman, H. Brix, S. Dutkiewicz, I. Fenty, M.M. Gierach, C. Hill, O. Jahn, P. Landschützer, J.M. Lauderdale, J. Liu, M. Manizza, J.D. Naviaux, C. Rödenbeck, D.S. Schimel, T. Van der Stocken, and H. Zhang. 2020. The ECCO-Darwin Data-Assimilative Global Ocean Biogeochemistry Model: estimates of seasonal to multidecadal surface ocean pCO2 and air-sea CO2 Flux. Journal of Advances in Modeling Earth Systems 12:e2019MS001888. https://doi.org/10.1029/2019MS001888
Gorelick, N., M. Hancher, M. Dixon, S. Ilyushchenko, D. Thau, and R. Moore. 2017. Google Earth Engine: Planetary-scale geospatial analysis for everyone. Remote Sensing of Environment 202:18–27. https://doi.org/10.1016/j.rse.2017.06.031
Köhler, P., C. Frankenberg, T.S. Magney, L. Guanter, J. Joiner, and J. Landgraf. 2018. Global Retrievals of Solar-Induced Chlorophyll Fluorescence With TROPOMI: First Results and Intersensor Comparison to OCO-2. Geophysical Research Letters 45:10456-10463. https://doi.org/10.1029/2018GL079031
Köhler, P., M.J. Behrenfeld, J. Landgraf, J. Joiner, T.S. Magney, and C. Frankenberg. 2020. Global Retrievals of Solar-Induced Chlorophyll Fluorescence at Red Wavelengths With TROPOMI. Geophysical Research Letters 47:e2020GL087541. https://doi.org/10.1029/2020GL087541
Madani, N., N. C. Parazoo, M. Manizza, A. Chatterjee, D. Carroll, D. Menemenlis, V. Le Fouest, A. Matsuoka, K. Luis, C. Serra-Pompei, and C. E. Miller. 2024. A machine learning approach to produce a continuous solar-induced chlorophyll fluorescence dataset for understanding Ocean productivity. ESS Open Archive (2024-03-28). https://doi.org/10.22541/essoar.171164956.61516407/v1
NASA. 2018a. Moderate-resolution Imaging Spectroradiometer (MODIS) Aqua Mapped Chlorophyll Data. NASA OB.DAAC, Greenbelt, MD, USA. https://doi.org/10.5067/AQUA/MODIS/L3M/CHL/2018
NASA. 2018b. Moderate-resolution Imaging Spectroradiometer (MODIS) Aqua Level-2 Ocean Color, version 2018. NASA OB.DAAC, Greenbelt, MD, USA. https://doi.org/10.5067/AQUA/MODIS/L2/OC/2018
R Core Team. 2017. R: a language and environment for statistical computing. R Foundation for Statistical Computing; Vienna, Austria. https://www.r-project.org/
Saha, K., X. Zhao, H.-M. Zhang, K.S. Casey, D. Zhang, S. Baker-Yeboah, K.A. Kilpatrick, R.H. Evans, T. Ryan, and J.M. Relph. 2016. AVHRR Pathfinder version 5.3 level 3 collated (L3C) global 4km sea surface temperature for 1981-Present. NOAA National Centers for Environmental Information. https://doi.org/10.7289/V52J68XX
Spalding, M.D., H.E. Fox, G.R. Allen, N. Davidson, Z.A. Ferdaña, M. Finlayson, B.S. Halpern, M.A. Jorge, A. Lombana, S.A. Lourie, K.D. Martin, E. McManus, J. Molnar, C.A. Recchia, and J. Robertson. 2007. Marine Ecoregions of the World: A Bioregionalization of Coastal and Shelf Areas. BioScience 57:573–583. https://doi.org/10.1641/B570707