Documentation Revision Date: 2018-09-18
Data Set Version: 1
Summary
The 473 soil samples in the study were part of a larger dataset of 650 soil samples collected by the United States Geological Survey (USGS) from the USGS Geochemical Landscapes Project (Smith et al., 2011). The concentration of SOC fractions in the 650 samples were predicted using mid-infrared (MIR) spectroscopy and partial least squares regression (PLSR) analysis. The spectra were then processed using Unscrambler 10.2 software. Predictions for SOC, PyC, and POC were made in Unscrambler using calibration models and fractionation analysis. The samples were statistically analyzed and validated using an independent 2014 soil dataset from the USGS to create the more robust dataset of 473 samples for further analysis and mapping in a random forest kriging regression model.
There are six data files with this dataset. This includes four files in GeoTIFF format (.tif), one file in comma-separated format (.csv), and one shapefile provided as a zip file.
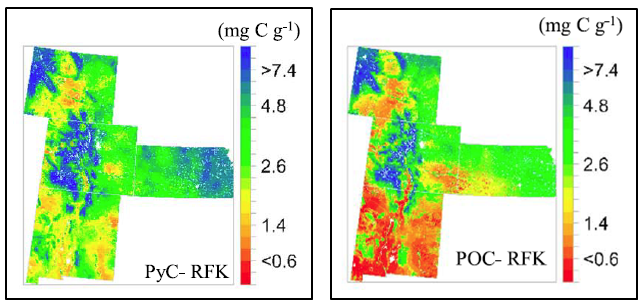
Figure 1. Maps of predicted pyrogenic carbon (PyC) and particulate organic carbon (POC) of Great Plains soils using random forest kriging (RFK) at 1 km resolution. The map files, pyrogenic_carbon_random_forest.tif and particulate_organic_carbon_random_forest.tif, are provided with this dataset.
Citation
Ahmed, Z.U., P.B. Woodbury, J. Sanderman, B. Hawke, V. Jauss, D. Solomon, and J. Lehmann. 2018. Stocks of Surface Soil Organic Carbon Fractions, Great Plains Region, USA, 2007-2010. ORNL DAAC, Oak Ridge, Tennessee, USA. https://doi.org/10.3334/ORNLDAAC/1603
Table of Contents
- Data Set Overview
- Data Characteristics
- Application and Derivation
- Quality Assessment
- Data Acquisition, Materials, and Methods
- Data Access
- References
Data Set Overview
This dataset provides estimates of total organic soil carbon (SOC), pyrogenic (PyC), particulate (POC), and other organic soil carbon (OOC) fractions in 473 surface layer soil samples collected from stratified-sampling locations in Colorado, Kansas, New Mexico, and Wyoming, USA. Terrain, climate, soil, fire, and land cover data used to predict and map SOC, PyC, POC, and OOC throughout the study region are also included. The estimates were derived using a best random forest kriging model and cover the period 2007-05-01 to 2010-10-01.
The 473 soil samples in the study were part of a larger dataset of 650 soil samples collected by the United States Geological Survey (USGS) as a part of the USGS Geochemical Landscapes Project (Smith et al., 2011). The concentration of SOC fractions in the 650 samples were predicted using mid-infrared (MIR) spectroscopy and partial least squares regression (PLSR) analysis. The spectra were then processed using Unscrambler 10.2 software. Predictions for SOC, PyC, and POC were made in Unscrambler using calibration models and fractionation analysis. The samples were statistically analyzed and validated using an independent 2014 soil dataset from the USGS to create the more robust dataset of 473 samples for further analysis in a random forest regression model.
Project: North American Carbon Program
The North American Carbon Program (NACP) is a multidisciplinary research program to obtain scientific understanding of North America's carbon sources and sinks and of changes in carbon stocks needed to meet societal concerns and to provide tools for decision makers. The NACP is supported by a number of different federal agencies. The central objective is to measure and understand the sources and sinks of Carbon Dioxide (CO2), Methane (CH4), and Carbon Monoxide (CO) in North America and in adjacent ocean regions.
Related Publication:
Ahmed, Z.U., P.B. Woodbury, J. Sanderman, B. Hawke, V. Jauss, D. Solomon, and J. Lehmann (2017), Assessing soil carbon vulnerability in the Western USA by geospatial modeling of pyrogenic and particulate carbon stocks, J. Geophys. Res. Biogeosci., 122, 354-369, https://doi.org/10.1002/2016JG003488
Acknowledgements:
This study was generated with support from the USDA (grant number 2008-35615-18961).
Data Characteristics
Spatial Coverage: Colorado, Kansas, New Mexico, and Wyoming, USA
Spatial Resolution: Soil samples were collected from multiple points
Temporal Resolution: The data cover the period 2007-05-01 to 2010-10-01
Study Area (coordinates in decimal degrees)
Site | Westernmost Longitude | Easternmost Longitude | Northernmost Latitude | Southernmost Latitude |
---|---|---|---|---|
Colorado, Kansas, New Mexico, and Wyoming, USA | -111.932 | -94.4406 | 45.83028 | 31.22028 |
Data File Information
There are six data files with this dataset. This includes four files in GeoTIFF format (.tif), one file in comma-separated format (.csv), and one shapefile provided as a zip file.
Table 1. Data files and descriptions
File names | Descriptions |
---|---|
soil_organic_c_great_plains.csv | Estimates of total organic soil (SOC), pyrogenic (PyC), particulate (POC), and other organic soil carbon (OOC) fractions in 473 soil samples from the A horizon in Colorado, Kansas, New Mexico, and Wyoming, USA. Terrain, climate, soil, fire, and land cover data used in the random forest model to predict and map SOC, PyC, POC, and OOC throughout the study region are also included. |
SOC_great_plains.zip | Soil organic carbon map provided as a shapefile (.shp) |
pyrogenic_carbon_random_forest.tif | Estimated pyrogenic carbon at 1 km2 resolution provided in GeoTIFF (.tif) format |
particulate_organic_carbon_random_forest.tif | Estimated particulate organic carbon at 1 km2 resolution provided in GeoTIFF (.tif) format |
other_SOC_random_forest.tif | Other estimated soil organic carbon fractions at 1 km2 resolution provided in GeoTIFF (.tif) format |
SOC_random_forest.tif | Soil organic carbon at 1 km2 resolution provided in GeoTIFF (.tif) format |
Variables in the data files
Table 2. Variables in the data files soil_organic_c_great_plains.csv and SOC_great_plains.zip
Variable name-.csv file | Variable name-.shp file | Units/format | Description |
---|---|---|---|
id | ID | sample ID | |
longitude | Not included | decimal degrees | Longitude |
latitude | Not included | decimal degrees | Latitude |
x | x | m | X coordinate (UTM northing) |
y | y | m | Y coordinate (UTM easting) |
pyrogenic_c | PyC | mg C/g soil | Pyrogenic OC (PyC) |
particulate_org_c | POC | mg C/g soil | Particulate OC (POC) |
soil_org_c | SOC | mg C/g soil | Total organic carbon |
other_org_c | OOC | mg C/g soil | Other OC: The OOC fraction was calculated as the SOC minus POC and PyC fractions |
elevation | ELEV | m | Elevation |
slope_gradient | Slope_Grad | degree | slope gradient |
slope_length | Slope_Leng | m | Slope length |
aspect | Aspect | Aspect | |
h_curvature | H_curvatur | m | Horizontal curvature (Ch) |
v_curvature | V_curvatur | m | Vertical curvature (Cv) |
topo_position_index | TPI | Topographic position index | |
k_factor | K_Factor | Soil erodibility factor | |
ndvi | NDVI | Normalized Difference Vegetation Index | |
mean_ann_precip | MAP | mm | mean annual precipitation |
mean_ann_air_temp | MAT | degrees C | Mean annual air temperature |
silt_clay | Silt_Clay | % | STAGO silt + clay content for surface soil |
topo_slope_position_id | TSP_ID | Topographic slope position ID: Five Topographic position indices (TPI) were calculated at each cell of the DEM | |
fire_regime_groups_id | FRG_ID | Fire Regime Groups ID | |
natl_land_cover_id | NLCD_ID | National Land Cover ID | |
topo_slope_position | TSP | Topographic slope position | |
natl_land_cover | NLCD | National Land Cover | |
fire_regime_groups | FRG | Fire Regime Groups- obtained from the LANDFIRE (LF) project. Fire regimes are classified into 5 groups based on the number of years and severity. Refer to Section 5 in this document | |
fire_regime_groups_descrip | FRG_DESCRI | Fire Regime Groups description-Refer to Section 5 in this document |
Shapefile
SOC_great_plains.zip
The variables and descriptions in the shapefile are provided in Table 2. Note: These data are also provided as a companion file in .kmz format for viewing in Google Earth.
Table 3. Geographic extent of the .shp file
N | S | E | W |
---|---|---|---|
44.98973 | 31.50637 | -94.9154 | -111.012 |
GeoTIFF data files
Each file contains one band (one variable). In all files, the units are mg C/g soil and the no data value is -9999, mapping units are in meters and native data type=float 64.
Table 4. Summary statistics for data in the .tif files
File name | MIn-max values | Std dev | Mean |
---|---|---|---|
SOC_random_forest.tif | 0.7 - 50 | 8 | 12.6 |
pyrogenic_carbon_random_forest.tif | 0.3 - 14.6 | 2 | 3.8 |
particulate_organic_carbon_random_forest.tif | 0 - 12.7 | 1.9 | 2.7 |
other_SOC_random_forest.tif | 1.1 - 20.5 | 3.6 | 5.9 |
Table 5. Geographic extent for all .tif files
N | S | E | W |
---|---|---|---|
45.83028 | 31.22028 | -94.4406 | -111.932 |
Spatial projection information for all .tif files:
PROJCS ['NAD 1983/CONUS Albers', GEOGCS ['NAD83', DATUM ['North_American_Datum_1983', SPHEROID ['GRS 1980',6378137,298.257222101, AUTHORITY ['EPSG','7019']], AUTHORITY['EPSG','6269']],
PRIMEM ['Greenwich',0],
UNIT ['degree',0.0174532925199433],
AUTHORITY ['EPSG','9122']] AUTHORITY ['EPSG','4269']],
PROJECTION ['Albers_Conic_Equal_Area'],
PARAMETER ['standard_parallel_1',29.5], PARAMETER ['standard_parallel_2',45.5],
PARAMETER ['latitude_of_center',23], PARAMETER ['longitude_of_center',-96], PARAMETER ['false_easting',0], PARAMETER ['false_northing',0],
UNIT ['metre',1, AUTHORITY['EPSG','9001']] AUTHORITY['EPSG','5070']]
Application and Derivation
These data could be useful to climate change studies and predicting how land management practices and climate change will affect soil carbon cycling, and improved understanding of factors controlling soil organic carbon fractions at large spatial scales. For these reasons, a fire-prone region was selected for this study with a wide range in elevation, climate, and ecosystem types including forests, grasslands, shrublands, and arable lands comprising the states of Kansas, Colorado, New Mexico, and Wyoming, USA.
Quality Assessment
Prediction data generated from Unscrambler consisted of values with associated deviation or uncertainty values. Samples with outlier data were then excluded, and only samples for which the absolute deviation between the sum of OC in all fractions and the analyzed SOC was within ± 5 mg C g/soil and percent PyC and POC less than 100 were selected. The subset of 473 samples was used for further statistical analysis of PyC and POC. Since the calibration dataset included only Australian soils, the MIR predicted values for total C and SOC were validated using an independent dataset from the United States Geological Survey (Smith et al. 2014).
Spatial distribution
The distributions of POC and PyC in the 473 selected soil samples were positively skewed (skewness>1.5) and showed statistically significant differences (p<0.05) from the normal distribution based on the the Kolmogorov-Smirnov (KS) normality test. The fraction of SOC comprised of PyC was more variable than for other fractions. The NMR-MIR prediction method adopted in this study captures the widest range of PyC among other chemical or spectroscopic methods. This method is expected to generate higher estimates than other PyC quantification approaches, possibly about double the estimates compared to BPCA or oxidative techniques and have been demonstrated to be reliable when properly calibrated (Ahmed et al., 2017).
Data Acquisition, Materials, and Methods
Study Area
Soil samples (650) in this study were from Colorado, Kansas, New Mexico, and Wyoming, collected by the United States Geological Survey (USGS) as a part of the USGS Geochemical Landscapes Project (Smith et al., 2011). The total area of the study region is approximately 1,051,029 km2 and was chosen for its highly variable topography, vegetation zones, climate, and fire regimes. It encompasses the Rocky Mountains in the west, grassland prairie of the Great Plains in the center, and Alluvial River and Osage Plains in the east.
Methods
The 650 samples were collected from soil surface layers of the A horizon using a stratified sampling scheme with sampling densities of one sample per ~1600 km2 (Smith et al., 2011). Sites were not sampled near roads (50 to 200 m depending on road size) or buildings (100 m) or downwind of major industrial sites (5 km). Samples were air dried and sieved through a 2mm stainless steel screen and then crushed to <150 μm in a ceramic mill.
Soil Analyses
The 650 samples were analyzed with mid-infrared (MIR) spectroscopy and partial least squares regression (PLSR) analysis.
- The spectra were truncated, baseline-corrected and mean centered using Unscrambler 10.2 software (CAMO Software AS, Oslo, Norway).
- After this spectral processing, predictions for total organic carbon (SOC), particulate organic carbon (POC), other organic carbon (OOC), and pyrogenic carbon (PyC) were made with Unscrambler, using MIR PLSR calibration models from a large Soil Carbon Research Project (SCaRP) conducted in Australia (Baldock et al., 2013).
MIR PLSR Calibration Samples and Calibration Model
In a set of 312 SCaRP samples:
- The content of soil OC was measured using an automated Dumas combustion carbon analyzer.
- The POC fraction was measured based on the amount of OC retained on a 53 μm sieve.
- PyC was measured using ultraviolet photooxidation and HF treatment followed by solid-state 13C nuclear magnetic resonance (NMR) spectroscopy.
- The OOC fraction was calculated as the soil OC minus POC and PyC fractions.
- MIR PLSR calibration models for POC, OOC and PyC were produced from these 312 samples.
Prediction of Soil Carbon in 473 sample subset
Prediction data generated from Unscrambler consisted of predicted values with associated deviation or uncertainty values which enabled the identification of outlier samples which were excluded from further analysis. The recovery of SOC within the fractions was calculated as a percentage and an absolute deviation where PyC, POC, OOC, and SOC are all expressed in units of mg C/g soil. To create a more robust dataset for further analysis, only samples for which the absolute deviation between the sum of OC in all fractions and the analyzed SOC was within ± 5 mg C/g soil and percent PyC and POC less than 100 were selected; these comprised 73% of the total (473/650). This subset of 473 samples was used for further statistical analysis and mapping of PyC and POC.
MIR PLSR Calibration Note
The calibration dataset used in Unscrambler in the analysis of the 650 samples included only soils from Australia. Therefore, the Great Plains samples MIR predicted values for total C and SOC were validated using an existing independent dataset from the United States Geological Survey (Smith et al., 2014). The Great Plains samples had previously been analyzed for Total C (TC) using an automated carbon analyzer at 1370°C to oxidize C to carbon dioxide (CO2), and the CO2 gas was measured by a solid state infrared detector. The concentration of SOC was calculated by subtracting the amount of inorganic C (carbonate) from TC concentration (Ahmed et al., 2017).
Spatial Mapping of POC and PyC
Random forest regression kriging (RFK) was used to generate prediction maps of POC and PyC concentration at a resolution of 1 km × 1 km throughout forest, planted/cultivated land, grassland/herbaceous land, and shrubland of the study area. See Figure 1.
The 473 samples with predicted carbon fractions were further divided in two random subsets -- 384 samples for initial RFK predictions and 89 for RFK method validation.
The RFK method was evaluated with the validation data (n = 89) to obtain an independent error estimate. SOC and OOC were modeled to calculate the percentage of SOC contributed by each fraction for the prediction grid. An index map of vulnerability was developed to SOC mineralization by defining the grids with POC:PyC values ≤ 0.5 as very low, 0.5–1.0 as low, 1.0–1.5 as medium, 1.5–2.0 as high, and ≥ 2.0 as very high (Ahmed et al., 2017).
Environmental Data
A comprehensive set of spatial environmental data were used in the prediction of SOC, PyC, POC, and OOC throughout the study region including terrain, climate, soil, fire, and land cover described below (Ahmed et al., 2017).
Terrain
A 30 m spatial resolution digital elevation model (DEM) obtained from a USGS database (Multi-Resolution Land Characteristics Consortium, 2007) was obtained and resampled to 90-m resolution. DEM Surface Tools for ArcGIS 10 were used to calculate slope gradient, length, vertical and horizontal curvature, topographic position index (1-5) and slope position. Topographic position indices (TPI) were calculated at each cell of the DEM by calculating the difference between the elevation of the cell and the mean elevation calculated for all cells of a moving rectangular window centered on the cell of interest (TPI values can be negative when the value is lower than their surroundings). Five topographic positions were calculated for grid cells ranging from 250 to 2000 m (TPI250, TPI500, TPI1000, TPI1500, and TPI2000).
Climate
Thirty-year (1971–2000) mean annual air temperature and mean annual precipitation were obtained from the Parameter-elevation Regressions on Independent Slopes Model (PRISM) climate mapping system (Daly et al., 2001); accessed at http://prism.oregonstate.edu/).
Soil Data
Soil data were derived from the Digital General Soil Map of the United States (STATSGO2; map scale 1:250,000). The component-weighted mean of silt + clay content was used for surface soils and the soil erodibility factor (K factor).
Fire Regime Groups
Fire Regime Groups (FRG) data were obtained from the LANDFIRE (LF) project also known as the Landscape Fire and Resource Management Planning Tools Project (accessed at http://www.landfire.gov/datatool.php). The FRG data characterizes the estimated historical fire regimes within landscapes based on interactions between vegetation dynamics, fire spread, fire effects, and spatial context. Fire regimes are classified into five groups or had indeterminate (unknown) fire regime characteristics:
- FRG I ≤35 year fire return interval, low and mixed severity
- FRG II ≤ 35 year fire return interval, replacement severity
- FRG III 35–200 year fire return interval, low and mixed severity
- FRG IV 35–200 year fire return interval, replacement severity
- FRG V>200 year fire return interval and replacement severity
Normalized Difference Vegetation Index
NDVI data for the months of June and July of the year 2000 to 2011, derived from the Moderate-Resolution Imaging Spectroradiometer Bands 1 (red) and 2 (near infrared), were obtained from the NASA Land Processes Distributed Active Archive Center, USGS/Earth Resources Observation and Science Center, Sioux Falls, South Dakota (accessed at https://lpdaac.usgs.gov/get_data).
Land Cover Classes
The National Land Cover Database 2006 (NLCD) 16 land cover classes were reclassified into nine major classes: (1) open water; (2) ice/snow; (2) developed (all developed lands); (4) barren; (5) forest (deciduous, evergreen, and mixed forest); (6) shrubland; (7) grass/herbaceous; (8) planted/cultivated (crops, pasture, and hay); and (9) wetlands (woody and herbaceous wetlands) (Ahmed et al., 2017).
Data Access
These data are available through the Oak Ridge National Laboratory (ORNL) Distributed Active Archive Center (DAAC).
Stocks of Surface Soil Organic Carbon Fractions, Great Plains Region, USA, 2007-2010
Contact for Data Center Access Information:
- E-mail: uso@daac.ornl.gov
- Telephone: +1 (865) 241-3952
References
Ahmed, Z.U., P.B. Woodbury, J. Sanderman, B. Hawke, V. Jauss, D. Solomon, and J. Lehmann (2017), Assessing soil carbon vulnerability in the Western USA by geospatial modeling of pyrogenic and particulate carbon stocks, J. Geophys. Res. Biogeosci., 122, 354-369, https://doi.org/10.1002/2016JG003488
Baldock, J. A., B. Hawke, J. Sanderman, and L. M. Macdonald (2013), Predicting contents of soil carbon and its component fractions in Australian soils from diffuse reflectance mid-infrared spectra, Soil Res., 51, 577–595. https://doi.org/10.1071/SR13077
Daly, C., G. H. Taylor, W. P. Gibson, T. W. Parzybok, G. L. Johnson, and P. Pasteris (2001), High quality spatial climate data sets for the United States and beyond, Trans. ASAE, 43, 1957–1962. https://doi.org/10.13031/2013.3101
Multi-Resolution Land Characteristics Consortium (2007), 2001 National Land Cover Data (NLCD 2006), USEPA, Multi-Resolution Land Characteristics Consortium, Washington, D.C.
Smith, D. B., W. F. Cannon, L. G. Woodruff, F. Solano, K. J. Ellefsen (2014), Geochemical and mineralogical maps for soils of the conterminous United States, U.S. Geological Survey Open-File Report 2014-1082 (386 pp). https://doi.org/10.3133/ofr20141082.
Smith, D. B., W. F. Cannon, and L. G. Woodruff (2011), A national-scale geochemical and mineralogical survey of soils of the conterminous United States, Appl. Geochem., 26, S250–S255. https://doi.org/10.1016/j.apgeochem.2011.03.116
U.S. General Soil Map (STATSGO2) for the United States of America. https://catalog.data.gov/dataset/u-s-general-soil-map-statsgo2-for-the-united-states-of-america