Documentation Revision Date: 2025-01-30
Dataset Version: 1
Summary
Delta-X was a joint airborne and field campaign in the Mississippi River Delta. This campaign conducted both airborne (remote sensing) and field (in situ) measurements to measure hydrology, water quality (e.g., total suspended solids (TSS)), and vegetation structure. These data serve to better understand rates of soil erosion, accretion, and creation in the delta system, with the goal of building better models of how river deltas will behave under relative sea level rise.
There are two data files with this dataset in cloud optimized GeoTIFF (.tif) format; one file each for the Atchafalaya and Terrebonne basins.
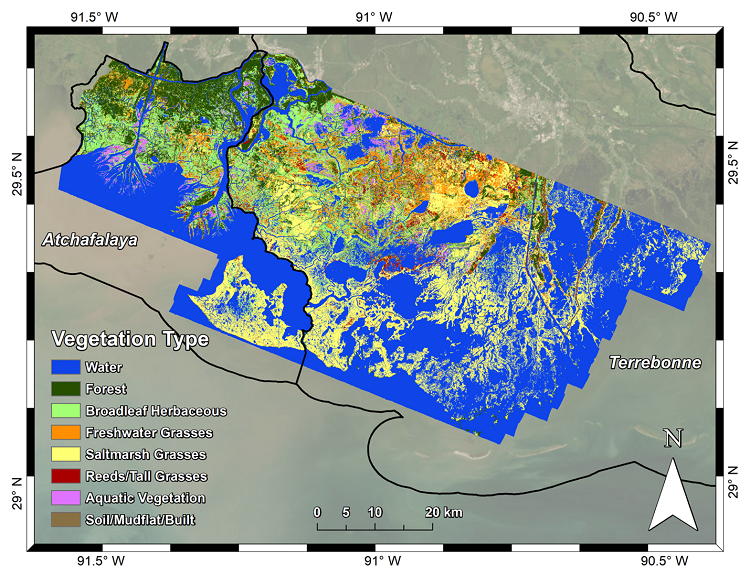
Figure 1. Vegetation type classification of the Atchafalaya and Terrebonne basins derived from AVIRIS-NG.
Citation
Jensen, D.J., E. Castañeda-Moya, E. Solohin, D.R. Thompson, and M. Simard. 2024. Delta-X AVIRIS-NG L3 Derived Vegetation Types, MRD, Louisiana, USA. ORNL DAAC, Oak Ridge, Tennessee, USA. https://doi.org/10.3334/ORNLDAAC/2352
Table of Contents
- Dataset Overview
- Data Characteristics
- Application and Derivation
- Quality Assessment
- Data Acquisition, Materials, and Methods
- Data Access
- References
Dataset Overview
This dataset provides maps of vegetation types for the Atchafalaya and Terrebonne basins in coastal Louisiana, U.S., derived from NASA’s Next Generation Airborne Visible Infrared Imaging Spectrometer (AVIRIS-NG) imagery acquired during spring and fall of 2021 for the Delta-X campaign. Vegetation types were classified from Level-2B BRDF-adjusted surface reflectance (Thompson et al. 2023). Local pixel reflectance spectra coincident with herbaceous vegetation field samples (Castañeda-Moya and Solohin, 2023) and vegetation plot data from Louisiana’s Coastwide Reference Monitoring System (CRMS; https://cims.coastal.louisiana.gov/) were used to generate a machine learning-based model to classify vegetation types. This model was then applied to the AVIRIS-NG mosaic imagery to map vegetation types across the Atchafalaya and Terrebonne Basins.
AVIRIS-NG acquired data over regions of interest in the Atchafalaya and Terrebonne basins. Multiple aircraft overflights covered the region of interest in strips to accumulate a combined map of the target area. For this campaign, AVIRIS-NG was implemented on a Dynamic Aviation King Air B200. The L2B BRDF-adjusted surface reflectance product (Thompson et al. 2023) was created from imagery that was atmospherically corrected to produce Hemispherical-Directional surface reflectance datasets (Thompson et al., 2018, 2019) followed by corrections for BRDF-effects and sun-glint over land and water pixels, respectively (Queally et al., 2021; Greenberg et al., 2022).
Project: Delta-X
The Delta-X mission is a 5-year NASA Earth Venture Suborbital-3 mission to study the Mississippi River Delta in the United States, which is growing and sinking in different areas. River deltas and their wetlands are drowning as a result of sea level rise and reduced sediment inputs. The Delta-X mission will determine which parts will survive and continue to grow, and which parts will be lost. Delta-X begins with airborne and in situ data acquisition and carries through data analysis, model integration, and validation to predict the extent and spatial patterns of future deltaic land loss or gain.
Related Datasets
Castañeda, E., A.I. Christensen, M. Simard, A. Bevington, R. Twilley, and A. Mccall. 2020. Pre-Delta-X: Vegetation Species, Structure, Aboveground Biomass, MRD, LA, USA, 2015. ORNL DAAC, Oak Ridge, Tennessee, USA. https://doi.org/10.3334/ORNLDAAC/1805
Castañeda-Moya, E., and E. Solohin. 2023. Delta-X: Aboveground Biomass and Necromass across Wetlands, MRD, Louisiana, 2021, V2. ORNL DAAC, Oak Ridge, Tennessee, USA. https://doi.org/10.3334/ORNLDAAC/2237
Jensen, D.J., M. Simard, R. Twilley, E. Castaneda, and A. McCall. 2021. Pre-Delta-X: Aboveground Biomass and Vegetation Maps, Wax Lake Delta, LA, USA, 2016. ORNL DAAC, Oak Ridge, Tennessee, USA. https://doi.org/10.3334/ORNLDAAC/1821
Jensen, D.J., E. Castañeda-Moya, E. Solohin, A. Rovai, D.R. Thompson, and M. Simard. 2023. Delta-X: AVIRIS-NG L3 Derived Aboveground Biomass, MRD, Louisiana, USA, 2021, V2. ORNL DAAC, Oak Ridge, Tennessee, https://doi.org/10.3334/ORNLDAAC/2138
Thompson, D.R., D.J. Jensen, J.W. Chapman, M. Simard, and E. Greenberg. 2023. Delta-X: AVIRIS-NG L2B BRDF-Adjusted Surface Reflectance, MRD, LA, 2021, V2. ORNL DAAC, Oak Ridge, Tennessee, USA. https://doi.org/10.3334/ORNLDAAC/2139
Acknowledgement
This work was supported by NASA's Earth Venture Suborbital-3 program (grant NNH17ZDA001N-EVS3) .
Data Characteristics
Spatial Coverage: Atchafalaya and Terrebonne basins, southern coast of Louisiana, USA
Spatial Resolution: 4.8 m
Temporal Coverage: 2021-08-20 to 2021-08-25
Temporal Resolution: One time
Site Boundaries: Latitude and longitude are given in decimal degrees.
Site | Westernmost Longitude | Easternmost Longitude | Northernmost Latitude | Southernmost Latitude |
---|---|---|---|---|
Atchafalaya and Terrebonne basins, Louisiana, US | -91.5934 | -90.3607 | 29.7070 | 28.9907 |
Data File Information
There are two data files with this dataset in GeoTIFF (.tif) format; one file each for the Atchafalaya and Terrebonne basins:
ang20210820-25_VegClass_Atcha.tif and ang20210820-25_VegClass_Terre.tif.
GeoTIFF characteristics:
- Coordinate system: projected into UTM zone 15N, WGS 84 datum (EPSG: 32615); units = m.
- Number of bands: 1
- Pixel values: vegetation type classes indicated by numeric codes (Table 1)
- Nodata value: -9999
Table 1. Vegetation types in GeoTIFFs with representative species.
Pixel value | Vegetation type | Vegetation species |
---|---|---|
0 | Water | - |
1 | Forest | Acer rubrum, Salix nigra, Morella cerifera, Nyssa aquatica, Triadica sebifera, Avicennia germinans |
2 | Broadleaf herbaceous | Sagittaria lancifolia, Vigna luteola, Colacasia esculenta, Polygonum punctatum, Murdannia keisak, Thelypteris palustris |
3 | Freshwater grasses | Panicum hemitomon, Schoenoplectus californicus, Luziola peruviana, Eleocharis montana, Eleocharis R. Br. |
4 | Saltmarsh grasses | Spartina patens, Spartina alterniflora, Lythrum lineare, Spartina cynosuroides, Juncus roemarianus |
5 | Reeds/Tall grasses | Phragmites australis, Typha domingensis, Typha latifolia, Zizaniopsis miliacea |
6 | Aquatic vegetation | Ludwigia grandiflora, Nelumbo lutea, Eichornia crassipes |
7 | Soil/Mudflat/Built | - |
Application and Derivation
Vegetation type and landcover are key components for assessing distributions of different plant types, mapping plant traits and structure across a region, and parameterizing models that are impacted by those characteristics. These maps of vegetation type accordingly show the distribution of different functional groups across the Atchafalaya and Terrebonne basins and how they vary with the basins’ salinity gradient. These maps are additionally used in the application of reflectance-based models of plant biomass, necromass, and primary productivity (Jensen et al., 2023), and can be further applied to parameterize hydrodynamic models.
Quality Assessment
Uncertainty in the vegetation classification model was characterized by comparing the map results to the validation dataset (see Section 5 below). A confusion matrix was produced for all classified vegetation types and the soil/mudflat/built class (Table 2) and used to calculate producer’s and user’s accuracy for each class, along with the overall accuracy and Cohen’s Kappa value (Table 3). The classification results attained an overall accuracy of 85.03% and a Kappa of 0.81.
Table 2. Confusion matrix for the validation subset of data points extracted from the Delta-X field samples and the CRMS vegetation dataset.
Reference Data | ||||||||
---|---|---|---|---|---|---|---|---|
Classification Data | Forest | Broadleaf Herbaceous | Freshwater Grasses | Saltmarsh Grasses | Reeds / Tall Grasses |
Aquatic Vegetation | Soil / Mudflat / Built | All |
Forest | 16 | 1 | 0 | 1 | 1 | 0 | 0 | 19 |
Broadleaf Herbaceous | 0 | 12 | 2 | 1 | 0 | 2 | 0 | 17 |
Freshwater Grasses | 1 | 3 | 14 | 3 | 0 | 0 | 0 | 21 |
Saltmarsh Grasses | 0 | 0 | 0 | 57 | 0 | 0 | 0 | 57 |
Reeds/ Tall Grasses |
0 | 0 | 2 | 5 | 18 | 0 | 0 | 25 |
Aquatic Vegetation | 0 | 2 | 0 | 0 | 0 | 11 | 0 | 13 |
Soil/Mudflat/Built | 0 | 0 | 0 | 1 | 0 | 0 | 14 | 15 |
All | 17 | 18 | 18 | 68 | 19 | 13 | 14 | 167 |
Table 3. Accuracy assessment metrics calculated from the Table 2 confusion matrix.
Vegetation Type | Producer's Accuracy (%) | User's Accuracy (%) |
---|---|---|
Forest | 94.12 | 84.21 |
Broadleaf Herbaceous | 66.67 | 70.59 |
Freshwater Grasses | 77.78 | 66.67 |
Saltmarsh Grasses | 83.82 | 100.00 |
Reeds/Tall Grasses | 94.74 | 72.00 |
Aquatic Vegetation | 84.62 | 84.62 |
Soil/Mudflat/Built | 100.00 | 93.33 |
Overall Accuracy | 85.03 % | |
Kappa | 0.81 |
Data Acquisition, Materials, and Methods
AVIRIS-NG, the Next Generation Airborne Visible/Infrared Imaging Spectrometer, is a pushbroom spectral mapping system with a high signal-to-noise ratio (SNR) designed for high performance spectroscopy. AVIRIS-NG was developed as a successor to the Classic Airborne Visible Infrared Imaging Spectrometer (AVIRIS-C) (Green et al., 1998). The instrument covers the entire solar reflected spectrum from 380-2510 nm with a single Focal Plane Array (FPA), at a spectral sampling of approximately 5 nm. The AVIRIS- NG sensor has a 1 milliradian instantaneous field of view, providing altitude-dependent ground sampling distance ranging from sub-meter to 20 m scales. Its detector has a 640×480-pixel array, from which standard products are generated using the sensor’s 600 cross-track spatial samples and 425 spectral samples. Each acquisition is a “flight line” forming a continuous strip of pushbroom data that typically takes 1-10 minutes to acquire. Multiple aircraft overflights cover the region of interest in these strips, accumulating a combined map of the target area. For this campaign, AVIRIS-NG was implemented on a Dynamic Aviation King Air B200. The instrument has four components: 1) a sensor with its mount and camera glass mounted at a nadir port; 2) an onboard calibrator (OBC), mounted in the cabin next to the sensor; 3) a forward operator electronics rack, and 4) an aft thermal-control electronics rack. Each AVIRIS-NG flightline was atmospherically corrected to produce Hemispherical-Directional surface reflectance datasets (Thompson et al., 2018, 2019), followed by corrections BRDF-effects and sun-glint over land and water pixels, respectively (Queally et al., 2021, Greenberg et al., 2022), to generate the L2B BRDF-adjusted surface reflectance product (Thompson et al. 2023) used for this vegetation classification.
A principal components analysis (PCA) of the AVIRIS-NG data was performed based on a PCA rotation calculated from our compiled spectral library of vegetation points. Thirteen final components were selected based on their explained variance (99.86% of the variance in the 300 selected bands explained in total) and lack of visible discrepancies between the mosaicked flightlines.
Corrected pixel reflectance values coincident with herbaceous vegetation field samples were extracted from both March-April and August 2021 surveys (Castañeda-Moya and Solohin, 2023). The dominant species of herbaceous vegetation at each point was paired with the corresponding pixel’s reflectance spectrum. All herbaceous and forested wetland vegetation plot data were added from Louisiana’s Coastwide Reference Monitoring System (CRMS; https://cims.coastal.louisiana.gov/) from Summer 2021 to this dataset, selecting only plots coincident with the AVIRIS-NG imagery and with coverage of a dominant plant species over 50%. In addition, persistent forest points from the Pre-Delta-X vegetation survey were added (Castañeda et al., 2020) along with manually selected soil, mudflat, and built surface points. The identified species at each plot were grouped into the following classes: Forest, Broadleaf Herbaceous, Saltmarsh Grass (including brackish species), Freshwater Grass, Reeds/Tall Grasses, and Aquatic Vegetation (including floating and submerged aquatic vegetation) (Table 1).
The vegetation data and their corresponding spectra were used to generate a machine learning model to classify vegetation types and land cover on a per-pixel basis. From the vegetation dataset, 504 points were selected for model development. Of these, two thirds were randomly subset for a training dataset (n=337) dataset, and the remainder were allocated to a validation dataset (n=167). A Random Forests classification model was then trained on 13 PCA bands calculated from the training dataset’s associated reflectance spectra.
This classification model was then applied to land pixels in the PCA bands derived from the August 2021 Atchafalaya and Terrebonne mosaics to classify the imagery (Thompson et al. 2023). Water masks were applied to classify only land pixels. Clouded and shadowed areas were filled with classification results from a follow-on AVIRIS-NG survey conducted from September 23-25, 2021, using the same Random Forests model. A majority filter using four adjacent pixels was applied to each classified land pixel to produce final vegetation type maps.
Data Access
These data are available through the Oak Ridge National Laboratory (ORNL) Distributed Active Archive Center (DAAC).
Delta-X AVIRIS-NG L3 Derived Vegetation Types, MRD, Louisiana, USA
Contact for Data Center Access Information:
- E-mail: uso@daac.ornl.gov
- Telephone: +1 (865) 241-3952
References
Castañeda-Moya, E., and E. Solohin. 2023. Delta-X: Aboveground Biomass and Necromass across Wetlands, MRD, Louisiana, 2021, V2. ORNL DAAC, Oak Ridge, Tennessee, USA. https://doi.org/10.3334/ORNLDAAC/2237
Castañeda, E., A.I. Christensen, M. Simard, A. Bevington, R. Twilley, and A. Mccall. 2020. Pre-Delta-X: Vegetation Species, Structure, Aboveground Biomass, MRD, LA, USA, 2015. ORNL DAAC, Oak Ridge, Tennessee, USA. https://doi.org/10.3334/ORNLDAAC/1805
Green, R.O., M.L. Eastwood, C.M. Sarture, T.G. Chrien, M. Aronsson, B.J. Chippendale, J.A. Faust, B.E. Pavri, C.J. Chovit, M. Solis, M.R. Olah, and O. Williams. 1998. Imaging Spectroscopy and the Airborne Visible/Infrared Imaging Spectrometer (AVIRIS). Remote Sensing of Environment 65:227-248. https://doi.org/10.1016/S0034-4257(98)00064-9
Greenberg, E., D.R. Thompson, D. Jensen, P.A. Townsend, N. Queally, A. Chlus, C.G. Fichot, J.P. Harringmeyer, and M. Simard. 2022. An improved scheme for correcting remote spectral surface reflectance simultaneously for terrestrial BRDF and water-surface sunglint in coastal environments. Journal of Geophysical Research: Biogeosciences 127:e2021JG006712. https://doi.org/10.1029/2021JG006712
Jensen, D.J., E. Castañeda-Moya, E. Solohin, A. Rovai, D.R. Thompson, and M. Simard. 2023. Delta-X: AVIRIS-NG L3 Derived Aboveground Biomass, MRD, Louisiana, USA, 2021, V2. ORNL DAAC, Oak Ridge, Tennessee, USA. https://doi.org/10.3334/ORNLDAAC/2138
Jensen, D.J., M. Simard, R. Twilley, E. Castaneda, and A. McCall. 2021. Pre-Delta-X: Aboveground Biomass and Vegetation Maps, Wax Lake Delta, LA, USA, 2016. ORNL DAAC, Oak Ridge, Tennessee, USA. https://doi.org/10.3334/ORNLDAAC/1821
Queally, N., Z. Ye, T. Zheng, A. Chlus, F. Schneider, R.P. Pavlick, and P.A. Townsend. 2022. FlexBRDF: A Flexible BRDF Correction for Grouped Processing of Airborne Imaging Spectroscopy Flightlines. Journal of Geophysical Research: Biogeosciences 127:e2021JG006622. https://doi.org/10.1029/2021JG006622
Thompson, D.R., V. Natraj, R.O. Green, M.C. Helmlinger, B.C. Gao, and M.L. Eastwood. 2018. Optimal estimation for imaging spectrometer atmospheric correction. Remote sensing of environment, 216:355-373. https://doi.org/10.1016/j.rse.2018.07.003
Thompson, D.R., K. Cawse-Nicholson, Z. Erickson, C.G. Fichot, C. Frankenberg, B.-C. Gao, M.M. Gierach, R.O. Green, D. Jensen, V. Natraj, and A. Thompson. 2019. A unified approach to estimate land and water reflectances with uncertainties for coastal imaging spectroscopy. Remote Sensing of Environment 231:111198. https://doi.org/10.1016/j.rse.2019.05.017
Thompson, D.R., D.J. Jensen, J.W. Chapman, M. Simard, and E. Greenberg. 2023. Delta-X: AVIRIS-NG L2B BRDF-Adjusted Surface Reflectance, MRD, LA, 2021, V2. ORNL DAAC, Oak Ridge, Tennessee, USA. https://doi.org/10.3334/ORNLDAAC/2139