Documentation Revision Date: 2021-03-22
Dataset Version: 1.1
Summary
The largest mean aboveground biomass concentrations were found in the Chesapeake Bay (1036.8 g/m2), across the large extent of the Terrebonne and St. Mary Parishes in Louisiana (820.9 g/m2) and in the San Francisco Bay region (812.1 g/m2).
There are six files in GeoTIFF format in this dataset; one file for each of the six estuarine study areas.
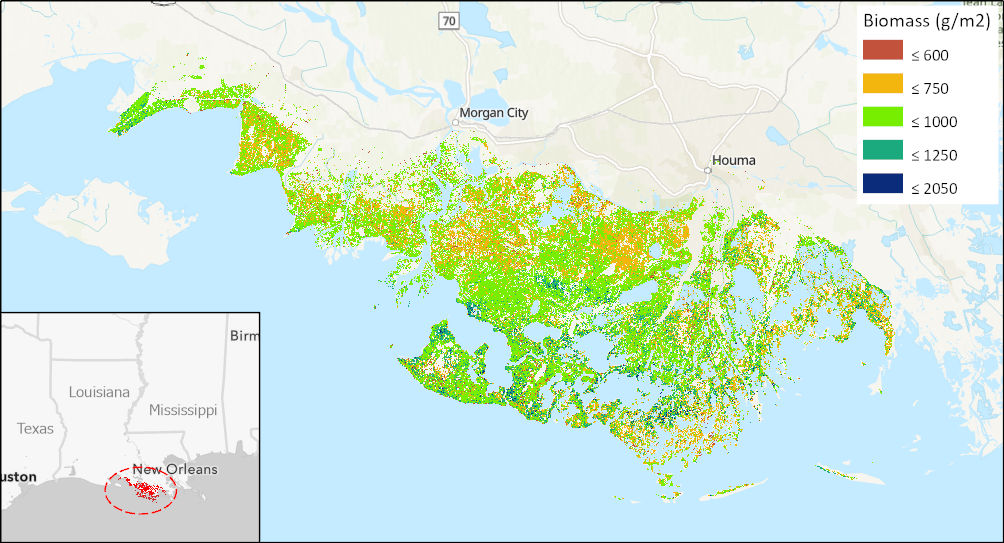
Figure 1. The largest present-day amount of tidal vegetation biomass were found across the Terrebonne and St. Mary Parishes of the Mississippi River Delta in Louisiana. (Source Louisiana_biomass_2015.tif)
Citation
Byrd, K.B., L. Ballanti, N. Thomas, D. Nguyen, J.R. Holmquist, M. Simard, and L. Windham-Myers. 2021. Aboveground Biomass High-Resolution Maps for Selected US Tidal Marshes, 2015. ORNL DAAC, Oak Ridge, Tennessee, USA. https://doi.org/10.3334/ORNLDAAC/1879
Table of Contents
- Dataset Overview
- Data Characteristics
- Application and Derivation
- Quality Assessment
- Data Acquisition, Materials, and Methods
- Data Access
- References
- Dataset Revisions
Dataset Overview
This dataset provides maps of aboveground tidal marsh biomass (g/m2) at 30 m resolution for six estuarine regions of the conterminous United States: Cape Cod, MA; Chesapeake Bay, MD, Everglades, FL; Mississippi Delta, LA; San Francisco Bay, CA; and Puget Sound, WA. Estuarine and palustrine emergent tidal marsh areas were based on a 2010 NOAA Coastal Change Analysis Program (C-CAP) map. Aboveground biomass maps were generated from a random forest model driven by Landsat vegetation indices and a national scale dataset of field-measured aboveground biomass. The final model, driven by six Landsat vegetation indices, with the soil adjusted vegetation index as the most important, successfully predicted biomass for a range of marsh plant functional types defined by height, leaf angle, and growth form. Biomass can be converted to carbon stocks using a mean plant carbon content of 44.1%.
The largest mean aboveground biomass concentrations were found in the Chesapeake Bay (1036.8 g/m2), across the large extent of the Terrebonne and St. Mary Parishes in Louisiana (820.9 g/m2) and in the San Francisco Bay region (812.1 g/m2).
User Note: This Version 1.1 includes revised biomass estimates for all study areas. See Byrd et al. (2020) for descriptions of the updated results from the revised models.
Project: Carbon Monitoring System
The NASA Carbon Monitoring System (CMS) is designed to make significant contributions in characterizing, quantifying, understanding, and predicting the evolution of global carbon sources and sinks through improved monitoring of carbon stocks and fluxes. The System will use the full range of NASA satellite observations and modeling/analysis capabilities to establish the accuracy, quantitative uncertainties, and utility of products for supporting national and international policy, regulatory, and management activities. CMS will maintain a global emphasis while providing finer scale regional information, utilizing space-based and surface-based data and will rapidly initiate generation and distribution of products both for user evaluation and to inform near-term policy development and planning.
Related Publications:
Byrd, K. B., Ballanti, L., Thomas, N., Nguyen, D., Holmquist, J. R., Simard, M., Windham-Myers, L. 2018. A remote sensing-based model of tidal marsh aboveground carbon stocks for the conterminous United States. ISPRS Journal of Photogrammetry and Remote Sensing. 139, 255-271. https://doi.org/10.1016/j.isprsjprs.2018.03.019
Byrd, K. B., L. Ballanti, N. Thomas, D. Nguyen, J. R. Holmquist, M. Simard, and L. Windham-Myers. 2020. Corrigendum to “A remote sensing-based model of tidal marsh aboveground carbon stocks for the conterminous United States” [ISPRS J. Photogram. Rem. Sens. 139 (2018) 255–271]. ISPRS Journal of Photogrammetry and Remote Sensing 166:63-67. https://doi.org/10.1016/j.isprsjprs.2020.05.005.
Related Datasets:
Ballanti, L., and K.B. Byrd. 2018. Green Vegetation Fraction High-Resolution Maps for Selected US Tidal Marshes, 2015. ORNL DAAC, Oak Ridge, Tennessee, USA. https://doi.org/10.3334/ORNLDAAC/1608
Ballanti, L., and K.B. Byrd. 2018. Vegetation and Open Water High-Resolution Maps for Selected US Tidal Marshes, 2015. ORNL DAAC, Oak Ridge, Tennessee, USA. https://doi.org/10.3334/ORNLDAAC/1609
Feagin, R.A., I. Forbrich, T.P. Huff, J.G. Barr, J. Ruiz-plancarte, J.D Fuentes, R.G. Najjar, R. Vargas, A. Vazquez-lule, L. Windham-Myers, K. Kroeger, E.J. Ward, G.W. Moore, M. Leclerc, K.W. Krauss, C.L. Stagg, M. Alber, S.H. Knox, K.V.R. Schafer, T.S. Bianchi, J.A. Hutchings, H.B. Nahrawi, A. Noormets, B. Mitra, A. Jaimes, A.L. Hinson, B. Bergamaschi, J. King, and G. Miao. 2020. Gross Primary Production Maps of Tidal Wetlands across Conterminous USA, 2000-2019. ORNL DAAC, Oak Ridge, Tennessee, USA. https://doi.org/10.3334/ORNLDAAC/1792
Holmquist, J.R., L. Windham-Myers, N. Bliss, S. Crooks, J.T. Morris, P.J. Megonigal, T. Troxler, D. Weller, J. Callaway, J. Drexler, M.C. Ferner, M.E. Gonneea, K. Kroeger, L. Schile-beers, I. Woo, K. Buffington, B.M. Boyd, J. Breithaupt, L.N. Brown, N. Dix, L. Hice, B.P. Horton, G.M. Macdonald, R.P. Moyer, W. Reay, T. Shaw, E. Smith, J.M. Smoak, C. Sommerfield, K. Thorne, D. Velinsky, E. Watson, K. Grimes, and M. Woodrey. 2019. Tidal Wetland Soil Carbon Stocks for the Conterminous United States, 2006-2010. ORNL DAAC, Oak Ridge, Tennessee, USA. https://doi.org/10.3334/ORNLDAAC/1612
Additional datasets are available from the ORNL DAAC's Carbon Monitoring System page.
Acknowledgement: This work was funded by NASA CMS Grant NNH14AY671 and the U.S. Geological Survey Land Change Science Program and LandCarbon Program.
Data Characteristics
Spatial Coverage: Six estuarine study regions across the conterminous US.
Spatial resolution: 30 m
All files are in the Universal Transverse Mercator (UTM) conformal projection system, each defined within a standard zone
Temporal coverage: 2015-08-01 to 2015-09-01.
Temporal resolution: One-time estimate.
Study Areas (All latitude and longitude given in decimal degrees)
Sites | Westernmost Longitude | Easternmost Longitude | Northernmost Latitude | Southernmost Latitude |
---|---|---|---|---|
Cape Cod | -70.7021 | -69.9267 | 42.0848 | 41.5152 |
Chesapeake Bay | -76.7252 | -76.3886 | 39.2384 | 38.7114 |
Everglades | -81.4637 | -80.3942 | 25.8899 | 25.0924 |
Louisiana | -91.8879 | -90.3631 | 29.8467 | 29.0374 |
Puget Sound | -122.7326 | -122.6658 | 47.1174 | 47.0678 |
San Francisco Bay | -122.6163 | -121.2325 | 38.3264 | 37.6605 |
Data File Information
There are six data files in GeoTIFF (.tif) format, one for each of the six estuarine study areas across the conterminous US. Each file is named as studyarea_biomass.tif. Raster cell size is 30 m x 30 m, and the units for cell values are grams per square meter (g m-2). Each file is in a UTM projection; the UTM zone matches its location. The data type is Float32, and the no data value is -9999.
Table 1. Filenames and description. All maps display aboveground biomass for tidal marshes in six study areas in the continental United States. Minimum, maximum, and mean cell values (in g m-2) are listed, along with coordinate system information (EPSG code, UTM zone).
Filenames | Study Area | Minimum | Maximum | Mean | EPSG | UTM zone |
---|---|---|---|---|---|---|
CapeCod_biomass_2015.tif | Cape Cod, Massachuttes | 184.9 | 1383.2 | 694.1 | 32619 | 19N |
Chesapeake_biomass_2015.tif | Chespeake Bay, Maryland | 319.3 | 1778.9 | 1036.8 | 32618 | 18N |
Everglades_biomass_2015.tif | Everglades, Florida | 7.0 | 699.2 | 237.8 | 32617 | 17N |
Louisiana_biomass_2015.tif | Terrebonne and St Mary Parishes of the Mississippi River Delta, Louisiana | 287.2 | 1632.7 | 820.9 | 32615 | 15N |
PugetSound_biomass_2015.tif | Puget Sound, Washington | 59.9 | 805.6 | 498.6 | 32610 | 10N |
SFBay_biomass_2015.tif | San Francisco Bay, California | 261.3 | 2035.3 | 812.1 | 32610 | 10N |
Application and Derivation
Remote sensing-based maps of tidal marshes, both of their extents and carbon stocks, have the potential to play a key role in conducting greenhouse gas inventories and implementing climate mitigation policies.
Remote sensing models (e.g., Byrd et al. 2018) can be used to develop national-level inventories of natural systems critical to carbon cycling and to aid in implementation of projects approved in the voluntary carbon markets. Given global coverage of Landsat, this approach can likely be expanded internationally as well. Although high resolution imagery such as NAIP is not available globally, Landsat-only models will likely provide suitable results, particularly in fully vegetated areas. Newly available global base maps of salt marshes (Mcowen et al. 2017) will also aid global implementation. Archived Landsat imagery can be used for historical estimates of aboveground C flux. Future trends can be calculated as well with the development of a 2015 C-CAP landcover map and continuation of Landsat satellites.
Quality Assessment
A multivariate delta method was used to calculate uncertainties in regional carbon densities and stocks (Byrd et al. 2018). The method considered standard errors in map area, mean biomass, mean %C per region, and wetland type. The random forest model was assessed for error by considering RMSE, and percent normalized RMSE of cross-validation prediction values, as well as model bias. The best model predicted aboveground biomass from Landsat data with an overall RMSE of 464 g m-2 (Byrd et al. 2020). Uncertainties were low for estimates of carbon density (standard errors: 0.006 - 0.086 Mg ha-1). Uncertainty in estimates of total aboveground carbon stocks was mainly influenced by error in estimating the mapped area (Table 8, Byrd et al. 2020).
After the publication of Byrd et al. (2018), the authors discovered a discrepancy in field biomass data used as dependent variables in their modeling efforts. After correcting this error, revised results are published in Byrd et al. (2020). This dataset was produced from the revised models.
Data Acquisition, Materials, and Methods
Study Sites
The study regions include:
- Cape Cod peninsula, Massachusetts
- Smithsonian Environmental Research Center Global Change Research Wetland (GCReW) on Chesapeake Bay in Anne Arundel County, Maryland
- Florida Coastal Everglades Long Term Ecological Research Site in Everglades National Park, Florida
- Terrebonne and Saint Mary Parishes, Louisiana, in the Mississippi Delta
- Billy Frank Jr. Nisqually National Wildlife Refuge (NWR) in Puget Sound, Washington
- San Francisco Bay, California, which was split into two sub-regions, the saline/brackish marshes and the freshwater marshes.
The specific locations of the estuarine and palustrine emergent tidal marshes in the study regions were as indicated by a modified NOAA Coastal Change Analysis Program (C-CAP) map (NOAA Office for Coastal Management 2015).
Data Sources and Processing
The investigators developed a U.S. national-scale remote sensing model of tidal marsh aboveground C stocks based on freely available satellite (Landsat and Sentinel-1) and airborne imagery (NAIP). Field-based measurements of aboveground biomass and plant %C served as dependent variables for the model development. Alternative models were evaluated using a random forest machine learning algorithm. Uncertainties in C stock estimates were calculated through propagation of error in biomass, %C, and C-CAP tidal marsh map area.
Details of data sources and processing may be found in the Byrd et al. (2018).
User Note: Time and effort in development of the remote sensing dataset used in this study was greatly reduced by taking advantage of the parallel processing and data archive available with Google Earth Engine (GEE). The GEE platform allowed for fast access to the massive archive of satellite images, and once the custom code was developed and tested, it allowed for fast, repeatable, automated extraction of and computations on pixel values from such images. Although GEE also contains collections of Sentinel-1 (though incomplete as of May 2017) and NAIP, we primarily used GEE’s Landsat imagery collections, which GEE kept current on a more regular basis, to build the Biomass/Remote Sensing dataset (Byrd et al., 2017). Despite the advantages of GEE for building large datasets however, in this study it was determined that some post-processing steps were best performed outside the GEE environment. See Supplementary Data S1 of Byrd et al. (2018) for the GEE code.
Figure 2. Photos of study regions with photo credits, from Byrd et al. (2018). (A) Puget Sound, Washington, Laurel Ballanti; (B) Cape Cod, Massachusetts, Lia McLaughlin; (C) Chesapeake Bay, Maryland, Grace Schwartz; (D) Everglades, Florida, paulsmithrj, CC BY-SA 3.0; (E) Mississippi River Delta, Louisiana, Greg Snedden; (F) San Francisco Bay, California, Jessica Schneider.
Data Access
These data are available through the Oak Ridge National Laboratory (ORNL) Distributed Active Archive Center (DAAC).
Aboveground Biomass High-Resolution Maps for Selected US Tidal Marshes, 2015
Contact for Data Center Access Information:
- E-mail: uso@daac.ornl.gov
- Telephone: +1 (865) 241-3952
References
Byrd, K. B., L. Ballanti, N. Thomas, D. Nguyen, J. R. Holmquist, M. Simard, and L. Windham-Myers. 2020. Corrigendum to “A remote sensing-based model of tidal marsh aboveground carbon stocks for the conterminous United States” [ISPRS J. Photogram. Rem. Sens. 139 (2018) 255–271]. ISPRS Journal of Photogrammetry and Remote Sensing 166:63-67. https://doi.org/10.1016/j.isprsjprs.2020.05.005.
Byrd, K. B., Ballanti, L., Thomas, N., Nguyen, D., Holmquist, J. R., Simard, M., Windham-Myers, L. 2018. A remote sensing-based model of tidal marsh aboveground carbon stocks for the conterminous United States. ISPRS Journal of Photogrammetry and Remote Sensing. 139, 255-271. https://doi.org/10.1016/j.isprsjprs.2018.03.019
Byrd, K., Ballanti, L., Thomas, N., Nguyen, D., Holmquist, J.R., Simard, M., Windham-Myers, L., Schile, L., Parker, V.T., Callaway, J.C., Vasey, M.C., Herbert, E.R., Davis, M., Woo, I., Cruz, S.D.L., Kroeger, K., Gonneea, M., Suttles, J.O.K., Megonigal, P., Lu, M., McFarland, L., Brooks, H., Drake, B., Peresta, G., Peresta, A., Troxler, T., Castaneda, E., 2017. Tidal marsh biomass field plot and remote sensing datasets for six regions in the conterminous United States. U.S. Geolog. Surv. Data Release. https://doi.org/10.5066/F77943K8
Mcowen, C.J., Weatherdon, L.V., Bochove, J.-W.V., Sullivan, E., Blyth, S., Zockler, C., Stanwell-Smith, D., Kingston, N., Martin, C.S., Spalding, M., Fletcher, S., 2017. A global map of saltmarshes. Biodivers. Data J. 5. https://doi.org/10.3897/BDJ.5.e11764
NOAA Office for Coastal Management, 2015. NOAA Coastal Change Analysis Program (C-CAP) Regional Land Cover Database. Data collected 1995-present. Data accessed at www.coast.noaa.gov/digitalcoast/data/ccapregional, Charleston.
Dataset Revisions
Version | Release Date | Revision Notes | Status |
---|---|---|---|
1.1 | 2021-03-22 | Corrects Version 1.0 | Current version |
1 | 2018-12-11 | Initial release | Now superseded |
User Note: This dataset includes revised biomass estimates for all study areas. After the publication of Byrd et al. (2018), the authors discovered a problem with their field data for the San Francisco Bay, CA, which produced biased parameters and biomass estimates. After correcting this error and reevaluating their models, the overall model structure remained unchanged but parameter values, and therefore, biomass estimates were altered (Byrd et al. 2020). See Byrd et al. (2020) for updated results from the revised models.