Documentation Revision Date: 2024-03-21
Dataset Version: 1
Summary
There are two files in this dataset: a cloud optimized GeoTIFF format and a shapefile in a compressed zip archive.
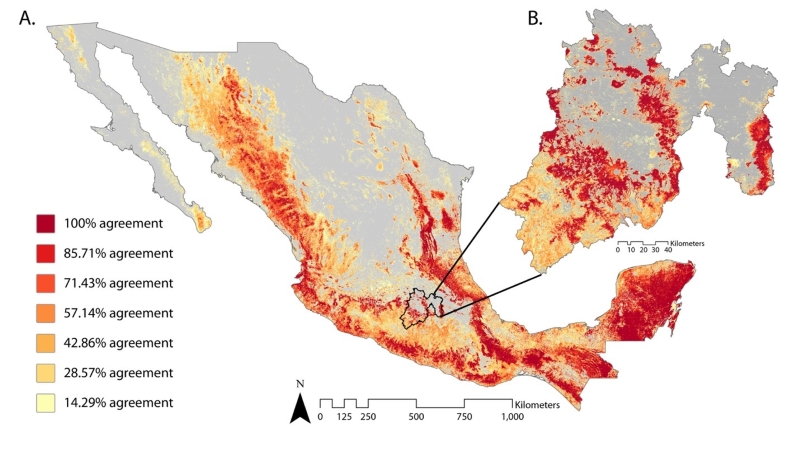
Figure 1. Percent agreement among seven satellite-based forest cover products (A). The percentage represents how many products identified each pixel as being forested. An enlarged representation of Edomex (Estado de Mexico) for the forest extent agreement map (B). Source: Braden et al. (2024).
Citation
Braden, D., P. Mondal, T. Park, J.A.A. De la rosa, M.I.A. Leal, R.A.C. Lara, R.M. Saucedo, V.M. Salas-Aguilar, M.A. Soriano-Luna, and R. Vargas. 2023. Satellite-Derived Forest Extent Likelihood Map for Mexico. ORNL DAAC, Oak Ridge, Tennessee, USA. https://doi.org/10.3334/ORNLDAAC/2320
Table of Contents
- Dataset Overview
- Data Characteristics
- Application and Derivation
- Quality Assessment
- Data Acquisition, Materials, and Methods
- Data Access
- References
Dataset Overview
This dataset provides a comparison of forest extent agreement from seven remote sensing-based products across Mexico. These satellite-derived products include European Space Agency 2020 Land Cover Map for Mexico (ESA), Globeland30 2020 (Globeland30), Commission for Environmental Cooperation 2015 Land Cover Map (CEC), Impact Observatory 2020 Land Cover Map (IO), NAIP Trained Mean Percent Cover Map (NEX-TC), Global Land Analysis and Discovery Global 2010 Tree Cover (Hansen-TC), and Global Forest Cover Change Tree Cover 30 m Global (GFCC-TC). All products included data at 10-30 m resolution and represented the state of forest or tree cover from 2010 to 2020. These seven products were chosen based on: a) feedback from end-users in Mexico; b) availability and FAIR (findable, accessible, interoperable, and replicable) data principles; and c) products representing different methodological approaches from global to regional scales. The combined agreement map documents forest cover for each satellite-derived product at 30-m resolution across Mexico. The data are in cloud optimized GeoTIFF format and cover the period 2010-2020. A shapefile is included that outlines Mexico mainland areas.
Project: Carbon Monitoring System
The NASA Carbon Monitoring System (CMS) program is designed to make significant contributions in characterizing, quantifying, understanding, and predicting the evolution of global carbon sources and sinks through improved monitoring of carbon stocks and fluxes. The System uses NASA satellite observations and modeling/analysis capabilities to establish the accuracy, quantitative uncertainties, and utility of products for supporting national and international policy, regulatory, and management activities. CMS data products are designed to inform near-term policy development and planning.
Related Publication
Braden, D., P. Mondal, T. Park, J.A. Alanís de la Rosa, M.I. Aldrete Leal, R.A. Cuenca Lara, R. Mayorga Saucedo, F. Paz, V.M. Salas-Aguilar, M. de L.Á. Soriano-Luna, and R. Vargas. 2024. Estimating forest extent across Mexico. Environmental Research Letters 19:014083. https://doi.org/10.1088/1748-9326/ad193e
Related Dataset
Park, T., and R. Vargas. 2022. Tree Cover Estimates at 30 m Resolution for Mexico, 2016-2018. ORNL DAAC, Oak Ridge, Tennessee, USA. https://doi.org/10.3334/ORNLDAAC/2137
Acknowledgement
This study was funded by NASA’s Carbon Monitoring System (CMS, grant 80NSSC21K0964).
Data Characteristics
Spatial Coverage: Mexico
Spatial Resolution: 30 m
Temporal Coverage: 2010-01-01 to 2020-12-31
Temporal Resolution: One 10-year period
Study Area: All latitude and longitude given in decimal degrees
Site (Region) | Westernmost Longitude | Easternmost Longitude | Northernmost Latitude | Southernmost Latitude |
---|---|---|---|---|
Mexico | -120.30611 | -84.295 | 34.51 | 12.4781 |
Data File Information:
There are two files in this dataset: a cloud optimized GeoTIFF format (*.tif) and a shapefile in a compressed zip archive (*.zip).
The shapefile, mex_shapefile_projected.zip, holds a single polygon that outlines the mainland regions of Mexico. The shapefile has the same coordinate system as the GeoTIFF.
The GeoTIFF is named: All_Products_Comp_Over30_Binary.tif.
GeoTIFF Information:
- Coordinate system: NAD 1983 Equidistant Conic North America (EPSG: 102010, Datum: NAD83);
Proj4: "+proj=aea +lat_1=20 +lat_2=60 +lat_0=40 +lon_0=-96 +x_0=0 +y_0=0 +datum=NAD83 +units=m +no_defs" - Spatial resolution: 30 m
- Data Type: UInt32 - 32 bit unsigned integer
- Valid Range: 0 and 10000001 to 11111111
- Nodata value: 4294967295
A pixel value of 0 indicates that the pixel was not characterized as forest in any of the remote sensing-based forest products. These "0" pixels occur over water and land areas of the map extent. The shapefile may be used as a mask to clip the mainland areas of the GeoTIFF.
Pixel values >0 indicate which of the seven satellite-based forest products identified the pixel as having forest cover. These values are 8-digit integer numbers with a leading "1" in the first position (Table 1). The following seven positions indicate forest (1) or non-forest (0) for each forest product. The leading 1 serves as a placeholder that prevents a "0" in position 2 from disappearing.
Table 1. Schema for indicating forest cover in pixel values for seven remote sensing-based forest products (Table 2) using an 8-digit number. 1 = forest, 0 = no forest.
Remote sensing-based forest product | ||||||||
---|---|---|---|---|---|---|---|---|
Leading 1 | NEX-TC | Hansen-TC | GFCC-TC | CEC | Globeland30 | IO | ESA | |
Digit position | 1 | 2 | 3 | 4 | 5 | 6 | 7 | 8 |
Number in the corresponding position of an example pixel value | 1 | 0 | 1 | 1 | 0 | 1 | 0 | 1 |
Example pixel value | 10110101 |
The example pixel value in Table 1 ("10110101") indicates that the pixel was recorded as forested in the Hansen-TC, GFCC-TC, and Globeland30 products but recorded as without forest in the NEX-TC, CEC, and IO products. Similarly, a pixel with "10000001" indicates that only the ESA product recorded forest at that pixel.
Table 2. Remote sensing-based forest products, spatial resolution, nominal year, and primary remote sensing inputs.
Product Name (Abbreviation) | Spatial Resolution | Nominal Year | Primary Remote Sensing Inputs | Citation |
---|---|---|---|---|
NAIP Trained Mean Percent Cover Map (NEX-TC) | 30 m | 2017 | Landsat 8 and NAIP | Park and Vargas (2022) |
Global Land Analysis and Discovery Global 2010 Tree Cover (Hansen-TC) | 30 m | 2010 | Landsat 7 | Hansen et al. (2013) |
Global Forest Cover Change Tree Cover 30m Global (GFCC-TC) | 30 m | 2010 | Landsat 5 and 7 | Townshend (2016) |
Commission for Environmental Cooperation 2015 Land Cover Map (CEC) | 30 m | 2015 | RapidEye | CEC (2020) |
Globeland30 2020 (Globeland30) | 30 m | 2020 | Landsat 8, HJ-1, and GF-1 | Chen et al. (2014) |
Impact Observatory 2020 Land Cover Map (IO) | 10 m | 2020 | Sentinel-2 | Karra et al. (2021) |
European Space Agency 2020 Land Cover Map for Mexico (ESA) | 10 m | 2020 | Sentinel-2A and 2B | Zanaga et al. (2022) |
Application and Derivation
This study provides a method to compare satellite-derived forest cover products. In many instances, country specific data depend heavily on remote sensing-based (RSB) forest cover maps for reporting statistics related to national and international commitments (FAO, 2012). Therefore, RSB forest cover estimates are vital information for national-to-regional measurement, reporting, and verification (MRV) systems. Different forest definitions, remote sensing-based (RSB) products, and data availability can lead to discrepancies in reporting total forest area. Consequently, errors in forest extent can be propagated into forest biomass and carbon estimates.
Quality Assessment
Plot-level data from the Mexican government’s Inventario Nacional Forestal y de Suelos (National Forest and Soil Inventory, INFyS; CONAFOR, 2014) from 2009-2014 were utilized to compare how the seven remotely sensed based (RSB) products agreed with national data. The most recent year of data on tree cover density was used at each location, and a 30% forest definition was applied to identify all “forest” plots for direct comparison to the seven products. Then, the number of locations identified as forest by both INFyS and each RSB product was divided by the total number of INFyS plots meeting the forest definition. The products had higher agreement with ground observations regarding forest status in tropical moist forest (mean agreement of 90%), tropical rainforest (mean agreement of 77%), and the tropical mountain system (mean agreement of 74%). Products had low agreement with ground observations in the subtropical steppe (mean agreement of 28%) and subtropical desert (32%).
Plot-level local data were also provided from Salas-Aguilar et al. (2017). A complementary analysis was performed to test the accuracy of each product within Edomex, which lies primarily in the subtropical mountain system. The same forest definition and methods were applied to these ground observations, and RSB products ranged in forest agreement from 75% to 94% with a mean agreement of 87%.
These assessments both provided valuable insights into regions where this forest extent agreement map, which is a combination of the seven individual products, has higher or lower agreement with ground observations in Mexico. Generally, accuracy was lower in drier, subtropical regions and higher in more forest dense regions (e.g., tropical moist forest and tropical rainforest). Within Edomex, RSB products had higher agreement with ground observation data than with INFyS data.
Data Acquisition, Materials, and Methods
Remotely sensed-based (RSB) forest extent products
Seven global, regional, and national forest and tree cover data products were selected for Mexico: ESA, Globeland30, CEC, IO, NEX-TC, Hansen-TC, and GFCC-TC (Table 2). All RSB products had 10-30 m resolution and represented the state of forest or tree cover from 2010 to 2020. Several other RSB products exist (Liu et al., 2021), but these seven were chosen based on: a) feedback from end-users in Mexico considering familiarity with the RSB products; b) availability and FAIR (i.e., findable, accessible, interoperable, and replicable) data principles; and c) products representing different methodological approaches from global to regional scales. The original seven products were developed from various RSB data sources (Table 2). Each RSB product was extracted for mainland Mexico.
ESA, CEC, IO, and Globeland30 were originally land cover products with various forest classes. For each product, all forest classes were combined into one forest class, and other pixels identified as non-forest classes (e.g., water, urban, etc.) were removed. The ESA and IO products were resampled to a 30-m spatial resolution.
NEX-TC, Hansen-TC, and GFCC-TC were originally tree cover products, reporting the percentage of tree coverage within a pixel. To determine forest/non-forest, a 30% threshold was used to determine forested pixels. In other words, pixels with ≥30% tree cover were considered ‘forest,’ and pixels with <30% cover were considered ‘non-forest.’ Non-forest pixels were removed.
A shapefile is included that outlines mainland Mexico and may be used as a mainland-ocean mask. Pixels with value of 0 outside of the mainland polygon should be considered nodata. The shapefile was modified from INEGI (2022).
Creation of Forest Extent Agreement Map
All RSB products forest pixels were given a unique binary value and were combined to create the included forest extent agreement map. This schema resulted in each pixel having an 8-digit binary code that establishes which of the RSB products classified the pixel as being forested or non-forested (Table 1).
The forest extent agreement map allows end-users to identify consensus of RSB products in Mexico. This map may be helpful for evaluating the impact of utilizing any one of the seven RSB products within a particular region of Mexico or for other analyses related to Mexican forest cover.
Refer to Braden et al. (2024) for more details.
Data Access
These data are available through the Oak Ridge National Laboratory (ORNL) Distributed Active Archive Center (DAAC).
Satellite-Derived Forest Extent Likelihood Map for Mexico
Contact for Data Center Access Information:
- E-mail: uso@daac.ornl.gov
- Telephone: +1 (865) 241-3952
References
Braden, D., P. Mondal, T. Park, J.A. Alanís de la Rosa, M.I. Aldrete Leal, R.A. Cuenca Lara, R. Mayorga Saucedo, F. Paz, V.M. Salas-Aguilar, M. de L.Á. Soriano-Luna, and R. Vargas. 2024. Estimating forest extent across Mexico. Environmental Research Letters 19:014083. https://doi.org/10.1088/1748-9326/ad193e
CEC. 2020. 2015 Land Cover of North America at 30 Meters. Commission for Environmental Cooperation (CEC): North American Land Change Monitoring System. Canada Centre for Remote Sensing (CCRS), U.S. Geological Survey (USGS), Comisión Nacional para el Conocimiento y Uso de la Biodiversidad (CONABIO), Comisión Nacional Forestal (CONAFOR), Instituto Nacional de Estadística y Geografía (INEGI). Ed. 2.0, Raster digital data [30-m]. http://www.cec.org/north-american-environmental-atlas/land-cover-30m-2015-landsat-and-rapideye/
CONAFOR. 2014. Inventario Nacional Forestal y de Suelos (INFyS 2009–2014); Comisión Nacional Forestal (CONAFOR): Zapopan, Jalisco, Mexico. https://datos.gob.mx/busca/organization/conafor
FAO. 2012. Global ecological zones for FAO forest reporting: 2010 update. Forest Resources Assessment Working Paper 179. Food and Agriculture Organization of the United Nations; Rome, Italy. https://www.fao.org/forest-resources-assessment/remote-sensing/global-ecological-zones-gez-mapping
Hansen, M.C., P.V. Potapov, R. Moore, M. Hancher, S.A. Turubanova, A. Tyukavina, D. Thau, S.V. Stehman, S.J. Goetz, T.R. Loveland, A. Kommareddy, A. Egorov, L. Chini, C.O. Justice, and J.R. G. Townshend. 2013. High-Resolution Global Maps of 21st-Century Forest Cover Change. Science 342:850–853. https://doi.org/10.1126/science.1244693
INEGI. 2022. Áreas geoestadísticas estatales, 2022, escala: 1:250000. Instituto Nacional de Estadística y Geografía. México. http://geoportal.conabio.gob.mx/metadatos/doc/html/dest22gw.html
Karra, K., C. Kontgis, Z. Statman-Weil, J.C. Mazzariello, M. Mathis, and S.P. Brumby. 2021. Global land use / land cover with Sentinel 2 and deep learning. 2021 IEEE International Geoscience and Remote Sensing Symposium IGARSS. Brussels, Belgium. https://doi.org/10.1109/IGARSS47720.2021.9553499
Liu, L., X. Zhang, Y. Gao, X. Chen, X. Shuai, and J. Mi. 2021. Finer-resolution mapping of global land cover: recent developments, donsistency analysis, and prospects. Journal of Remote Sensing 2021:5289697. https://doi.org/10.34133/2021/5289697
Park, T., and R. Vargas. 2023. Tree Cover Estimates at 30 m Resolution for Mexico, 2016-2018. ORNL Distributed Active Archive Center. https://doi.org/10.3334/ORNLDAAC/2137
Salas-Aguilar, V., C. Sánchez-Sánchez, F. Rojas-García, F. Paz-Pellat, J. Valdez-Lazalde, and C. Pinedo-Alvarez. 2017. Estimation of Vegetation Cover Using Digital Photography in a Regional Survey of Central Mexico. Forests 8:392. https://doi.org/10.3390/f8100392
Sexton, J.O., Noojipady, P., Song, X.-P., Feng, M., Song, D.-X., Kim, D.-H., Anand, A., Huang, C., Channan, S., Pimm, S.L., Townshend, J.R., 2016. Conservation policy and the measurement of forests. Nat. Clim. Change 6, 192–196. https://doi.org/10.1038/nclimate2816
Townshend, J. 2016. Global Forest Cover Change (GFCC) Tree Cover Multi-Year Global 30 m V003. NASA EOSDIS Land Processes Distributed Active Archive Center. https://doi.org/10.5067/MEaSUREs/GFCC/GFCC30TC.003
UNFCCC, 2022. Report on the technical assessment of the proposed forest reference emission level of Mexico submitted in 2020. https://unfccc.int/documents/460852
Zanaga, D., R. Van De Kerchove, D. Daems, W. De Keersmaecker, C. Brockmann, G. Kirches, J. Wevers, O. Cartus, M. Santoro, S. Fritz, M. Lesiv, M. Herold, N.-E. Tsendbazar, P. Xu, F. Ramoino, and O. Arino. 2022, October 28. ESA WorldCover 10 m 2021 v200. Zenodo. https://doi.org/10.5281/zenodo.7254221