Documentation Revision Date: 2024-05-13
Dataset Version: 1
Summary
This dataset contains a total of 3,154 files: 3,152 files in compressed LAS (*.laz) file format, one file in comma separated values (*.csv) format, and one file in compressed Keyhole Markup Language (.kmz).
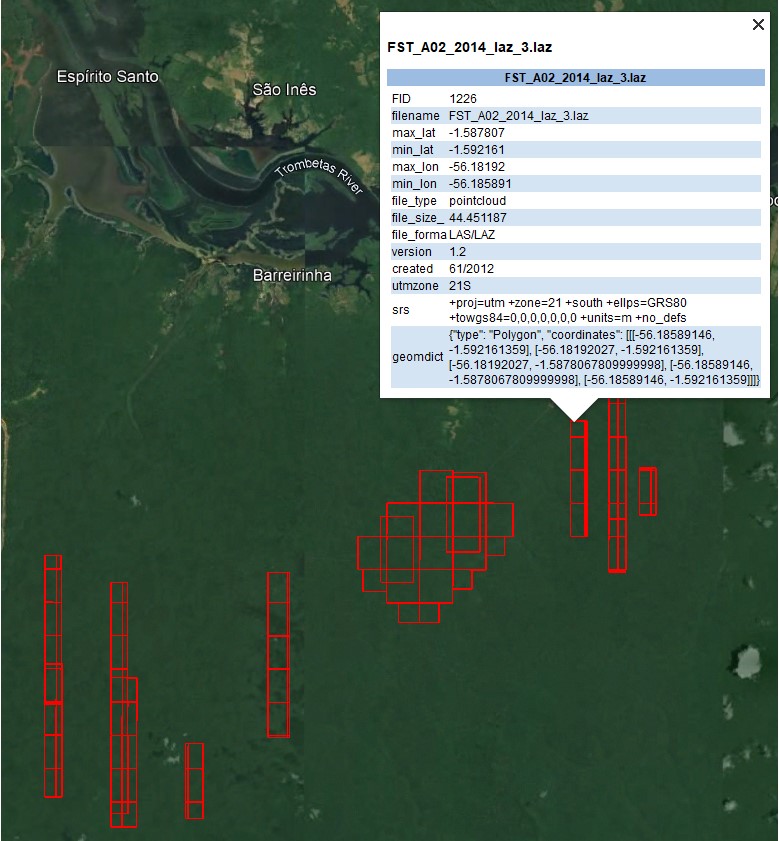
Figure 1. Bounding boxes for LiDAR tiles from surveys over western Para, Brazil are depicted in Google Earth from the KMZ file. Each feature in the KMZ provides key metadata about the corresponding tile. Source: cms_brazil_lidar_tile_inventory.kmz
Citation
dos-Santos, M.N., M.M. Keller, and D.C. Morton. 2019. LiDAR Surveys over Selected Forest Research Sites, Brazilian Amazon, 2008-2018. ORNL DAAC, Oak Ridge, Tennessee, USA. https://doi.org/10.3334/ORNLDAAC/1644
Table of Contents
- Dataset Overview
- Data Characteristics
- Application and Derivation
- Quality Assessment
- Data Acquisition, Materials, and Methods
- Data Access
- References
- Dataset Revisions
Dataset Overview
This dataset provides the complete catalog of point cloud data collected during LiDAR surveys over selected forest research sites across the Amazon rainforest in Brazil between 2008 and 2018 for the Sustainable Landscapes Brazil Project. Flight lines were selected to overfly key field research sites in the Brazilian states of Acre, Amazonas, Bahia, Goias, Mato Grosso, Para, Rondonia, Santa Catarina, and Sao Paulo. The point clouds have been georeferenced, noise-filtered, and corrected for misalignment of overlapping flight lines. They are provided in 1 km2 tiles. The data were collected to measure forest canopy structure across Amazonian landscapes to monitor the effects of selective logging on forest biomass and carbon balance, and forest recovery over time.
Project: Carbon Monitoring System (CMS)
The CMS is designed to make significant contributions in characterizing, quantifying, understanding, and predicting the evolution of global carbon sources and sinks through improved monitoring of carbon stocks and fluxes. The System will use the full range of NASA satellite observations and modeling/analysis capabilities to establish the accuracy, quantitative uncertainties, and utility of products for supporting national and international policy, regulatory, and management activities. CMS will maintain a global emphasis while providing finer scale regional information, utilizing space-based and surface-based data.
Related Publications:
Citations for the numerous related publications are given in the main reference section of this document, Section 7.
Related Datasets:
dos-Santos, M.N., and M.M. Keller. 2016. CMS: Forest Inventory and Biophysical Measurements, Para, Brazil, 2012-2014. ORNL DAAC, Oak Ridge, Tennessee, USA. https://doi.org/10.3334/ORNLDAAC/1301
dos-Santos, M.N., and M.M. Keller. 2016. CMS: LiDAR Data for Forested Areas in Paragominas, Para, Brazil, 2012-2014. ORNL DAAC, Oak Ridge, Tennessee, USA. https://doi.org/10.3334/ORNLDAAC/1302
Keller, M.M., P. Duffy, and W. Barnett. 2019. LiDAR and PALSAR-Derived Forest Aboveground Biomass, Paragominas, Para, Brazil, 2012. ORNL DAAC, Oak Ridge, Tennessee, USA. https://doi.org/10.3334/ORNLDAAC/1648
Acknowledgements:
LiDAR surveys performed through the Sustainable Landscapes project were commissioned by the United States Forest Service in collaboration with the Brazilian Enterprise for Agricultural Research (EMBRAPA) (https://www.paisagenslidar.cnptia.embrapa.br) and are archived through the Carbon Monitoring System project funded by NASA.
Data Characteristics
Spatial Coverage: Selected areas of the Amazon Basin and other regions in Brazil
Spatial Resolution: ~ 10 points per m2 provided in 1 km2 tiles over key field research sites
Temporal Coverage: June 2008 to August 2018
Temporal Resolution: single acquisition over most sites (multiple acquisition over some sites)
Study Area: (all latitude and longitudes given in decimal degrees)
Site | Westernmost Longitude | Easternmost Longitude | Northernmost Latitude | Southernmost Latitude |
---|---|---|---|---|
LiDAR flightlines over Brazil | -68.2897 | -39.058 | -1.5794 | -26.6964 |
Data File Information
This dataset contains a total of 3,154 files: 3,152 files in compressed LAS (*.laz) file format, one file in comma separated values (*.csv) format, and one file in compressed Keyhole Markup Language (.kmz).
The LAS files hold LiDAR data (Table 1).
The files cms_brazil_lidar_tile_inventory.csv and cms_brazil_lidar_tile_inventory.kmz provide summaries of the LAS file contents in tabular form (.csv) and as viewable using Google Earth (.kmz) (Table 2). Both files provide similar information about the LiDAR inventory.
LAS File characteristics
File organization and naming convention: Files are organized according to the Survey_reference_code given in Table 1 below.
For example, the reference code ANT_A01_2011_LiDAR associates the *.las data files collected at the "Floresta Estadual do Antimary" research site in 2011 with the metadata uuid shown in Table 1.
Please refer to the Sustainable Landscapes metadata for the definitions of the 3-character site codes and additional site descriptions.
File names begin with their respective Survey_reference_code and end with a sequential number identifying the specific 1 km2 tile data provided.
- ANT_A01_2011_laz_0.laz
- ANT_A01_2011_laz_1.laz
- …
- ANT_A01_2011_laz_11.laz
Note: not all file names follow this structure. Please refer to specific survey reference code Sustainable Landscapes metadata for details about these alternatively-named files.
File format: The LAS standard is developed and maintained by the American Society for Photogrammetry and Remote Sensing (ASPRS; https://www.asprs.org/divisions-committees/lidar-division/laser-las-file-format-exchange-activities) and is currently on version 1.4. These data are distributed in LAS versions 1.0 through 1.2.
Table 1. LiDAR survey information. The Survey_reference_code associates the *.las data files collected at a research site in a given year. The WebGIS of the Sustainable Landscapes Brazil project provides capabilities for finding LiDAR and related field data.
State* | Survey_reference_code | UTM zone |
---|---|---|
AC | ANT_A01_2011_LiDAR | 19S |
AC | BON_A01_2013_LiDAR | 19S |
AC | HUM_A01_2013_LiDAR | 19S |
AC | RIB_A01_2014_LiDAR | 19S |
AC | TAL_A01_2013_LiDAR | 19S |
AM | BA3_A01_2014_LiDAR | 20S |
AM | BA3_A02_2014_LiDAR | 20S |
AM | BAR_A01_2014_LiDAR | 20S |
AM | DUC_A01_2012_LiDAR | 21S |
AM | DUC_A01_2017_LiDAR | 20S |
AM | ZF2_A01_2017_LiDAR | 20S |
AM | ZF2_A02_2017_LiDAR | 20S |
BA | CON_A01_2015_LiDAR | 24S |
GO | GO1_A01_2014_LiDAR | 22S |
MT | COT_A01_2011_LiDAR | 21S |
MT | FN1_A01_2013_LiDAR | 22S |
MT | FN1_A01_2016_LiDAR | 21S |
MT | FN2_A01_2013_LiDAR | 21S |
MT | FN2_A01_2016_LiDAR | 21S |
MT | FN3_A01_2014_LiDAR | 21S |
MT | FNA_A01_2013_LiDAR | 21S |
MT | FNB_A01_2014_LiDAR | 21S |
MT | FNC_A01_2017_LiDAR | 21S |
MT | FNC_A02_2017_LiDAR | 21S |
MT | FNC_A03_2017_LiDAR | 21S |
MT | FNC_A04_2017_LiDAR | 21S |
MT | FND_A01_2017_LiDAR | 21S |
MT | FND_A02_2017_LiDAR | 21S |
MT | FND_A03_2017_LiDAR | 21S |
MT | TAN_A01_2012_LiDAR | 22S |
MT | TAN_A01_2014_LiDAR | 22S |
MT | FN3_A01_2017_LiDAR | 21S |
PA | ANA_A01_2017_LiDAR | 21S |
PA | AND_A01_2013_LiDAR | 23S |
PA | AND_A01_2017_LiDAR | 23S |
PA | CAU_A01_2017_LiDAR | 22S |
PA | FST_A01_2013_LiDAR | 21S |
PA | PAR_A01_2017_LiDAR | 23S |
PA | PRG_A01_2017_LiDAR | 23S |
PA | SAN_A01_2014_LiDAR | 21S |
PA | SAN_A02_2014_LiDAR | 21S |
PA | SFX_A01_2012_LiDAR | 22S |
PA | SFX_A02_2012_LiDAR | 22S |
PA | ST1_A01_2013_LiDAR | 21S |
PA | ST1_A01_2016_LiDAR | 21S |
PA | ST2_A01_2013_LiDAR | 21S |
PA | ST2_A01_2016_LiDAR | 21S |
PA | ST3_A01_2014_LiDAR | 21S |
PA | ST3_A01_2017_LiDAR | 21S |
PA | TAC_A01_2013_LiDAR | 22S |
PA | TAP_A01_2012_LiDAR | 21S |
PA | TAP_A01_2017_LiDAR | 21S |
PA | TAP_A02_2012_LiDAR | 21S |
PA | TAP_A02_2013_LiDAR | 21S |
PA | TAP_A02_2016_LiDAR | 21S |
PA | TAP_A03_2012_LiDAR | 21S |
PA | TAP_A03_2013_LiDAR | 21S |
PA | TAP_A03_2016_LiDAR | 21S |
RO | JAM_A01_2011_LiDAR | 20S |
RO | JAM_A02_2011_LiDAR | 20S |
RO | JAM_A02_2013_LiDAR | 20S |
RO | JAM_A03_2013_LiDAR | 20S |
SC | CAG_A01_2013_LiDAR | 22S |
SC | MMA_A01_2017_LiDAR | 22S |
SP | CAN_A01_2014_LIDAR | 23S |
SP | CAN_A01_2017_LIDAR | 23S |
SP | CAN_A02_2014_LIDAR | 23S |
SP | CAN_A02_2017_LIDAR | 23S |
SP | SDM_A01_2012_LiDAR | 23S |
SP | SDM_A01_2017_LiDAR | 23S |
* State codes in the leftmost column refer to the Brazilian states of Acre (AC), Amazonas (AM), Bahia (BA), Goias (GO), Mato Grosso (MT), Para (PA), Rondonia (RO), Santa Catarina (SC), and Sao Paulo (SP).
Spatial Reference System (Well Known Text)
Note -- these data are located across six Universal Transverse Mercator (UTM) zones: 19S, 20S, 21S, 22S, 23S, and 24S. Please refer to Table 1 for the UTM zone for a respective survey reference code and file.
The OGC standard Well Known Text representation of the UTM system used for these data:
PROJCS["WGS 84 / UTM zone __S", ### Seven zones: 19, 20, 21, 22, 23, 24 |
Table 2. Variables in cms_brazil_lidar_tile_inventory.csv and cms_brazil_lidar_tile_inventory.kmz.
Field | Description |
---|---|
filename | filename |
max_lat | maximum latitude extent (decimal degrees) |
min_lat | minimum latitude extent (decimal degrees) |
max_lon | maximum longitude extent (decimal degrees) |
min_lon | minimum longitude extent (decimal degrees) |
file_type | always "pointcloud" |
file_size_mb | size of the file in megabytes |
file_format | always "LAS/LAZ" |
version | LAS specification version (always from v1.0, v1.1, v1.2) |
created | file creation date |
utmzone | UTM zone given as "##S" (always from 19S, 20S, 21S, 22S, 23S, 24S) |
srs | proj4 string for the UTM zone |
Application and Derivation
These LiDAR acquisitions are meant to measure forest canopy structure across Amazonian landscapes to monitor the effects of selective logging on forest biomass and carbon balance and forest recovery over time.
Quality Assessment
Checks for horizontal and vertical accuracy, and other standard quality control measures were performed by affiliated LiDAR survey vendors. Please refer to http://mapas.cnpm.embrapa.br/paisagenssustentaveis/ for vendor information.
Data Acquisition, Materials, and Methods
Project Overview
Brazilian tropical forests contain approximately one-third of the global carbon stock in above-ground tropical forest biomass. Deforestation has cleared about 15% of the extensive forest on the Brazilian Amazon frontier. Logging, and understory forest fires may have degraded a similar area of forest. In response to the potential climatic effects of deforestation, policy makers have suggested reductions in emissions through deforestation and forest degradation and enhanced forest carbon stocks (REDD+). Carbon accounting for REDD+ requires knowledge of deforestation, degradation, and associated changes in forest carbon stocks.
Degradation is more difficult to detect than deforestation. This LiDAR inventory will continue to help researchers to quantify carbon stocks and changes and associated uncertainties in the Brazilian Amazon.
LiDAR Acquisition
LiDAR surveys were flown between June 2008 and August 2018. The data were collected and processed to point cloud files by commercial vendors under several grants, and incorporated into the broader integrated effort of the Sustainable Landscapes project. Sustainable Landscapes is supported by the United States Agency for International Development (USAID) and US Department of State. LiDAR surveys performed through the Sustainable Landscapes project were commissioned by the United States Forest Service in collaboration with the Brazilian Enterprise for Agricultural Research (EMBRAPA) and are archived through the Carbon Monitoring System project funded by NASA.
EMBRAPA maintains a metadata portal for the Sustainable Landscapes project at: https://www.paisagenslidar.cnptia.embrapa.br/webgis/.
Data Access
These data are available through the Oak Ridge National Laboratory (ORNL) Distributed Active Archive Center (DAAC).
LiDAR Surveys over Selected Forest Research Sites, Brazilian Amazon, 2008-2018
Contact for Data Center Access Information:
- E-mail: uso@daac.ornl.gov
- Telephone: +1 (865) 241-3952
References
Related Publications:
Andersen, Hans-Erik; McGaughey, Robert J.; Reutebuch, Stephen E. 2005. Estimating forest canopy fuel parameters using LIDAR data. Remote Sensing of Environment. 94: 441-449. https://doi.org/10.1016/j.rse.2004.10.013
Avitabile, V. , Herold, M. , Heuvelink, G. B., Lewis, S. L., Phillips, O. L., Asner, G. P., Armston, J. , Ashton, P. S., Banin, L. , Bayol, N. , Berry, N. J., Boeckx, P. , Jong, B. H., DeVries, B. , Girardin, C. A., Kearsley, E. , Lindsell, J. A., Lopez-Gonzalez, G. , Lucas, R. , Malhi, Y. , Morel, A. , Mitchard, E. T., Nagy, L. , Qie, L. , Quinones, M. J., Ryan, C. M., Ferry, S. J., Sunderland, T. , Laurin, G. V., Gatti, R. C., Valentini, R. , Verbeeck, H. , Wijaya, A. and Willcock, S. (2016), An integrated pan-tropical biomass map using multiple reference datasets. Glob Change Biol, 22: 1406-1420. https://doi.org/10.1111/gcb.13139
Chen, Q.; Lu, D.; Keller, M.; Dos-Santos, M.N.; Bolfe, E.L.; Feng, Y.; Wang, C. Modeling and Mapping Agroforestry Aboveground Biomass in the Brazilian Amazon Using Airborne Lidar Data. Remote Sens. 2016, 8, 21. https://doi.org/10.3390/rs8010021
Görgens, E. B., Soares, C. P., Nunes, M. H. and Rodriguez, L. C. (2016), Characterization of Brazilian forest types utilizing canopy height profiles derived from airborne laser scanning. Appl Veg Sci, 19: 518-527. https://doi.org/10.1111/avsc.12224
Hunter, M. O., Keller, M., Victoria, D., and Morton, D. C.: Tree height and tropical forest biomass estimation, Biogeosciences, 10, 8385–8399, 2013. https://doi.org/10.5194/bg-10-8385-2013
Hunter MO, Keller M, Morton D, Cook B, Lefsky M, Ducey M, et al. (2015) Structural Dynamics of Tropical Moist Forest Gaps. PLoS ONE 10(7): e0132144. https://doi.org/10.1371/journal.pone.0132144
Jucker, T. , Caspersen, J. , Chave, J. , Antin, C. , Barbier, N. , Bongers, F. , Dalponte, M. , Ewijk, K. Y., Forrester, D. I., Haeni, M. , Higgins, S. I., Holdaway, R. J., Iida, Y. , Lorimer, C. , Marshall, P. L., Momo, S. , Moncrieff, G. R., Ploton, P. , Poorter, L. , Rahman, K. A., Schlund, M. , Sonké, B. , Sterck, F. J., Trugman, A. T., Usoltsev, V. A., Vanderwel, M. C., Waldner, P. , Wedeux, B. M., Wirth, C. , Wöll, H. , Woods, M. , Xiang, W. , Zimmermann, N. E. and Coomes, D. A. (2017), Allometric equations for integrating remote sensing imagery into forest monitoring programmes. Glob Change Biol, 23: 177-190. https://doi.org/10.1111/gcb.13388
Leitold, V., Keller, M., Morton, D.C. et al. Airborne lidar-based estimates of tropical forest structure in complex terrain: opportunities and trade-offs for REDD+. Carbon Balance Manage 10, 3 (2015). https://doi.org/10.1186/s13021-015-0013-x
Leitold, V. , Morton, D. C., Longo, M. , dos Santos, M. N., Keller, M. and Scaranello, M. 2018. El Niño drought increased canopy turnover in Amazon forests. New Phytol, 219: 959-971. https://doi.org/10.1111/nph.15110
Longo, M., Keller, M., dos-Santos, M. N., Leitold, V., Pinagé, E. R., Baccini, A., Saatchi, S., Nogueira, E. M., Batistella, M., and Morton, D. C. ( 2016), Aboveground biomass variability across intact and degraded forests in the Brazilian Amazon, Global Biogeochem. Cycles, 30, 1639– 1660, https://doi.org/10.1002/2016GB005465
Meyer, V., Saatchi, S., Clark, D. B., Keller, M., Vincent, G., Ferraz, A., Espírito-Santo, F., d'Oliveira, M. V. N., Kaki, D., and Chave, J.: Canopy area of large trees explains aboveground biomass variations across neotropical forest landscapes, Biogeosciences, 15, 3377–3390, 2018. https://doi.org/10.5194/bg-15-3377-2018
Yhasmin Mendes de Moura, Thomas Hilker, Alexei I. Lyapustin, Lênio Soares Galvão, João Roberto dos Santos, Liana O. Anderson, Célio Helder Resende de Sousa, Egidio Arai, Seasonality and drought effects of Amazonian forests observed from multi-angle satellite data, Remote Sensing of Environment, Volume 171, 2015, Pages 278-290, ISSN 0034-4257. https://doi.org/10.1016/j.rse.2015.10.015
Danielle I Rappaport, Douglas C Morton, Marcos Longo, Michael Keller, Ralph Dubayah, and Maiza Nara dos-Santos. 2018. Quantifying long-term changes in carbon stocks and forest structure from Amazon forest degradation. Environmental Research Letters, Volume 13, Number 6, 065-113. https://doi.org/10.1088/1748-9326/aac331
Sato, L.Y.; Gomes, V.C.F.; Shimabukuro, Y.E.; Keller, M.; Arai, E.; Dos-Santos, M.N.; Brown, I.F.; Aragão, L.E.O.C. Post-Fire Changes in Forest Biomass Retrieved by Airborne LiDAR in Amazonia. Remote Sens. 2016, 8, 839. https://doi.org/10.3390/rs8100839
Silva, C.A.; Hudak, A.T.; Vierling, L.A.; Klauberg, C.; Garcia, M.; Ferraz, A.; Keller, M.; Eitel, J.; Saatchi, S. Impacts of Airborne Lidar Pulse Density on Estimating Biomass Stocks and Changes in a Selectively Logged Tropical Forest. Remote Sens. 2017, 9, 1068. https://doi.org/10.3390/rs9101068
R. Treuhaft et al., "Tropical-Forest Biomass Estimation at X-Band From the Spaceborne TanDEM-X Interferometer," in IEEE Geoscience and Remote Sensing Letters, vol. 12, no. 2, pp. 239-243, Feb. 2015. https://doi.org/10.1109/LGRS.2014.2334140
Dataset Revisions
Version | Release Date | Revision Notes |
---|---|---|
2024-XX-XX | File names updated for ten files: TAP_A0*_2008*.laz. Fourteen duplicate data files were deleted. CSV and KMZ companion files were updated and moved to data files. |
|
1.0 | 2019-12-31 | Original release |