Documentation Revision Date: 2017-07-19
Data Set Version: 1
Summary
There are five data files in GeoTIFF (.tif) format.
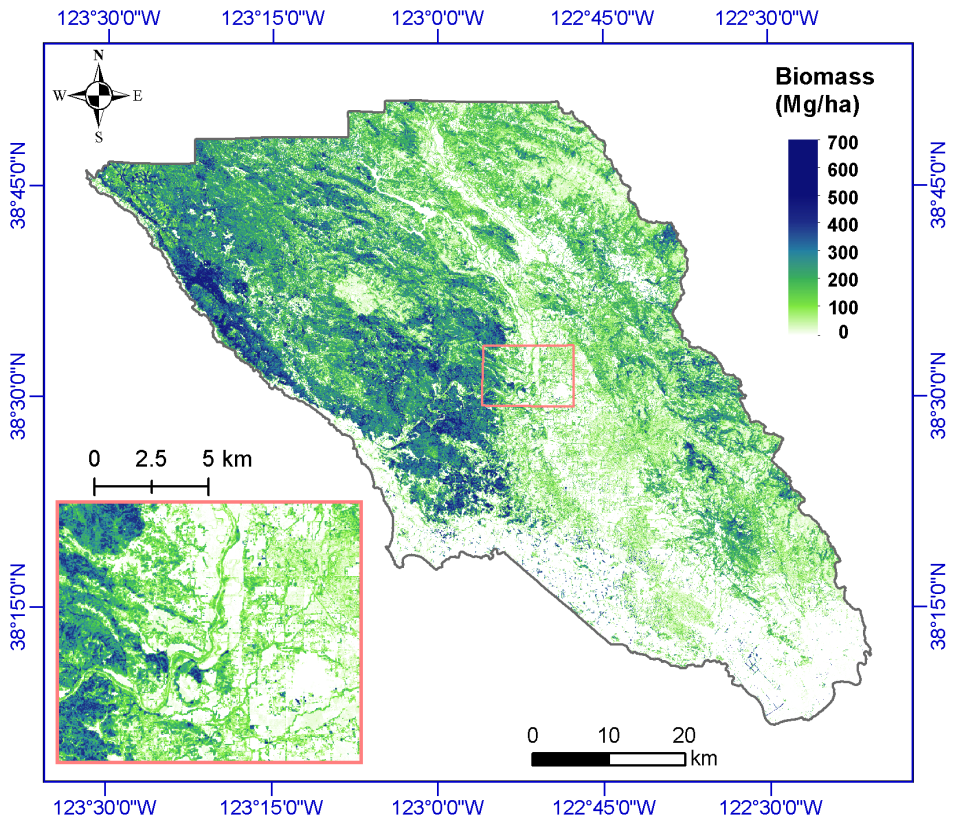
Figure 1. Estimated aboveground biomass (Mg/ha) for Sonoma County at 30-m spatial resolution using the random forest method. Zoom-in figure is a subset near Santa Rosa, the largest city in California's North Coast.
Citation
Dubayah, R.O., A. Swatantran, W. Huang, L. Duncanson, H. Tang, K. Johnson, J.O. Dunne, and G.C. Hurtt. 2017. CMS: LiDAR-derived Biomass, Canopy Height and Cover, Sonoma County, California, 2013. ORNL DAAC, Oak Ridge, Tennessee, USA. https://doi.org/10.3334/ORNLDAAC/1523
Table of Contents
- Data Set Overview
- Data Characteristics
- Application and Derivation
- Quality Assessment
- Data Acquisition, Materials, and Methods
- Data Access
- References
Data Set Overview
This data set provides estimates of above-ground biomass (AGB), canopy height, and percent tree cover at 30-m spatial resolution for Sonoma County, California, USA, for the nominal year 2013. Biomass estimates, megagrams of biomass per hectare (Mg/ha) were generated using a combination of LiDAR data, field plot measurements, and random forest modeling approaches. Estimates of AGB uncertainty are also provided. Maximum canopy height and tree cover were derived from LiDAR data and high-resolution National Agriculture Imagery Program (NAIP) images.
Project: Carbon Monitoring System
The NASA Carbon Monitoring System (CMS) is designed to make significant contributions in characterizing, quantifying, understanding, and predicting the evolution of global carbon sources and sinks through improved monitoring of carbon stocks and fluxes. The System will use the full range of NASA satellite observations and modeling/analysis capabilities to establish the accuracy, quantitative uncertainties, and utility of products for supporting national and international policy, regulatory, and management activities. CMS will maintain a global emphasis while providing finer scale regional information, utilizing space-based and surface-based data and will rapidly initiate generation and distribution of products both for user evaluation and to inform near-term policy development and planning.
Related data sets:
Cook, B., A. Swatantran, L. Duncanson, A. Armstrong, N. Pinto, R. Nelson. 2014. CMS: LiDAR-derived Estimates of Aboveground Biomass at Four Forested Sites, USA. ORNL DAAC, Oak Ridge, Tennessee, USA. https://doi.org/10.3334/ORNLDAAC/1257
Dubayah, R.O., A. Swatantran, W. Huang, L. Duncanson, K. Johnson, H. Tang, J.O. Dunne, and G.C. Hurtt. 2016. CMS: LiDAR-derived Aboveground Biomass, Canopy Height and Cover for Maryland, 2011. ORNL DAAC, Oak Ridge, Tennessee, USA. https://doi.org/10.3334/ORNLDAAC/1320
Data Characteristics
Spatial Coverage: Sonoma County, California, USA
Spatial Resolution: Grid cells at 30-meter resolution
Temporal Coverage: The data set has no explicit temporal component. Data are nominally for the year 2013.
Temporal Resolution: One time
Study Area (All latitude and longitude given in decimal degrees)
Site | Westernmost Longitude | Easternmost Longitude | Northernmost Latitude | Southernmost Latitude |
---|---|---|---|---|
Sonoma County, California | -123.542 | -122.339 | 38.86417 | 38.10167 |
Data file information
This data set provides five data files in GeoTIFF (.tif) format. Pixels with no data (out of county boundary) are represented by values of -9999.
Table 1. File names and descriptions
File names: | Description |
---|---|
sonoma_treecover_percent.tif | Percentage tree cover derived from LiDAR and NAIP imagery. Provided as decimal fraction. |
sonoma_canopyheight.tif | Maximum tree canopy height derived from Lidar and NAIP imagery provided in meters |
sonoma_biomass_predicted.tif | Aboveground biomass estimates based on a Random Forest model provided in Mg/ha |
sonoma_biomass_low.tif | Lower 10% confidence limit for each grid cell based on Quantile Random Forest model, Mg/ha |
sonoma_biomass_high.tif | Upper 90% confidence limit for each grid cell based on Quantile Random Forest model, Mg/ha |
Properties of the GeoTIFF files
Table 2. All files have the following properties:
Map units | File type | Projection/Coord System | # bands | Data type | Fill value | Westernmost Longitude | Easternmost Longitude | Northernmost Latitude | Southernmost Latitude |
---|---|---|---|---|---|---|---|---|---|
meter | raster | Transverse Mercator / GCS North American 1983 | 1 | Tree cover and canopy height data are float32. All other data are data type Int16. | -9999 | -123.542 | -122.339 | 38.86417 | 38.10167 |
Table 3. Pixel value range in each file
File name | Minimum value | Maximum value |
---|---|---|
sonoma_treecover_percent.tif | 0 | 1 |
sonoma_canopyheight.tif | 0 | 151.2 |
sonoma_biomass_predicted.tif | 0 | 527 |
sonoma_biomass_low.tif | 0 | 367 |
sonoma_biomass_high.tif | 0 | 625 |
Application and Derivation
These data represent initial attempts to estimate local to regional scale of vegetation carbon stocks using existing in situ, airborne, and satellite observations. One goal of this CMS project was to demonstrate how well biomass can be quantified with high-quality remotely-sensed data taken at fine spatial resolution for selected sites representative of US forest types and conditions (Huang et al., 2017).
Quality Assessment
The authors captured the uncertainty in the modeled biomass estimates for the Sonoma site using the Quantile Random Forest Model (QRF). Spatially-gridded estimates of the upper and lower bounds of the 90% confidence interval of the mean, at 30-m spatial resolution, are provided for the Sonoma QRF models as part of the data set.
Uncertainty in the Sonoma biomass estimates was derived from multiple factors, including, but not limited to: field sampling errors and uneven spatial distribution, uncertainty in remote-sensing data products, choice of grid cell size, and model error. The RF didn’t extrapolate beyond the range of the training data. Therefore they underestimate high and overestimate low biomass (Huang et al., 2017).
Figure 2. Estimated aboveground biomass (Mg/ha) and uncertainty percentiles for the Sonoma County at 30-m spatial resolution. For any 30-m pixel, the lower 10th percentile and upper 90th percentiles of confidence interval generated by Quantile Random Forests are shown (Huang et al., 2017).
Data Acquisition, Materials, and Methods
Above-ground biomass, canopy height data (m), and percent tree cover were determined and mapped for the nominal year 2013 for Sonoma County, CA.
Aboveground Biomass (AGB)
AGB maps were generated using a combination of field data (forest inventory and LiDAR) and Random Forest modeling approaches. The principle of linking LiDAR derived metrics with field estimated AGB has been demonstrated in a previous pilot CMS project in two counties of Maryland (Dubayah, 2012) and the whole State of Maryland (Huang et al., 2015; Dubayah et al., 2016).
The Random Forest model was first trained and calibrated using LiDAR and ancillary metrics, and field estimates of biomass from 179 variable radius plots. The RF model was then applied to the countywide LiDAR and ancillary metrics at 30-m resolution. Model validation was performed through local comparisons with US Forest Inventory and Analysis National Program (FIA) data (https://www.fia.fs.fed.us/tools-data/).
Field Plots
The field sample plots were located and selected through stratified sampling of land cover strata defined by the Classification Assessment with LANDSAT of Visible Ecological Groupings (CALVEG) land cover product (evergreen, deciduous, shrub, mixed and non-forest) and LiDAR canopy heights (low: 0 - 5 m, medium: 5 - 25 m and high: > 25 m). Tree measurements of diameter at breast height were recorded in each plot. Allometric estimates of AGB (Mg ha-1) were calculated for each tree using equations from FIA’s Component Ratio Method (Heath et al, 2008; Woodall et al., 2011) and appropriate blow up factors were applied to estimate biomass density for the variable radius plots. Model validation was performed through local comparisons with FIA data. Field sample data will be made available in forthcoming data sets (Huang et al., 2017).
LiDAR Data
LiDAR data (~8 points/ sq.m.) were acquired over Sonoma County by Watershed Sciences Inc (WSI) in September – November of 2013 covering ~440,000 ha (44 flights). Airborne discrete return LiDAR instrument - Leica ALS70 sensor was mounted on a Cessna Grand Caravan. The LiDAR data were processed and classified to generate bare earth DEMs and Canopy Height Models for aboveground biomass estimation.
Tree Canopy Height and Cover
The tree canopy cover map was created using an object-based, data-fusion approach (LiDAR and high-resolution National Agriculture Imagery Program (NAIP) images), and then aggregated to 30-m by averaging.
The canopy height map was generated using LiDAR-derived normalized digital surface model (ndsm) and tree cover map, and then aggregated to 30-m by maximum (Huang et al., 2017).
Figure 3. Max canopy height (in meters) for Sonoma County of California at 30-m spatial resolution. Insert figure is a subset near Santa Rosa, the largest city in California's North Coast (Huang et al., 2017).
Figure 4. Percent tree cover (%) for the Sonoma at 30-m spatial resolution. Insert figure is a subset near Santa Rosa, the largest city in California’s North Coast (Huang et al., 2017).
Data Access
These data are available through the Oak Ridge National Laboratory (ORNL) Distributed Active Archive Center (DAAC).
CMS: LiDAR-derived Biomass, Canopy Height and Cover, Sonoma County, California, 2013
Contact for Data Center Access Information:
- E-mail: uso@daac.ornl.gov
- Telephone: +1 (865) 241-3952
References
CALVEG 2000 (existingvegnorcoastmid_1998_2007_v1 & existingvegnorcoastwest_2000_2007_v1). USDA Forest Service - Pacific Southwest Region - Remote Sensing Lab, Ed. McClellan, CA, 2014.
Dubayah, R.O., A. Swatantran, W. Huang, L. Duncanson, K. Johnson, H. Tang, J.O. Dunne, and G.C. Hurtt. 2016. CMS: LiDAR-derived Aboveground Biomass, Canopy Height and Cover for Maryland, 2011. ORNL DAAC, Oak Ridge, Tennessee, USA. https://doi.org/10.3334/ORNLDAAC/1320
Dubayah, R., County-scale Carbon Estimation in NASA's Carbon Monitoring System (2012). Biomass and Carbon Storage, available online: https://nacp-files.nacarbon.org/biomass_pilot/presentations/County%20Biomass%20Report.pdf
Duncanson, L., Huang, W., Johnson, K., Swatantran, A., McRoberts, R., and Dubayah, R. 2017. Implications of Allometric Equation Selection for County-Level Biomass Estimates. Carbon Balance and Management (under review).
Heath, L.S.; Hansen, M.; Smith, J.E.; Miles, P.D.; Smith, B.W. (2008). Investigation into Calculating Tree Biomass and Carbon in the FIADB using a Biomass Expansion Factor Approach. McWilliams, WH, Moisen, GG & Czaplewski, RL (eds.).
Huang, W., Duncanson, L.I., Swatantran, A., Johnson, K., O-Neil Dune, J., Hurtt, G., & Dubayah, R. (2017). County-Scale Biomass Map Comparison: a Case Study for Sonoma, California in NASA's Carbon Monitoring System. Carbon Management (in revision).
Huang, W., Swatantran, A., Johnson, K., Duncanson, L., Tang, H., O'Neil Dunne, J., Hurtt, G., & Dubayah, R. (2015). Local Discrepancies in Continental Scale Biomass Maps: A Case Study over Forested and Non-Forested Landscapes in Maryland, USA. Carbon Balance and Management, 10. https://doi.org/10.1186/s13021-015-0030-9
Woodall, C.; Heath, L.S.; Domke, G.M.; Nichols, M.C., Methods and Equations for Estimating Aboveground Volume, Biomass, and Carbon for Trees in the US Forest Inventory (2010). US Department of Agriculture, Forest Service, Northern Research Station.