Documentation Revision Date: 2025-03-26
Dataset Version: 1
Summary
This dataset holds 12 tar.gz files and two files in text format.
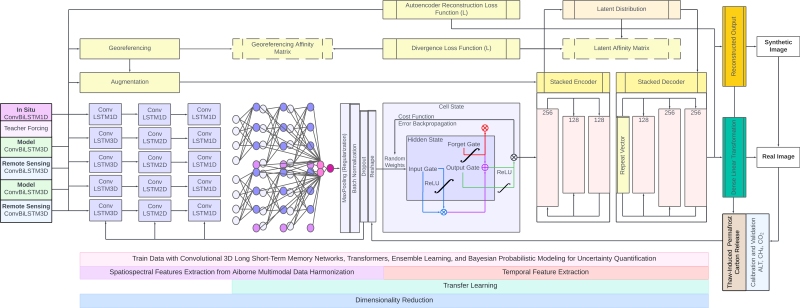
Figure 1. Elements of the model architecture and assembly, which includes model compilation, hyperparameter tuning, optimization, fitting, inverse transformation, evaluation, prediction, and error quantification. Source: documentation/architecture.pdf
Citation
Gay, B.A., N.J. Pastick, J.D. Watts, A.H. Armstrong, K. Miner, and C.E. Miller. 2025. GeoCryoAI: Ensemble Learning and the Permafrost Carbon Feedback in Alaska, 1963-2022. ORNL DAAC, Oak Ridge, Tennessee, USA. https://doi.org/10.3334/ORNLDAAC/2371
Table of Contents
- Dataset Overview
- Data Characteristics
- Application and Derivation
- Quality Assessment
- Data Acquisition, Materials, and Methods
- Data Access
- References
Dataset Overview
This dataset provides model code, input data, sample results, and documentation for an artificial intelligence-driven model, GeoCryoAI. GeoCryoAI is a hybridized process-constrained ensemble learning framework consisting of stacked convolutionally layered long short-term memory-encoded recurrent neural networks (Figure 1). The purpose of GeoCryoAI is to quantify permafrost thaw dynamics and greenhouse gas emissions in Alaska. The dataset includes pre-processed input data (i.e., thaw depth, active layer thickness (ALT), thaw subsidence; CO2 flux, CH4 flux) acquired from in situ measurements (e.g., CALM, GTNP, ITEX, SMALT STDM, ReSALT, AmeriFlux, NEON), remote sensing platforms (e.g., Uninhabited Aerial Vehicle Synthetic Aperture Radar (UAVSAR), Airborne Visible InfraRed Imaging Spectrometer - Next Generation (AVIRIS-NG)), and process-based modeling products. Field data were included to quantify CO2 and CH4 flux (e.g., chamber, eddy-covariance, and tall-tower measurements via flux tower networks) and active layer thickness (e.g., mechanical probing, borehole temperatures, ground-penetrating radar). These measurements were resampled to a 1-km grid, standardized, transformed, and assimilated into GeoCryoAI, a framework that simultaneously ingests, scales, and analyzes input data after resolving disparate spatiotemporal sampling and data densities. Model outputs were generated from two process-based models: SIBBORK Taiga-Tundra Ecotone (SIBBORK-TTE) derived thaw subsidence (Bonan, 1990; Brazhnik and Shugart, 2016) and Arctic Terrestrial Carbon Flux Model (TCFM-Arctic) generated carbon flux outputs (Watts et al., 2023). The objective was to quantify how the Arctic is changing in response to climate change and how evidence of the permafrost carbon feedback may contribute toward a better understanding of the uncertainty of nonlinear feedbacks and their impact on the earth system.
Project: Arctic-Boreal Vulnerability Experiment
The Arctic-Boreal Vulnerability Experiment (ABoVE) is a NASA Terrestrial Ecology Program field campaign based in Alaska and western Canada between 2016 and 2021. Research for ABoVE links field-based, process-level studies with geospatial data products derived from airborne and satellite sensors, providing a foundation for improving the analysis and modeling capabilities needed to understand and predict ecosystem responses and societal implications.
Related Publications:
Gay, B.A., N.J. Pastick, J.D. Watts, A. H. Armstrong, K.R. Miner, and C.E. Miller. 2025. Decoding the spatiotemporal complexities of the permafrost carbon feedback with multimodal ensemble learning, Journal of Geophysical Research, Machine Learning and Computation 2:e2024JH000402. https://doi.org/10.1029/2024JH000402
Gay, B.A., N.J. Pastick, A. E. Züfle, A.H. Armstrong, K.R. Miner, and J.J. Qu. 2023. Investigating permafrost carbon dynamics in Alaska with artificial intelligence. Environmental Research Letters 18:125001. https://doi.org/10.1088/1748-9326/ad0607
Gay, B.A., N.J. Pastick, J.D. Watts, A. H. Armstrong, K.R. Miner, and C.E. Miller. 2023. geocryoai. (Version 2.0.0). [Computer Software]. GitHub repository. https://github.com/bradleygay/geocryoai/tree/v2.0.0
Related Datasets:
Acknowledgements:
This study was funded by NASA's Postdoctoral Program at the Jet Propulsion Laboratory, California Institute of Technology, administered under NASA grant 80NM0018D0004.
Data Characteristics
Spatial Coverage: Alaska
Spatial Resolution: 1 km
Temporal Coverage: 1969-01-01 to 2022-12-31
Temporal Resolution: Monthly
Study Area (All latitude and longitude are given in decimal degrees.)
Site | Northern Extent | Western Extent | Eastern Extent | Southern Extent |
---|---|---|---|---|
Alaska | 72.0 | -170.0 | -134.77 | 54.0 |
Data File Information
This dataset holds 12 tar.gz files and two files in text format.
The text file, geocryoai_documentation.txt, provides details about files in this dataset. There are minor discrepancies between these details and the files included in the tar.gz archives described below.
Most data files are organized into four folders, and the content is provided in compressed tar.gz archives. Larger folders were split into separate archives:
- documentation - detailed description of model, including architecture and work flow
- documentation.tar.gz .
- sources - input data used by GeoCryoAI organized by source and type. These data were retrieved and compiled by the authors of this dataset from different sources, including the datasets listed in the Related Datasets table.
- sources_avirisng.tar.gz: CO2- and CH4-related absorption and flux products from AVIRIS-NG spectroscopy.
- sources_era5.tar.gz: two netCDFs, blh_interpolated_data.nc (boundary level height) and ws_interpolated_data.nc (wind speed)
- sources_insitu.tar.gz: in situ measurements of carbon flux and active layer thickness
- sources_sibborktte.tar.gz: thaw subsidence derived from SIBBORK-TTE model
- sources_tcfmarctic.tar.gz: carbon flux estimates from the TCFM-Arctic model
- sources_uavsar.tar.gz: thaw subsidence measured by vertical displacement by UAVSAR
-
- preprocessing_data.tar.gz - input data files, processed and derived from the input data sources listed above, used in the AI model
- preprocessing_code.tar.gz - scripts for preparing input data files for the AI model
- modeling - the source codebase and architecture of the GeoCryoAI framework, input and output files, and postprocessing deliverables.
- modeling_code.tar.gz - holds the code, development, and evaluation subfolders. The code subfolder includes a Jupyter notebook to demonstrate model execution.
- modeling_data.tar.gz - holds the data subfolder that contains sample input data files.
- modeling_results.tar.gz - holds the results subfolder that contains sample output data files.
The text file, geocryoai_file_list.txt, lists the names of files in tar.gz archives organized by the order listed above.
The GeoCryoAI model code generates input files and intermediate products holding spatial data using the NSIDC EASE-Grid 2.0 Global projection (EPSG: 6933). Input data with various spatial resolutions are resampled to a 1-km grid during pre-processing.
Application and Derivation
The objective was to quantify how the Arctic is changing in response to climate change and how evidence of the permafrost carbon feedback may contribute toward a better understanding of the uncertainty of nonlinear feedbacks and their impact on the earth system.
Quality Assessment
The quantification of precision for permafrost degradation and carbon release were captured with each iteration of the pre-processing workflow prior to resampling, which required aggregation or interpolation, thus introducing generalization and further uncertainty. The uncertainty benchmarks were evaluated prior to and concluding resampling methods using the spatial average and standard deviation. All uncertainties were computed with the uncertain panda Python library. Collectively, ALT, CH4 flux, and CO2 flux and their derived monthly uncertainties were computed as -0.4238 ± 0.134 m, 0.499 ± 3.659 nmol C(CH4) km-2 month-1, and -5.272 ± 127.717 µmol C(CO2) km-2 month-1, respectively.
Data Acquisition, Materials, and Methods
GeoCryoAI leverages a multimodal composite of in situ measurements (Gay et al., 2023), airborne remote sensing observations, and process-based modeling (PBM) outputs to ensure biogeophysical processes are emulated by physical laws while improving the performance and predictive accuracy of the model. Moreover, this framework integrates optimization equations, regularization cost functions, and a Bayesian Optimization search algorithm to populate a hyperparameter dictionary, reconcile data mismatches, expedite convergence, and minimize error residuals during teacher forcing.
The spatial coverage and study domain consists of the State of Alaska (1.723 million km2), covering 26.92% of the Arctic-Boreal Vulnerability Experiment (ABoVE) Domain (6.4 million km2) and 11.88% of the Arctic landscape (14.5 million km2). Sites were identified as suitable for investigation and were selected in alignment with in situ and flux tower collections, UAVSAR and AVIRIS-NG flight operations, and SIBBORK-TTE and TCFM-Arctic model simulations. Mechanical probing, borehole temperatures, and ground penetrating radar were used to quantify active layer thickness during the field season. In addition, CH4 and CO2 flux measurements were derived from the AmeriFlux and National Ecological Observatory Network (NEON) flux tower networks. Additional GeoCryoAI inputs include permafrost thaw displacement and surface subsidence from UAVSAR-based SAR backscattering with subsequent interferometric SAR (inSAR) phase unwrapping and vertical displacement as well as AVIRIS-NG-based CH4 and CO2 imaging spectroscopy.
The preprocessing code includes methods for resampling, scaling, and transforming data for assimiliation into the GeoCryoAI framework. All products and outputs were upscaled and down-sampled to 1 km for ease of nesting and spatial alignment. Likewise, rescaling was used to reduce temporal heterogeneity and align measurements in time; this approach ensured that sampling frequency remained in alignment throughout the modalities. These datasets are concatenated, scaled, and flattened to specific modalities. Concluding pre-processing, resampling, scaling, and transforming, the multimodal dataset was assimilated into the GeoCryoAI framework. Model outputs were generated from two process-based models: SIBBORK-TTE derived thaw subsidence (Bonan, 1990; Brazhnik and Shugart, 2016) and TCFM-Arctic generated carbon flux outputs (Watts et al., 2023).
For a demonstration of model execution, see the JGRMLC_GeoCryoAI_Modeling_Codebase.ipynp Jupyter notebook found in modeling_code.tar.gz in the code/notebook folder.
Table 1. The pre-processing, GeoCryoAI architecture, and post-processing elements of the workflow.
Workflow Steps | Objectives and Actions |
---|---|
Step 1: Multimodal Data Integration | Objective: Collect and integrate data for permafrost carbon feedback (PCF) modeling. Gather field measurements (ALT, CH4, CO2), integrate high-resolution remote sensing data (UAVSAR, AVIRIS-NG), incorporate outputs from process-based models (SIBBORK-TTE, TCFM-Arctic), and reconcile data into a unified framework. |
Step 2: Data Preprocessing | Objective: Prepare data for GeoCryoAI framework. Apply dimensionality reduction to handle high-dimensional data, resample and scale data to a common grid resolution (i.e., 1 km), and address spatial and temporal disparities using specialized scaling laws and diagnostic statistical testing. |
Step 3: GeoCryoAI Development | Objective: Simulate the PCF using GeoCryoAI. Employ a hybridized ensemble learning framework combining convolutional layers with a Long Short-Term Memory Recurrent Neural Network (LSTM-RNN), integrate process-constrained learning to ensure physical accuracy, and use optimization techniques like Bayesian Optimization and regularization methods to fine-tune the model. |
Step 4: GeoCryoAI Training | Objective: Model training and validation. Use teacher forcing to accelerate learning, incorporate time-lagged inputs and outputs to capture temporal dynamics, and validate model performance using Root Mean Squared Error (RMSE) and inverse transformations. |
Step 5: GeoCryoAI Forecasting | Objective: Predict future states of the PCF with GeoCryoAI. Generate spatiotemporal forecasts for ALT, CH4, and CO2 fluxes, and capture both gradual and abrupt changes in the permafrost carbon system. |
Step 6: Data Post-processing | Objective: Analyze and interpret model predictions. Perform error analysis to quantify uncertainty, leverage ecological memory for long-term predictions, and visualize results for stakeholder communication. |
ALT, thawing soil, frozen ground, active layer depth, thaw depth, thaw subsidence, and height displacement are often used interchangeably throughout this data product and related publication; these terms serve as proxies to determine the amount of permafrost degradation is occurring in space and time. Additionally, Net Ecosystem Exchange (NEE) was used as a proxy for carbon dioxide flux derived from TCFM-Arctic and eddy covariance flux towers (AmeriFlux), because it represents the difference between total carbon sequestration from photosynthesis (i.e., Gross Primary Productivity, GPP) and the total carbon release through evapotranspiration (i.e., Ecosystem Respiration, ER). Consequently, carbon dioxide flux, CO2 flux, and NEE are used interchangeably.
Additional details of model development are included in the geocryoai_documentation.txt file and in Gay et al. (2025).
Data Access
These data are available through the Oak Ridge National Laboratory (ORNL) Distributed Active Archive Center (DAAC).
GeoCryoAI: Ensemble Learning and the Permafrost Carbon Feedback in Alaska, 1963-2022
Contact for Data Center Access Information:
- E-mail: uso@daac.ornl.gov
- Telephone: +1 (865) 241-3952
References
Bakian-Dogaheh, K., R.H. Chen, M. Moghaddam, Y. Yi, and A. Tabatabaeenejad. 2020. ABoVE: Active Layer Soil Characterization of Permafrost Sites, Northern Alaska, 2018. ORNL Distributed Active Archive Center. https://doi.org/10.3334/ORNLDAAC/1759
Bonan, G.B. 1990. Carbon and nitrogen cycling in North American boreal forests. Biogeochemistry 10:1-28. https://doi.org/10.1007/bf00000889
Brazhnik, K., and H.H. Shugart. 2016. SIBBORK: A new spatially-explicit gap model for boreal forest. Ecological Modelling 320:182–196. https://doi.org/10.1016/j.ecolmodel.2015.09.016
Chen, R.H., R.J. Michaelides, J. Chen, A.C. Chen, L.K. Clayton, K. Bakian-Dogaheh, L. Huang, E. Jafarov, L. Liu, M. Moghaddam, A.D. Parsekian, T.D. Sullivan, A. Tabatabaeenejad, E. Wig, H.A. Zebker, and Y. Zhao. 2022. ABoVE: Active Layer Thickness from Airborne L- and P- band SAR, Alaska, 2017, Ver. 3. ORNL Distributed Active Archive Center. https://doi.org/10.3334/ORNLDAAC/2004
Chen, A., A. Parsekian, K. Schaefer, E. Jafarov, S.K. Panda, L. Liu, T. Zhang, and H.A. Zebker. 2015. Pre-ABoVE: Ground-penetrating Radar Measurements of ALT on the Alaska North Slope. ORNL Distributed Active Archive Center. https://doi.org/10.3334/ORNLDAAC/1265
Chen, D., X. Zhu, M. Kogure, E.E. Hoy, X. Xu, N.H. F. French, L.T. Berner, A.L. Breen, S. Bret-Harte, S.J. Davidson, J.J. Ebersole, G.V. Frost, S.J. Goetz, R.E. Hewitt, J.K. Y. Hung, C.M. Iversen, G. Iwahana, R. Jandt, L.K. Jenkins, A.N. Kade, I. Klupar, T.V. Loboda, S. Ludwig, M.J. Macander, M.C. Mack, C.R. Meyers, R.J. Michaelides, E.A. Miller, S. Natali, T.W. Nawrocki, P.R. Nelson, A.D. Parsekian, E. Rastetter, M.K. Raynolds, A.V. Rocha, K. Schaefer, U. Schickhoff, E.A. G. Schuur, S. Tsuyuzaki, C.E. Tweedie, S.V. Zesati, D.A. Walker, P.J. Webber, M. Williams, and D. Zona. 2023. Field Data on Soils, Vegetation, and Fire History for Alaska Tundra Sites, 1972-2020. ORNL Distributed Active Archive Center. https://doi.org/10.3334/ORNLDAAC/2177
Douglas, T.A. 2019. ABoVE: Soil Active Layer Thaw Depths at CRREL sites near Fairbanks, Alaska, 2014-2018. ORNL Distributed Active Archive Center. https://doi.org/10.3334/ORNLDAAC/1701
Farina, M.K., and J.D. Watts. 2024. Gridded CO2 and CH4 Flux Estimates for pan-Arctic and Boreal Regions, 2003-2015. ORNL Distributed Active Archive Center. https://doi.org/10.3334/ORNLDAAC/2121
Gay, B.A., N.J. Pastick, J.D. Watts, A. H. Armstrong, K.R. Miner, and C.E. Miller. 2023. geocryoai. (Version 2.0.0). [Computer Software]. GitHub repository. https://github.com/bradleygay/geocryoai/tree/v2.0.0
Gay, B.A., N.J. Pastick, J.D. Watts, A. H. Armstrong, K.R. Miner, and C.E. Miller. 2025. Decoding the spatiotemporal complexities of the permafrost carbon feedback with multimodal ensemble learning, Journal of Geophysical Research, Machine Learning and Computation 2:e2024JH000402. https://doi.org/10.1029/2024JH000402
Gay, B.A., N.J. Pastick, A. E. Züfle, A.H. Armstrong, K.R. Miner, and J.J. Qu. 2023. Investigating permafrost carbon dynamics in Alaska with artificial intelligence. Environmental Research Letters 18:125001. https://doi.org/10.1088/1748-9326/ad0607
Liu, L., K. Schaefer, A. Chen, A. Gusmeroli, E. Jafarov, T. Schaefer, A. Parsekian, S. Panda, T. Zhang, and H.A. Zebker. 2015. Pre-ABoVE: Remotely Sensed Active Layer Thickness, Barrow, Alaska, 2006-2011. ORNL Distributed Active Archive Center. https://doi.org/10.3334/ORNLDAAC/1266
Jafarov, E., A. Parsekian, K. Schaefer, L. Liu, A. Chen, S.K. Panda, and T. Zhang. 2016. Pre-ABoVE: Active Layer Thickness and Soil Water Content, Barrow, Alaska, 2013. ORNL Distributed Active Archive Center. https://doi.org/10.3334/ORNLDAAC/1355
Miller, C.E., R.O. Green, D.R. Thompson, A.K. Thorpe, M. Eastwood, I.B. Mccubbin, W. Olson-Duvall, M. Bernas, C.M. Sarture, S. Nolte, L.M. Rios, M.A. Hernandez, B.D. Bue, and S.R. Lundeen. 2022. ABoVE: Hyperspectral Imagery AVIRIS-NG, Alaskan and Canadian Arctic, 2017-2019 V2. ORNL Distributed Active Archive Center. https://doi.org/10.3334/ORNLDAAC/2009
Miller, C.E., R.O. Green, D.R. Thompson, A.K. Thorpe, M. Eastwood, I.B. Mccubbin, W. Olson-Duvall, M. Bernas, C.M. Sarture, S. Nolte, L.M. Rios, M.A. Hernandez, B.D. Bue, and S.R. Lundeen. 2024. ABoVE: AVIRIS-NG Imaging Spectroscopy for Alaska, Canada, and Iceland, 2017-2022, V3. ORNL Distributed Active Archive Center. https://doi.org/10.3334/ORNLDAAC/2362
Miller, C.E., P. Griffith, E.E. Hoy, N. Pinto, Y. Lou, S. Hensley, B. Chapman, J.L. Baltzer, K. Bakian-Dogaheh, W.R. Bolton, L.L. Bourgeau-Chavez, R.H. Chen, B.-H. Choe, L.K. Clayton, T.A. Douglas, N.H. F. French, J.E. Holloway, G. Hong, L. Huang, G. Iwahana, L.K. Jenkins, J.S. Kimball, T.V. Loboda, M.C. Mack, P. Marsh, R.J. Michaelides, M. Moghaddam, A.D. Parsekian, K. Schaefer, P. Siqueira, D. Singh, A. Tabatabaeenejad, M.R. Turetsky, R. Touzi, E. Wig, P. Wilson, C.J. Wilson, S.D. Wullschleger, Y. Yi, H.A. Zebker, Y. Zhang, Y. Zhao, and S.J. Goetz. 2023. Summary of the ABoVE L-band and P-band Airborne SAR Surveys, 2012-2022. ORNL Distributed Active Archive Center. https://doi.org/10.3334/ORNLDAAC/2150
Minions, C., S. Natali, S. Ludwig, and J. Watts. 2018. ABoVE: Year-round Soil CO2 Efflux in Alaskan Ecosystems. ORNL Distributed Active Archive Center. https://doi.org/10.3334/ORNLDAAC/1620
Minions, C., S. Natali, J.D. Watts, S. Ludwig, and D. Risk. 2020, February 14. ABoVE: Year-Round Soil CO2 Efflux in Alaskan Ecosystems, Version 2.1. ORNL Distributed Active Archive Center. https://doi.org/10.3334/ORNLDAAC/1762
Natali, S., S. Ludwig, C. Minions, and J.D. Watts. 2018, April 17. ABoVE: Thaw Depth at Selected Unburned and Burned Sites Across Alaska. ORNL Distributed Active Archive Center. https://doi.org/10.3334/ORNLDAAC/1579
Natali, S., J. Watts, S. Potter, B.M. Rogers, S. Ludwig, A. Selbmann, P. Sullivan, B. Abbott, K. Arndt, A.A. Bloom, G. Celis, T. Christensen, C. Christiansen, R. Commane, E. Cooper, P.M. Crill, C.I. Czimczik, S. Davydov, J. Du, J. Egan, B. Elberling, S.E. Euskirchen, T. Friborg, H. Genet, J. Goodrich, P. Grogan, M. Helbig, E. Jafarov, J. Jastrow, A. Kalhori, Y. Kim, J.S. Kimball, L. Kutzbach, M. Lara, K. Larsen, B. Lee, Z. Liu, M.M. Loranty, M. Lund, M. Lupascu, N. Madani, A. Malhotra, R. Matamala, J. Mcfarland, A. Mcguire, A. Michelsen, C. Minions, W. Oechel, D. Olefeldt, F. Parmentier, N. Pirk, B. Poulter, W. Quinton, F. Rezanezhad, D. Risk, T. Sachs, K. Schaefer, N. Schmidt, E. Schuur, P. Semenchuk, G. Shaver, O. Sonnentag, G. Starr, C. Treat, M. Waldrop, Y. Wang, J. Welker, C. Wille, X. Xu, Z. Zhang, Q. Zhuang, and D. Zona. 2019. Synthesis of Winter In Situ Soil CO2 Flux in pan-Arctic and Boreal Regions, 1989-2017. ORNL Distributed Active Archive Center. https://doi.org/10.3334/ORNLDAAC/1692
Oechel, W., and A. Kalhori. 2018. ABoVE: CO2 and CH4 Fluxes and Meteorology at Flux Tower Sites, Alaska, 2015-2017. ORNL Distributed Active Archive Center. https://doi.org/10.3334/ORNLDAAC/1562
Schaefer, K., L.K. Clayton, M. Battaglia, L.L. Bourgeau-Chavez, R.H. Chen, A.C. Chen, J. Chen, K. Bakian-Dogaheh, T.A. Douglas, S.E. Grelick, G. Iwahana, E. Jafarov, L. Liu, S. Ludwig, R.J. Michaelides, M. Moghaddam, S. Natali, S.K. Panda, A.D. Parsekian, A.V. Rocha, S.R. Schaefer, T.D. Sullivan, A. Tabatabaeenejad, K. Wang, C.J. Wilson, H.A. Zebker, T. Zhang, and Y. Zhao. 2021. ABoVE: Soil Moisture and Active Layer Thickness in Alaska and NWT, Canada, 2008-2020. ORNL Distributed Active Archive Center. https://doi.org/10.3334/ORNLDAAC/1903
Schaefer, K., R.J. Michaelides, R.H. Chen, T.D. Sullivan, A.D. Parsekian, Y. Zhao, K. Bakian-Dogaheh, A. Tabatabaeenejad, M. Moghaddam, J. Chen, A.C. Chen, L. Liu, and H.A. Zebker. 2021, January 14. ABoVE: Active Layer Thickness Derived from Airborne L- and P-band SAR, Alaska, 2017. ORNL Distributed Active Archive Center. https://doi.org/10.3334/ORNLDAAC/1796
Virkkala, A.-M., S. Natali, B.M. Rogers, J.D. Watts, K. Savage, S.J. Connon, M.E. Mauritz-tozer, E.A. G. Schuur, D.L. Peter, C. Minions, J. Nojeim, R. Commane, C.A. Emmerton, M. Goeckede, M. Helbig, D. Holl, H. Iwata, H. Kobayashi, P. Kolari, E. Lopez-blanco, M.E. Marushchak, M. Mastepanov, L. Merbold, M. Peichl, O. Sonnentag, T. Sachs, M. Ueyama, C. Voigt, M. Aurela, J. Boike, G. Celis, N. Chae, T. Christensen, S. Bret-Harte, S. Dengel, H. Dolman, C. Edgar, B. Elberling, S.E. Euskirchen, A. Grelle, J. Hatakka, E.R. Humphreys, J. Jaerveoja, A. Kotani, L. Kutzbach, T. Laurila, A. Lohila, I. Mammarella, Y. Matsuura, G. Meyer, M.B. Nilsson, S.F. Oberbauer, S.J. Park, F.J. W. Parmentier, R. Petrov, A.S. Prokushkin, S. Zyrianov, C. Schulze, V.L. St.louis, E.S. Tuittila, J.P. Tuovinen, W. Quinton, A. Varlagin, D. Zona, and V.I. Zyryanov. 2021. The ABCflux Database: Arctic-Boreal CO2 Flux and Site Environmental Data, 1989-2020. ORNL Distributed Active Archive Center. https://doi.org/10.3334/ORNLDAAC/1934
Watts, J.D., M. Farina, J.S. Kimball, L.D. Schiferl, Z. Liu, K.A. Arndt, D. Zona, A. Ballantyne, E.S. Euskirchen, F.W. Parmentier, M. Helbig, O. Sonnentag, T. Tagesson, J. Rinne, H. Ikawa, M. Ueyama, H. Kobayashi, T. Sachs, D.F. Nadeau, J. Kochendorfer, M. Jackowicz-Korczynski, A. Virkkala, M. Aurela, R. Commane, B. Byrne, L. Birch, M.S. Johnson, N. Madani, B. Rogers, J. Du, A. Endsley, K. Savage, B. Poulter, Z. Zhang, L.M. Bruhwiler, C.E. Miller, S. Goetz, and W.C. Oechel. 2023. Carbon uptake in Eurasian boreal forests dominates the high-latitude net ecosystem carbon budget. Global Change Biology 29:1870–1889. https://doi.org/10.1111/gcb.16553
Watts, J.D., S. Natali, and C. Minions. 2022. Soil Respiration Maps for the ABoVE Domain, 2016-2017. ORNL Distributed Active Archive Center. https://doi.org/10.3334/ORNLDAAC/1935
Whitley, M., G. Frost, M.T. Jorgenson, M. Macander, C.V. Maio, and S.G. Winder. 2018. ABoVE: Permafrost Measurements and Distribution Across the Y-K Delta, Alaska, 2016. ORNL Distributed Active Archive Center. https://doi.org/10.3334/ORNLDAAC/1598