Documentation Revision Date: 2023-02-16
Dataset Version: 1
Summary
This dataset contains 23 shapefiles (.shp) in Zip archives.
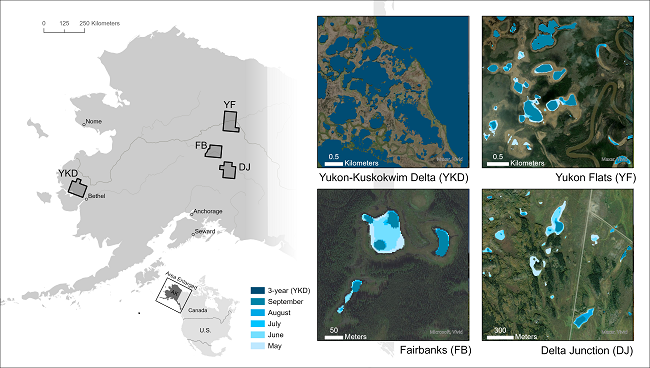
Figure 1. Four subregions of Alaska (left) with example of lakes and ponds derived from PlanetScope imagery (right). Lake and pond extents include monthly climatological composites (May - September) and 3-year composites for 2019-2021. Seasonal dynamics of expansion and contraction for these bodies of water are documented. The inset for the Fairbanks (FB) region shows a close-up of the water extents overlain on sub-meter Esri base map imagery, illustrating the 3-m resolution of the PlanetScope imagery. The Yukon Flats (YF) inset shows variability in surface area of large lakes, which is common in this region. The Delta Junction (DJ) inset shows seasonal changes in the area of small ponds. The Yukon-Kuskokwim Delta (YKD) inset shows the spatial variability of water body areas in this region and is an example of a 3-year composite.
Citation
Mullen, A., J.D. Watts, B.M. Rogers, M.L. Carroll, J.A. Caraballo-Vega, J. Noomah, Z.W. Williams, J.K.Y. Hung, and S. Natali. 2022. ABoVE: Lake and Pond Extents in Alaskan Boreal and Tundra Subregions, 2019-2021. ORNL DAAC, Oak Ridge, Tennessee, USA. https://doi.org/10.3334/ORNLDAAC/2134
Table of Contents
- Dataset Overview
- Data Characteristics
- Application and Derivation
- Quality Assessment
- Data Acquisition, Materials, and Methods
- Data Access
- References
Dataset Overview
This dataset provides polygon spatial files of lake and pond extents for three sub-regions of Interior Alaska's boreal forest, and one tundra region located in Alaska's Yukon-Kuskokwim Delta. Files provide lake and pond extents of standing water without wetland vegetation or other obstructions with a minimum area of 0.01 ha. Water extents were derived from Planet Labs PlanetScope imagery with resolution of 3.125 m. A deep learning model (U-Net) was applied to PlanetScope orthotile imagery from Planet Labs' Dove-R and Super Dove satellites. The U-Net model used the red, green, blue, and near-infrared bands along with a slope raster derived from a 30-m DEM as inputs. The U-Net detected water bodies in all available cloud-free images from the snow-free period (May-September) of 2019-2021. Water body data are provided as 3-year composites (2019-2021) for all four regions and monthly climatological composites (May-September) over 2019-2021 for the three boreal forest regions. The composite water files indicate the presence of open, standing water in >40% of valid PlanetScope observations for a given composite time-slice.
Project: Arctic-Boreal Vulnerability Experiment
The Arctic-Boreal Vulnerability Experiment (ABoVE) is a NASA Terrestrial Ecology Program field campaign based in Alaska and western Canada between 2016 and 2021. Research for ABoVE links field-based, process-level studies with geospatial data products derived from airborne and satellite sensors, providing a foundation for improving the analysis and modeling capabilities needed to understand and predict ecosystem responses and societal implications.
Related Publication
Mullen, A. J.D. Watts, B.M. Rogers, M.L. Carroll, C.D. Elder, J. Noomah, Z. Williams, A. Bredder, E. Rickenbaugh, J.A. Caraballo-Vega, E. Levenson, S.W. Cooley, S. Potter, Y. Yang, G. Fiske, C.E. Miller, S.M. Natali, J.K.Y. Hung and T.A. Douglass. 2022. Using high-resolution satellite imagery and deep learning to track dynamic seasonality in small water bodies. In review, 2022.
Acknowledgements
This project was funded by the NASA ABoVE program, grant number 80NSSC21K1161. Resources supporting this work were provided by the NASA High-End Computing (HEC) Program through the NASA Center for Climate Simulation (NCCS) at Goddard Space Flight Center. Additional support was provided by the Gordan and Betty Moore Foundation (8414).
Data Characteristics
Spatial Coverage: Alaska
Spatial Resolution: 3.125 m
Temporal Coverage: 2019-05-19 to 2021-09-28
Temporal Resolution: 3-year composites (all regions), monthly climatological composites (May-September, boreal forest regions)
Study Area: Latitude and longitude are given in decimal degrees.
Site | Westernmost Longitude | Easternmost Longitude | Northernmost Latitude | Southernmost Latitude |
---|---|---|---|---|
Alaska | -164.1265551 | -144.85616969 | 67.08180796 | 60.80058682 |
Data File Information
This dataset include 23 shapefiles (.shp) in compressed Zip archives.
Nineteen shapefiles hold composite lake and pond extents for a given subregion and time period. The file naming convention for these files is SITE_TIMEPERIOD_lakes-and-ponds.zip , where
- SITE = Abbreviation for the subregion: Delta Junction ("DJ"), Fairbanks ("FB"), Yukon Flats ("YF"), or Yukon-Kuskokwim Delta ("YKD")
- TIMEPERIOD = "3Y" indicating a three-year composite or a numeric month indicating the monthly climatological composite for May ("05"), June ("06"), July ("07"), August ("08"), or September ("09").
- Example file name: YF_05_lakes-and-ponds.zip holds the monthly climatological composite for May for Yukon Flats.
For the three boreal subregions (Delta Junction, Fairbanks, and Yukon Flats), six shapefiles (.shp) are provided. Each monthly climatological composite (May-September) has its own shapefile, and there is a shapefile for the 3-year (2019-2021) composite. For the tundra subregion, Yukon-Kuskokwim Delta, only a 3-year composite shapefile is provided.
Four additional shapefiles hold the outlines of study areas for the four subregions. The naming convention is SITE_footprint.zip.
- Example file name: YKD_footprint.zip holds an outline of the Yukon-Kuskokwim Delta study area.
Details for Shapefiles:
- Coordinate system: Canada Albers Equal Area Conic (EPSG 102001)
- Datum: NAD 1983
- Units: meters
- Proj4: "+proj= aea +lat_1= 50 +lat_2= 70 +lat_0= 40 +lon_0= -96 +x_0= 0 +y_0= 0 +datum= NAD83 +units=m +no_defs"
- Attributes: a single label for each polygon "water"
Application and Derivation
This dataset provides high-resolution polygon spatial estimates of open water in lakes and ponds and shows their seasonal variability in extent and location. Data can be used to assess spatial and intraseasonal surface water variability, inform biogeochemical models, and assist with water resource and wildlife management.
Quality Assessment
The water mapping deep learning model (U-Net) was validated with 419 PlanetScope image tiles (each 256 x 256 pixels) that contained at least one lake or pond. Open water lakes and ponds were hand-delineated in ArcGIS Pro. Precision, recall, F1, and intersection-over-union (IOU) scores were calculated based on the hand-delineated water polygons and U-Net predictions for each image tile. This test reported a precision score of 0.96, recall: 0.95, F1: 0.95, and IOU: 0.91.
As a second layer of validation, U-Net predictions were compared with 53 ground-surveyed open water body shorelines. Forty-six shorelines in the Yukon-Kuskokwim Delta region and seven shorelines were surveyed within the boreal study regions using a global positioning system (GPS) surveyor. The U-Net model was applied to PlanetScope imagery collected within one week of GPS surveys. IOU scores were calculated between the GPS-surveyed shorelines and U-Net water body predictions (Figure 2). The IOU scores indicate the smallest water body detectable was 0.01 ha. Ponds >0.01 ha and < 0.1 ha had a mean IOU of 0.4, and 54% of these ponds were at least partially detected by the U-Net. Ponds >0.1 ha had a mean IOU score of 0.81.
Figure 2. Intersection over union (IOU) scores calculated between predictions from the U-Net and ground-based GNNS shoreline surveys, based on water body area from the GNNS surveys. Each point represents one surveyed water body and points are colored by region. Forty-six shorelines were surveyed in the Yukon-Kuskokwim Delta, six were surveyed in Delta Junction, and one was surveyed in the Fairbanks subregion.
Data Acquisition, Materials, and Methods
The study sites spanned ecosystem gradients in Alaska’s interior boreal forest and the coastal Yukon-Kuskokwim Delta. The Delta Junction (DJ) study region spans from the northern foothills of the Alaska Range to the lowlands of the Tanana River, encompassing Fort Greely and the towns of Delta Junction and Big Delta. The Fairbanks (FB) study region covers the city of Fairbanks, the flat lowlands south of the Tanana River, and the Goldstream Valley and Chatanika River Valley to the north of Fairbanks. The Yukon Flats (YF) region is a low-lying fluvial plane with many lakes and ponds, situated between the Yukon-Tanana Uplands and the Brooks Range. The Yukon-Kuskokwim Delta (YKD) is a peat plateau and wetland tundra landscape located in the Yukon Delta National Wildlife Refuge in southwest Alaska with drastically varying surface water fractions across the region.
The primary data source used to map lakes and ponds was optical imagery from Planet Labs’ PlanetScope constellation (Planet Team, 2021), specifically orthotile images from the Dove-R and Super Dove PlanetScope sensors. These orthotiles had a spatial resolution of 3.125 m, were orthorectified and atmospherically corrected, and had a revisit frequency of ~1 day over these study regions. The PlanetScope imagery and a slope angle raster derived from a 30-m digital elevation model (3DEP DEM; U.S. Geological Survey, 2020) were inputs into a U-Net (Ronneberger et al., 2015), which is a class of convolutional neural network. Convolutional neural networks are a supervised classification method. To train the U-Net model, >13,000 lakes and ponds in were hand-delineated using PlanetScope imagery. References layers were used to determine the precise boundaries of water bodies during hand-delineation: 2-m Maxar WorldView-2 and Worldview-3 imagery (NextView License), ESRI base map imagery (http://goto.arcgisonline.com/maps/World_Imagery), and the 2-m ArcticDEM (Porter et al., 2018).
The trained U-Net model was used to estimate lake and pond extents in every available cloud-free PlanetScope image from the snow-free period (May-September) for 2019-2021. Occurrence ratios were calculated for each pixel by dividing the number of lake/pond observations by the total number of valid observations for a given compositing time-slice. Occurrence ratio values were then reclassified to produce water presence (value of 1 for occurrence ratio ≥0.40, otherwise value 0; Figure 2). 3-year composites used all available imagery from the study period (2019-2021). The monthly climatological composites were generated using all images from a given month (May to September) over the 3-year period.
Additional details are available in Mullen et al. (2022, In review).
Data Access
These data are available through the Oak Ridge National Laboratory (ORNL) Distributed Active Archive Center (DAAC).
ABoVE: Lake and Pond Extents in Alaskan Boreal and Tundra Subregions, 2019-2021
Contact for Data Center Access Information:
- E-mail: uso@daac.ornl.gov
- Telephone: +1 (865) 241-3952
References
Mullen, A., J.D. Watts, B.M. Rogers, M.L. Carroll, C.D. Elder, J. Noomah, Z. Williams, A. Bredder, E. Rickenbaugh, J.A. Caraballo-Vega, E. Levenson, S.W. Cooley, S. Potter, Y. Yang, G. Fiske, C.E. Miller, S.M. Natali, J.K.Y. Hung, T.A. Douglass, and E.D. Kyzivat. 2022. Using high-resolution satellite imagery and deep learning to track dynamic seasonality in small water bodies. In review, 2022.
Planet Team. 2021. Planet Imagery Product Specifications. https://assets.planet.com/docs/Planet_Combined_Imagery_Product_Specs_letter_screen.pdf
Porter, C., P. Morin, I. Howat, M.J. Noh, B. Bates, K. Peterman, S. Keesey, M. Schlenk, J. Gardiner, K. Tomko, M. Willis, C. Kelleher, M. Cloutier, E. Husby, S. Foga, H. Nakamura, M. Platson, M. Wethington, C. Williamson, G. Bauer, J. Enos, A. Galen, W. Kramer, P. Becker, A. Doshi, C. D'Souza, P. Cummens, F. Laurier, and M. Bojesen. 2018. ArcticDEM, Version 3 [Data set]. Harvard Dataverse. https://doi.org/10.7910/DVN/OHHUKH
Ronneberger, O., P. Fischer, and T. Brox. 2015. U-Net: convolutional networks for biomedical image segmentation. In N. Navab, J. Hornegger, W.M. Wells, and A.F. Frangi (eds), Medical Image Computing and Computer-Assisted Intervention – MICCAI 2015. Lecture Notes in Computer Science 9351:234–241. Springer International Publishing. https://doi.org/10.1007/978-3-319-24574-4_28
U.S. Geological Survey. 2020. 1 Arc-second Digital Elevation Models (DEMs)—USGS National Map 3DEP Downloadable Data Collection. U.S. Geological Survey. https://nationalmap.gov/3DEP/